基于Python|“数据分析岗位”招聘情况分析!
统计与数据分析实战
共 7192字,需浏览 15分钟
·
2020-09-12 15:27

数据处理
数据分析
分析报告
import pandas as pd
import warnings
import numpy as np
warnings.simplefilter(action='ignore', category=FutureWarning)
warnings.simplefilter(action='ignore', category=UserWarning)
import matplotlib as mlt
import matplotlib.pyplot as plt
%matplotlib inline
# 读取txt格式的数据
dataset = pd.read_table(r'C:/Users/Administrator/Desktop/recruits.txt',low_memory = False)
dataset.info()# 全览数据可以发现:数据缺少字段名
RangeIndex: 1193846 entries, 0 to 1193845
Data columns (total 13 columns):
940864 1193846 non-null int64
UI 1193846 non-null object
用户界面(UI)设计 1193846 non-null object
8001-10000 1193846 non-null object
3-5 1193846 non-null object
本科 1193846 non-null object
全职 1193846 non-null object
2017-11-15 1193846 non-null object
1 1193846 non-null object
杭州****技术有限公司 1193846 non-null object
计算机软件 1193690 non-null object
20-99 1193669 non-null object
杭州 1193801 non-null object
dtypes: int64(1), object(12)
memory usage: 118.4+ MB
# 自定义字段名
data = pd.read_table(r'C:/Users/Administrator/Desktop/recruits.txt',header = None,index_col = '序号',low_memory = False,
names =['序号','岗位职责','岗位名称','薪资','工作时长','学历','职业类型','发布时间','招聘人数','公司名称','所处行业','公司规模','工作地点'])
data.head()
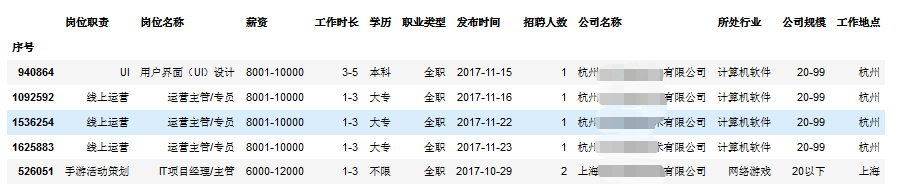
# 自定义字段名添加成功
data.info()
# result
Int64Index: 1193847 entries, 940864 to 926760
Data columns (total 12 columns):
岗位职责 1193847 non-null object
岗位名称 1193847 non-null object
薪资 1193847 non-null object
工作时长 1193847 non-null object
学历 1193847 non-null object
职业类型 1193847 non-null object
发布时间 1193847 non-null object
招聘人数 1193847 non-null object
公司名称 1193847 non-null object
所处行业 1193691 non-null object
公司规模 1193670 non-null object
工作地点 1193802 non-null object
dtypes: object(12)
memory usage: 118.4+ MB
None
# 重置索引
data = data.reset_index()
data.tail()
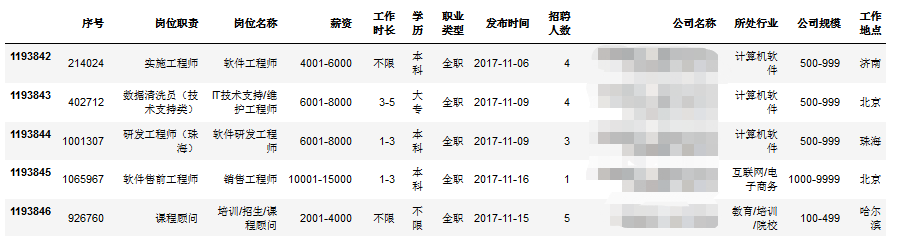
# 获取数据集的列名
data.columns
# result
Index(['岗位职责', '岗位名称', '薪资', '工作时长', '学历', '职业类型', '发布时间', '招聘人数', '所处行业', '公司规模', '工作地点'],
dtype='object')
# 转换数据集,去掉序号,去掉公司名称(脱敏)
data = data[['岗位职责', '岗位名称', '薪资', '工作时长', '学历', '职业类型', '发布时间', '招聘人数','所处行业', '公司规模', '工作地点']]
data.info()
# result
data = data[['岗位职责', '岗位名称', '薪资', '工作时长', '学历', '职业类型', '发布时间', '招聘人数','所处行业', '公司规模', '工作地点']]
data.info()
RangeIndex: 1193847 entries, 0 to 1193846
Data columns (total 11 columns):
岗位职责 1193847 non-null object
岗位名称 1193847 non-null object
薪资 1193847 non-null object
工作时长 1193847 non-null object
学历 1193847 non-null object
职业类型 1193847 non-null object
发布时间 1193847 non-null object
招聘人数 1193847 non-null object
所处行业 1193691 non-null object
公司规模 1193670 non-null object
工作地点 1193802 non-null object
dtypes: object(11)
memory usage: 100.2+ MB
data.head(10)
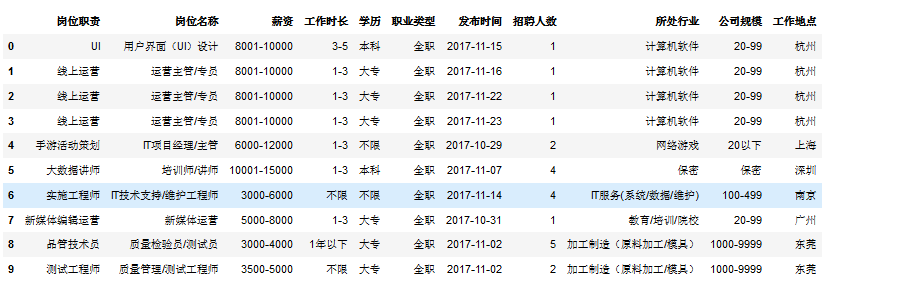
# 查看数据集是否存在异常值
print('最早发布时间:',data['发布时间'].unique().min(),',最晚发布时间:',data['发布时间'].unique().max())
# 可以发现:发布时间异常
最早发布时间:1970-01-01 ,最晚发布时间:2017-11-23
# 查看异常数据
data[(data['发布时间']<'2017-01-01')]
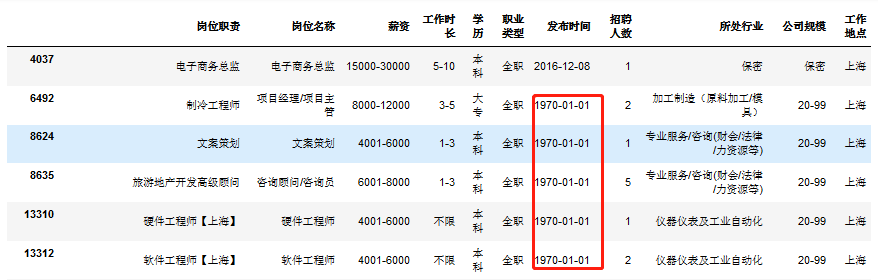
# 仅保留17年及以后的招聘信息
data = data[(data['发布时间']>='2017-01-01')]
# 重复值统计
data.duplicated().sum()
# result
35950
# 去重
data.drop_duplicates(inplace=True)
# 再次查看数据集情况,发现存在空值
data.info()
# result
Int64Index: 1157827 entries, 0 to 1193846
Data columns (total 11 columns):
岗位职责 1157827 non-null object
岗位名称 1157827 non-null object
薪资 1157827 non-null object
工作时长 1157827 non-null object
学历 1157827 non-null object
职业类型 1157827 non-null object
发布时间 1157827 non-null object
招聘人数 1157827 non-null object
所处行业 1157674 non-null object
公司规模 1157667 non-null object
工作地点 1157796 non-null object
dtypes: object(11)
memory usage: 106.0+ MB
# 空值处理:统计空值数量
data.isnull().sum()
# result
岗位职责 0
岗位名称 0
薪资 0
工作时长 0
学历 0
职业类型 0
发布时间 0
招聘人数 0
所处行业 153
公司规模 160
工作地点 31
dtype: int64
# 公司规模列空值较多,可具体查看
data[data['公司规模'].isnull()]
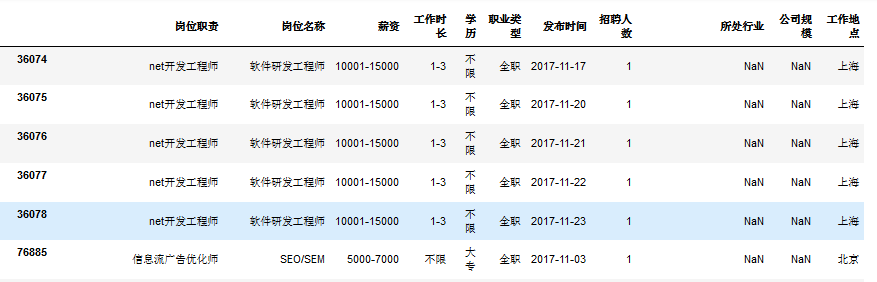
# 删除空值
data.dropna(inplace=True)
# 再次查看,发现所有数据都处理完毕
data.info()
# result
Int64Index: 1157647 entries, 0 to 1193846
Data columns (total 11 columns):
岗位职责 1157647 non-null object
岗位名称 1157647 non-null object
薪资 1157647 non-null object
工作时长 1157647 non-null object
学历 1157647 non-null object
职业类型 1157647 non-null object
发布时间 1157647 non-null object
招聘人数 1157647 non-null object
所处行业 1157647 non-null object
公司规模 1157647 non-null object
工作地点 1157647 non-null object
dtypes: object(11)
memory usage: 106.0+ MB
# 仅选择数据分析师岗位进行分析,大家还可以进行数据分析专员等分析
data_da = data[data['岗位名称']=='数据分析师'].copy() # 不加copy()容易警告:SettingWithCopyWarning
data_da[ data_da['招聘人数']=='若干'] # 为了分析的方便,去掉“若干”情况

# 数据处理,重新赋值
data_da.loc[ data_da['招聘人数']=='若干','招聘人数'] = 0
data_da['招聘人数'].value_counts()
# result1
35313 9575
9492 9346
7224 4328
2427 16210
14512 7830
719 2920
1518 1415
760 40
225 116
113 1
Name: 招聘人数, dtype: int64
# 查看招聘人数
data_da['招聘人数'] = data_da['招聘人数'].astype(int)
grb = data_da.groupby(['工作地点']).agg({'岗位名称':'count','招聘人数':sum}).sort_values(by = '岗位名称',ascending = False).head(10)
grb
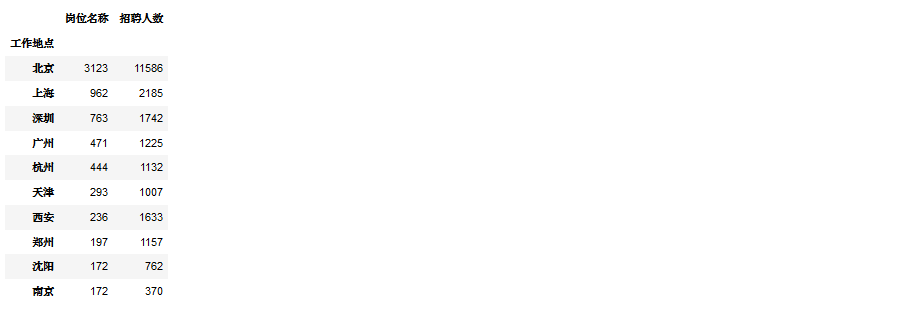
# 绘图说明不同城市对数据分析师的需求数量
grb.plot(kind = 'bar',figsize=(10,5),fontsize=12)
plt.legend(['岗位数量','招聘人数'])
plt.xlabel('工作地点',fontsize=15)
plt.show()
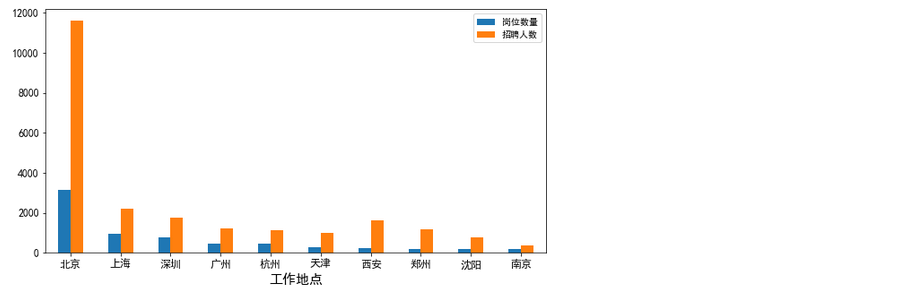
# 查看所处行业情况
data['所处行业'].value_counts().head(10)
# result
互联网/电子商务 267519
计算机软件 261188
IT服务(系统/数据/维护) 95320
教育/培训/院校 94988
专业服务/咨询(财会/法律/力资源等) 52931
媒体/出版/影视/文化传播 49300
基金/证券/期货/投资 32005
电子技术/半导体/集成电路 28652
房地产/建筑/建材/工程 25118
通信/电信/网络设备 24828
Name: 所处行业, dtype: int64
# 筛选出北京地区互联网公司数据分析师招聘数据
subdata = data[data['所处行业'].isin(['互联网/电子商务'])][(data['岗位名称']=='数据分析师')&(data['工作地点']=='北京')]
subdata.iloc[:20,:]
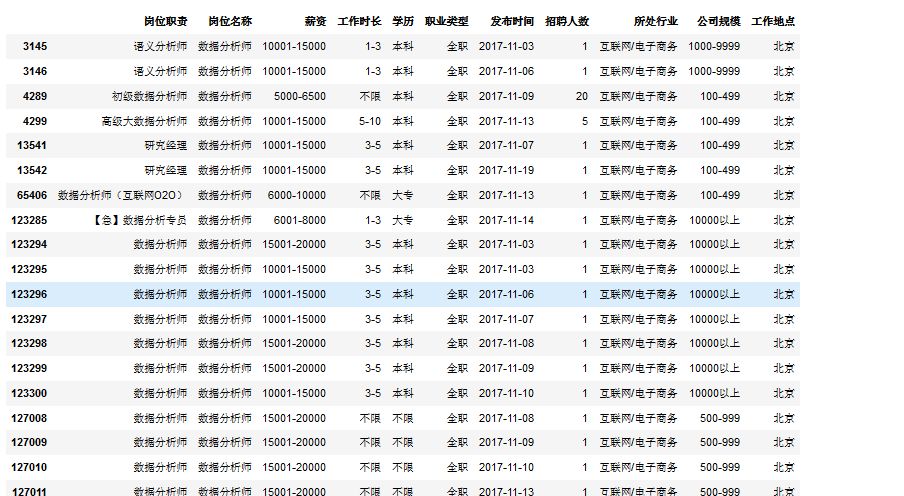
# 学历因素
subdata['学历'].value_counts(normalize = True)
# result
本科 0.568910
硕士 0.174679
大专 0.142628
不限 0.113782
Name: 学历, dtype: float64
# 其他城市可能会用到
-- subdata[subdata['学历'].isin(['中专','中技'])]
-- subdata.loc[(subdata['学历']=='中专')|(subdata['学历']=='中技'),'学历'] = '不限'
# 绘图说明数据分析师对学历的要求
subdata['学历'].value_counts(normalize = True)
plt.pie(subdata['学历'].value_counts(normalize = True),labels = ['本科','硕士','大专','不限'],autopct='%.1f%%',startangle=180)
plt.show()
plt.close()
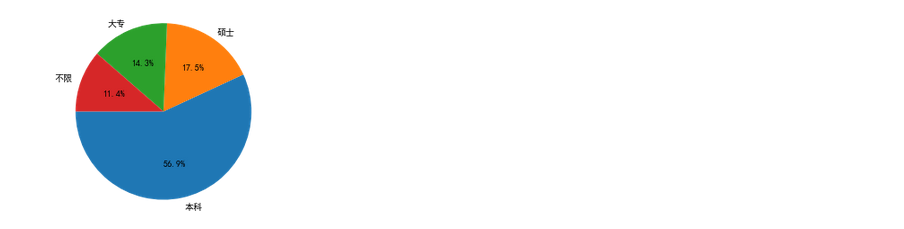
# 薪资情况
subdata.groupby(['学历','工作时长'])[['薪资']].describe()
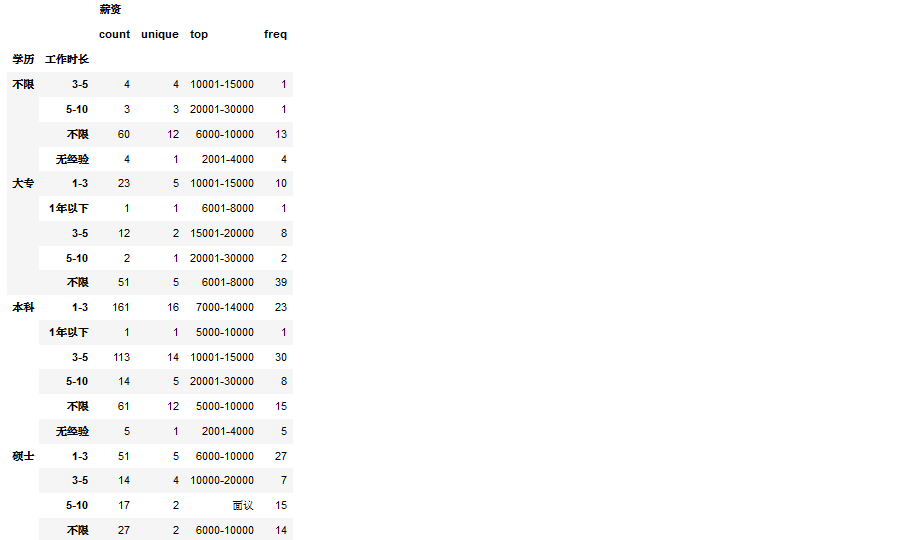
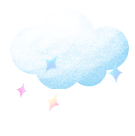
评论