特征选择:11 种特征选择策略总结
数据派THU
共 4612字,需浏览 10分钟
·
2022-05-14 17:55

来源:DeepHub IMBA 本文约4800字,建议阅读10+分钟
本文与你分享可应用于特征选择的各种技术的有用指南。
删除未使用的列 删除具有缺失值的列 不相关的特征 低方差特征 多重共线性 特征系数 p 值 方差膨胀因子 (VIF) 基于特征重要性的特征选择 使用 sci-kit learn 进行自动特征选择 主成分分析 (PCA)
import pandas as pddata = 'https://raw.githubusercontent.com/pycaret/pycaret/master/datasets/automobile.csv'
df = pd.read_csv(data)
df.sample(5)
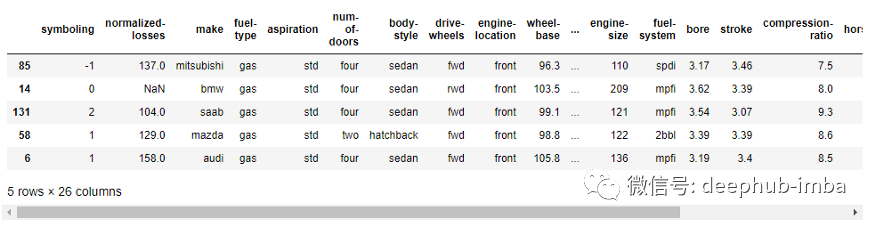
df.columns
>> Index(['symboling', 'normalized-losses', 'make', 'fuel-type', 'aspiration', 'num-of-doors', 'body-style', 'drive-wheels', 'engine-location','wheel-base', 'length', 'width', 'height', 'curb-weight', 'engine-type', 'num-of-cylinders', 'engine-size', 'fuel-system', 'bore', 'stroke', 'compression-ratio', 'horsepower', 'peak-rpm', 'city-mpg', 'highway-mpg', 'price'], dtype='object')
现在让我们深入研究特征选择的 11 种策略。
删除未使用的列
删除具有缺失值的列
# total null values per column
df.isnull().sum()
>>
symboling 0
normalized-losses 35
make 0
fuel-type 0
aspiration 0
num-of-doors 2
body-style 0
drive-wheels 0
engine-location 0
wheel-base 0
length 0
width 0
height 0
curb-weight 0
engine-type 0
num-of-cylinders 0
engine-size 0
fuel-system 0
bore 0
stroke 0
compression-ratio 0
horsepower 0
peak-rpm 0
city-mpg 0
highway-mpg 0
price 0
dtype: int64
不相关的特征
# correlation between target and features
(df.corr().loc['price']
.plot(kind='barh', figsize=(4,10)))
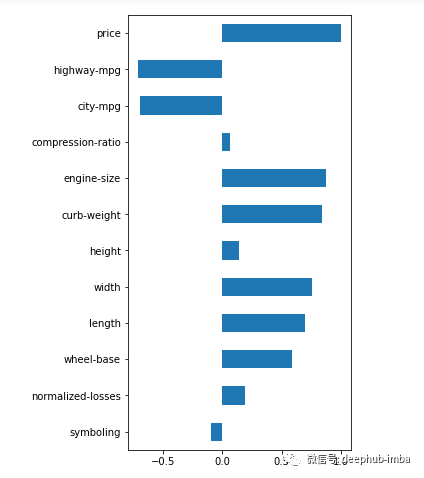
# drop uncorrelated numeric features (threshold <0.2)
corr = abs(df.corr().loc['price'])
corr = corr[corr<0.2]
cols_to_drop = corr.index.to_list()
df = df.drop(cols_to_drop, axis=1)
import seaborn as sns
sns.boxplot(y = 'price', x = 'fuel-type', data=df)
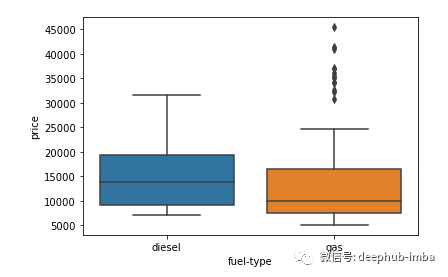
低方差特征
import numpy as np
# variance of numeric features
(df
.select_dtypes(include=np.number)
.var()
.astype('str'))
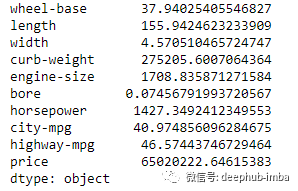
df['bore'].describe()
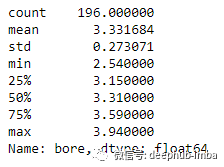
多重共线性
import matplotlib.pyplot as plt
sns.set(rc={'figure.figsize':(16,10)})
sns.heatmap(df.corr(),
annot=True,
linewidths=.5,
center=0,
cbar=False,
cmap="PiYG")
plt.show()
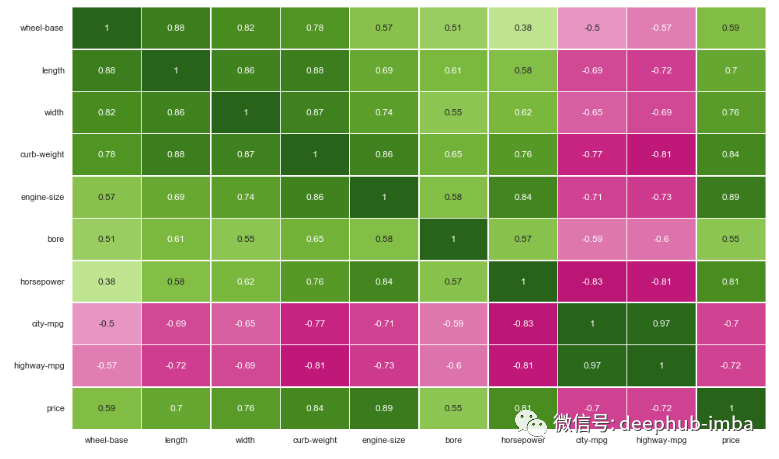
# drop correlated features
df = df.drop(['length', 'width', 'curb-weight', 'engine-size', 'city-mpg'], axis=1)
还可以使用称为方差膨胀因子 (VIF) 的方法来确定多重共线性并根据高 VIF 值删除特征。我稍后会展示这个例子。
df_cat = df[['fuel-type', 'body-style']]
df_cat.sample(5)
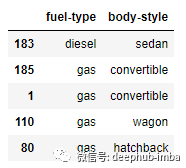
crosstab = pd.crosstab(df_cat['fuel-type'], df_cat['body-style'])
crosstab
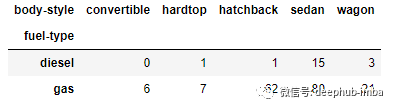
from scipy.stats import chi2_contingency
chi2_contingency(crosstab)

# drop columns with missing values
df = df.dropna()
from sklearn.model_selection import train_test_split
# get dummies for categorical features
df = pd.get_dummies(df, drop_first=True)
# X features
X = df.drop('price', axis=1)
# y target
y = df['price']
# split data into training and testing set
X_train, X_test, y_train, y_test = train_test_split(X, y, test_size=0.3, random_state=42)
from sklearn.linear_model import LinearRegression
# scaling
from sklearn.preprocessing import StandardScaler
scaler = StandardScaler()
X_train = scaler.fit_transform(X_train)
X_test = scaler.fit_transform(X_test)
# convert back to dataframe
X_train = pd.DataFrame(X_train, columns = X.columns.to_list())
X_test = pd.DataFrame(X_test, columns = X.columns.to_list())
# instantiate model
model = LinearRegression()# fit
model.fit(X_train, y_train)
特征系数
# feature coefficients
coeffs = model.coef_
# visualizing coefficients
index = X_train.columns.tolist()
(pd.DataFrame(coeffs, index = index, columns = ['coeff']).sort_values(by = 'coeff')
.plot(kind='barh', figsize=(4,10)))
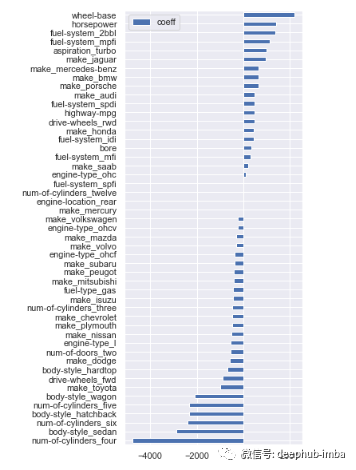
# filter variables near zero coefficient value
temp = pd.DataFrame(coeffs, index = index, columns = ['coeff']).sort_values(by = 'coeff')
temp = temp[(temp['coeff']>1) | (temp['coeff']< -1)]
# drop those features
cols_coeff = temp.index.to_list()
X_train = X_train[cols_coeff]
X_test = X_test[cols_coeff]
p 值
import statsmodels.api as sm
ols = sm.OLS(y, X).fit()
print(ols.summary())
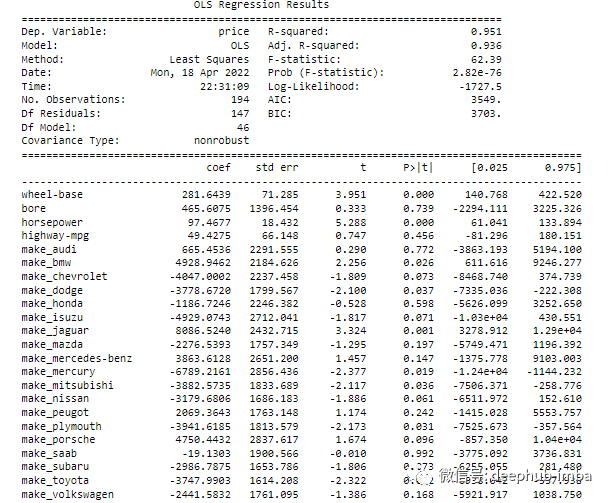
方差膨胀因子 (VIF)
VIF = 1 表示无相关性 VIF = 1-5 中等相关性 VIF >5 高相关
from statsmodels.stats.outliers_influence import variance_inflation_factor
# calculate VIF
vif = pd.Series([variance_inflation_factor(X.values, i) for i in range(X.shape[1])], index=X.columns)
# display VIFs in a table
index = X_train.columns.tolist()
vif_df = pd.DataFrame(vif, index = index, columns = ['vif']).sort_values(by = 'vif', ascending=False)
vif_df[vif_df['vif']<10]
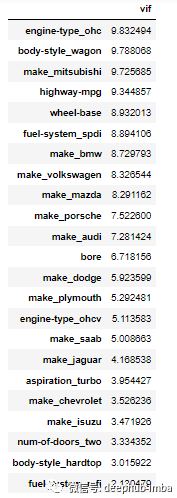
基于特征重要性选择
from sklearn.ensemble import RandomForestClassifier
# instantiate model
model = RandomForestClassifier(n_estimators=200, random_state=0)
# fit model
model.fit(X,y)
importances = model.feature_importances_
cols = X.columns
(pd.DataFrame(importances, cols, columns = ['importance'])
.sort_values(by='importance', ascending=True)
.plot(kind='barh', figsize=(4,10)))
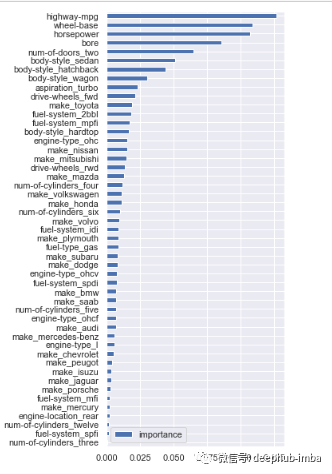
# calculate standard deviation of feature importances
std = np.std([i.feature_importances_ for i in model.estimators_], axis=0)
# visualization
feat_with_importance = pd.Series(importances, X.columns)
fig, ax = plt.subplots(figsize=(12,5))
feat_with_importance.plot.bar(yerr=std, ax=ax)
ax.set_title("Feature importances")
ax.set_ylabel("Mean decrease in impurity")
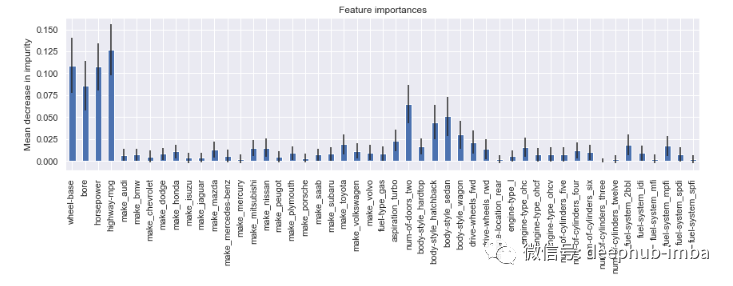
使用 Scikit Learn 自动选择特征
# import modules
from sklearn.feature_selection import (SelectKBest, chi2, SelectPercentile, SelectFromModel, SequentialFeatureSelector, SequentialFeatureSelector)
基于卡方的技术
# select K best features
X_best = SelectKBest(chi2, k=10).fit_transform(X,y)
# number of best features
X_best.shape[1]
>> 10
# keep 75% top features
X_top = SelectPercentile(chi2, percentile = 75).fit_transform(X,y)
# number of best features
X_top.shape[1]
>> 36
# implement algorithm
from sklearn.svm import LinearSVC
model = LinearSVC(penalty= 'l1', C = 0.002, dual=False)
model.fit(X,y)
# select features using the meta transformer
selector = SelectFromModel(estimator = model, prefit=True)
X_new = selector.transform(X)
X_new.shape[1]
>> 2
# names of selected features
feature_names = np.array(X.columns)
feature_names[selector.get_support()]
>> array(['wheel-base', 'horsepower'], dtype=object)
# instantiate model
model = RandomForestClassifier(n_estimators=100, random_state=0)
# select features
selector = SequentialFeatureSelector(estimator=model, n_features_to_select=10, direction='backward', cv=2)
selector.fit_transform(X,y)
# check names of features selected
feature_names = np.array(X.columns)
feature_names[selector.get_support()]
>> array(['bore', 'make_mitsubishi', 'make_nissan', 'make_saab',
'aspiration_turbo', 'num-of-doors_two', 'body style_hatchback', 'engine-type_ohc', 'num-of-cylinders_twelve', 'fuel-system_spdi'], dtype=object)
主成分分析 (PCA)
# import PCA module
from sklearn.decomposition import PCA
# scaling data
X_scaled = scaler.fit_transform(X)
# fit PCA to data
pca = PCA()
pca.fit(X_scaled)
evr = pca.explained_variance_ratio_
# visualizing the variance explained by each principal components
plt.figure(figsize=(12, 5))
plt.plot(range(0, len(evr)), evr.cumsum(), marker="o", linestyle="--")
plt.xlabel("Number of components")
plt.ylabel("Cumulative explained variance")
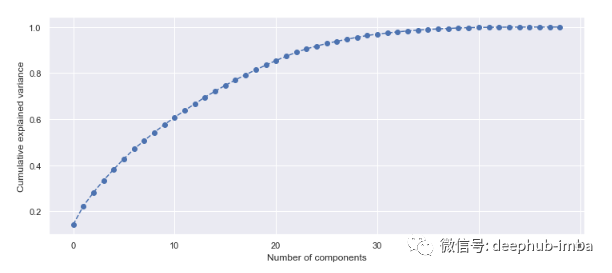
总结
评论