21 句话入门机器学习!
机器学习算法与Python实战
共 22428字,需浏览 45分钟
·
2023-10-17 10:13
对于程序员来说,机器学习的重要性毋庸赘言。也许你还没有开始,也许曾经失败过,都没有关系,你将在这里找到或者重拾自信。只要粗通Python,略知NumPy,认真读完这21句话,逐行敲完示例代码,就可以由此进入自由的AI王国。
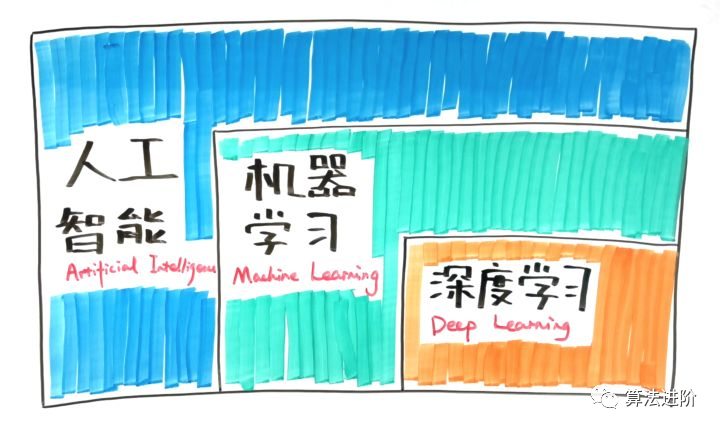
分类是对个体样本做出定性判定,回归是对个体样本做出定量判定,二者同属于有监督的学习,都是基于经验的。举个例子:有经验的老师预测某学生考试及格或不及格,这是分类;预测某学生能考多少分,这是回归;不管是预测是否及格还是预测考多少分,老师的经验数据和思考方法是相同的,只是最后的表述不同而已。
4
as np numpy
members = np.array([
['男', '25', 185, 80, '程序员', 35, 200, 30],
['女', '23', 170, 55, '公务员', 15, 0, 80],
['男', '30', 180, 82, '律师', 60, 260, 300],
['女', '27', 168, 52, '记者', 20, 180, 150]
])
6
:,-1])) # 提取有价证券特征列数据 > security = np.float32((members[
> security
array([ 30., 80., 300., 150.], dtype=float32)
# 减去均值再除以标准差 > (security - security.mean())/security.std()
array([-1.081241, -0.5897678, 1.5727142, 0.09829464], dtype=float32)
9
归一化是对样本集的每个特征列减去该特征列的最小值进行中心化,再除以极差(最大值最小值之差)进行缩放。
:,-1])) # 提取有价证券特征列数据 > security = np.float32((members[
> security
array([ 30., 80., 300., 150.], dtype=float32)
# 减去最小值再除以极差 > (security - security.min())/(security.max() - security.min())
array([0., 0.18518518, 1., 0.44444445], dtype=float32)
> from sklearn import preprocessing as pp
> X = [
['男', '程序员'],
['女', '公务员'],
['男', '律师', ],
['女', '记者', ]
]
> ohe = pp.OneHotEncoder().fit(X)
> ohe.transform(X).toarray()
array([[0., 1., 0., 0., 1., 0.],
[1., 0., 1., 0., 0., 0.],
[0., 1., 0., 1., 0., 0.],
[1., 0., 0., 0., 0., 1.]])
11
datasets.load_boston([return_X_y]) :加载波士顿房价数据集
datasets.load_breast_cancer([return_X_y]) :加载威斯康星州乳腺癌数据集
datasets.load_diabetes([return_X_y]) :加载糖尿病数据集
datasets.load_digits([n_class, return_X_y]) :加载数字数据集
datasets.load_iris([return_X_y]) :加载鸢尾花数据集。
datasets.load_linnerud([return_X_y]) :加载体能训练数据集
datasets.load_wine([return_X_y]) :加载葡萄酒数据集
datasets.fetch_20newsgroups([data_home, …]) :加载新闻文本分类数据集
datasets.fetch_20newsgroups_vectorized([…]) :加载新闻文本向量化数据集
datasets.fetch_california_housing([…]) :加载加利福尼亚住房数据集
datasets.fetch_covtype([data_home, …]) :加载森林植被数据集
datasets.fetch_kddcup99([subset, data_home, …]) :加载网络入侵检测数据集
datasets.fetch_lfw_pairs([subset, …]) :加载人脸(成对)数据集
datasets.fetch_lfw_people([data_home, …]) :加载人脸(带标签)数据集
datasets.fetch_olivetti_faces([data_home, …]) :加载 Olivetti 人脸数据集
datasets.fetch_rcv1([data_home, subset, …]):加载路透社英文新闻文本分类数据集
datasets.fetch_species_distributions([…]) :加载物种分布数据集
>>> from sklearn.datasets import load_iris
>>> X, y = load_iris(return_X_y=True)
>>> X.shape # 数据集X有150个样本,4个特征列
(150, 4)
>>> y.shape # 标签集y的每一个标签和数据集X的每一个样本一一对应
(150,)
>>> X[0], y[0]
(array([5.1, 3.5, 1.4, 0.2]), 0)
> iris = load_iris()
# 查看标签的名字 > iris.target_names
array(['setosa', 'versicolor', 'virginica'], dtype='<U10')
> X = iris.data
> y = iris.target
>>> from sklearn.datasets import load_iris
>>> from sklearn.model_selection import train_test_split as tsplit
>>> X, y = load_iris(return_X_y=True)
>>> X_train, X_test, y_train, y_test = tsplit(X, y, test_size=0.1)
>>> X_train.shape, X_test.shape
((135, 4), (15, 4))
>>> y_train.shape, y_test.shape
((135,), (15,))
> from sklearn.datasets import load_iris
> from sklearn.model_selection import train_test_split as tsplit
# 导入k-近邻分类模型 > from sklearn.neighbors import KNeighborsClassifier
# 获取鸢尾花数据集,返回样本集和标签集 > X, y = load_iris(return_X_y=True)
0.1) # 拆分为训练集和测试集 > X_train, X_test, y_train, y_test = tsplit(X, y, test_size=
10) # 模型实例化,n_neighbors参数指定k值,默认k=5 > m = KNeighborsClassifier(n_neighbors=
# 模型训练 > m.fit(X_train, y_train)
KNeighborsClassifier()
# 对测试集分类 > m.predict(X_test)
array([2, 1, 2, 2, 1, 2, 1, 2, 2, 1, 0, 1, 0, 0, 2])
# 这是实际的分类情况,上面的预测只错了一个 > y_test
array([2, 1, 2, 2, 2, 2, 1, 2, 2, 1, 0, 1, 0, 0, 2])
# 模型测试精度(介于0~1) > m.score(X_test, y_test)
0.9333333333333333
> from sklearn.datasets import load_boston
> from sklearn.model_selection import train_test_split as tsplit
> from sklearn.neighbors import KNeighborsRegressor
# 加载波士顿房价数据集 > X, y = load_boston(return_X_y=True)
# 该数据集共有506个样本,13个特征列,标签集为浮点型,适用于回归模型 > X.shape, y.shape, y.dtype
((506, 13), (506,), dtype('float64'))
0.01) # 拆分为训练集和测试集 > X_train, X_test, y_train, y_test = tsplit(X, y, test_size=
10) # 模型实例化,n_neighbors参数指定k值,默认k=5 > m = KNeighborsRegressor(n_neighbors=
# 模型训练 > m.fit(X_train, y_train)
KNeighborsRegressor(n_neighbors=10)
# 预测6个测试样本的房价 > m.predict(X_test)
array([27.15, 31.97, 12.68, 28.52, 20.59, 21.47])
# 这是测试样本的实际价格,除了第2个(索引为1)样本偏差较大,其他样本偏差还算差强人意 > y_test
array([29.1, 50. , 12.7, 22.8, 20.4, 21.5])
> from sklearn import metrics
> y_pred = m.predict(X_test)
# 均方误差 > metrics.mean_squared_error(y_test, y_pred)
60.27319999999995
# 中位数绝对误差 > metrics.median_absolute_error(y_test, y_pred)
1.0700000000000003
# 复相关系数 > metrics.r2_score(y_test, y_pred)
0.5612816401629652
> from sklearn.datasets import load_boston
> from sklearn.model_selection import train_test_split as tsplit
> from sklearn.tree import DecisionTreeRegressor
# 加载波士顿房价数据集 > X, y = load_boston(return_X_y=True)
0.01) # 拆分为训练集和测试集 > X_train, X_test, y_train, y_test = tsplit(X, y, test_size=
10) # 实例化模型,决策树深度为10 > m = DecisionTreeRegressor(max_depth=
# 训练 > m.fit(X, y)
DecisionTreeRegressor(max_depth=10)
# 预测 > y_pred = m.predict(X_test)
# 这是测试样本的实际价格,除了第2个(索引为1)样本偏差略大,其他样本偏差较小 > y_test
array([20.4, 21.9, 13.8, 22.4, 13.1, 7. ])
# 这是6个测试样本的预测房价,非常接近实际价格 > y_pred
array([20.14, 22.33, 14.34, 22.4, 14.62, 7. ])
# 复相关系数 > metrics.r2_score(y_test, y_pred)
0.9848774474870712
# 均方误差 > metrics.mean_squared_error(y_test, y_pred)
0.4744784865112032
# 中位数绝对误差 > metrics.median_absolute_error(y_test, y_pred)
0.3462962962962983
> from sklearn.datasets import load_diabetes
> from sklearn.model_selection import train_test_split as tsplit
> from sklearn.svm import SVR
> from sklearn import metrics
> X, y = load_diabetes(return_X_y=True)
> X.shape, y.shape, y.dtype
((442, 10), (442,), dtype('float64'))
0.02) > X_train, X_test, y_train, y_test = tsplit(X, y, test_size=
'rbf', C=0.1) # 实例化SVR模型,rbf核函数,C=0.1 > svr_1 = SVR(kernel=
'rbf', C=100) # 实例化SVR模型,rbf核函数,C=100 > svr_2 = SVR(kernel=
# 模型训练 > svr_1.fit(X_train, y_train)
SVR(C=0.1)
# 模型训练 > svr_2.fit(X_train, y_train)
SVR(C=100)
# 模型预测 > z_1 = svr_1.predict(X_test)
# 模型预测 > z_2 = svr_2.predict(X_test)
# 这是测试集的实际值 > y_test
array([ 49., 317., 84., 181., 281., 198., 84., 52., 129.])
# 这是C=0.1的预测值,偏差很大 > z_1
array([138.10720127, 142.1545034 , 141.25165838, 142.28652449,
143.19648143, 143.24670732, 137.57932272, 140.51891989,
143.24486911])
# 这是C=100的预测值,偏差明显变小 > z_2
array([ 54.38891948, 264.1433666 , 169.71195204, 177.28782561,
283.65199575, 196.53405477, 61.31486045, 199.30275061,
184.94923477])
# C=0.01的均方误差 > metrics.mean_squared_error(y_test, z_1)
8464.946517460194
# C=100的均方误差 > metrics.mean_squared_error(y_test, z_2)
3948.37754995066
# C=0.01的复相关系数 > metrics.r2_score(y_test, z_1)
0.013199351909129464
# C=100的复相关系数 > metrics.r2_score(y_test, z_2)
0.5397181166871942
# C=0.01的中位数绝对误差 > metrics.median_absolute_error(y_test, z_1)
57.25165837797314
# C=100的中位数绝对误差 > metrics.median_absolute_error(y_test, z_2)
22.68513954888364
>>> from sklearn.datasets import load_breast_cancer # 导入数据加载函数
>>> from sklearn.tree import DecisionTreeClassifier # 导入随机树
>>> from sklearn.ensemble import RandomForestClassifier # 导入随机森林
>>> from sklearn.model_selection import cross_val_score # 导入交叉验证
>>> ds = load_breast_cancer() # 加载威斯康星州乳腺癌数据集
>>> ds.data.shape # 569个乳腺癌样本,每个样本包含30个特征
(569, 30)
>>> dtc = DecisionTreeClassifier() # 实例化决策树分类模型
>>> rfc = RandomForestClassifier() # 实例化随机森林分类模型
>>> dtc_scroe = cross_val_score(dtc, ds.data, ds.target, cv=10) # 交叉验证
>>> dtc_scroe # 决策树分类模型交叉验证10次的结果
array([0.92982456, 0.85964912, 0.92982456, 0.89473684, 0.92982456,
0.89473684, 0.87719298, 0.94736842, 0.92982456, 0.92857143])
>>> dtc_scroe.mean() # 决策树分类模型交叉验证10次的平均精度
0.9121553884711779
>>> rfc_scroe = cross_val_score(rfc, ds.data, ds.target, cv=10) # 交叉验证
>>> rfc_scroe # 随机森林分类模型交叉验证10次的结果
array([0.98245614, 0.89473684, 0.94736842, 0.94736842, 0.98245614,
0.98245614, 0.94736842, 0.98245614, 0.94736842, 1. ])
>>> rfc_scroe.mean()# 随机森林分类模型交叉验证10次的平均精度
0.9614035087719298
# 导入样本生成器 > from sklearn import datasets as dss
# 从聚类子模块导入聚类模型 > from sklearn.cluster import KMeans
> import matplotlib.pyplot as plt
'font.sans-serif'] = ['FangSong'] > plt.rcParams[
'axes.unicode_minus'] = False > plt.rcParams[
300,400,300], n_features=2) > X_blob, y_blob = dss.make_blobs(n_samples=[
1000, noise=0.05, factor=0.5) > X_circle, y_circle = dss.make_circles(n_samples=
1000, noise=0.05) > X_moon, y_moon = dss.make_moons(n_samples=
'k-means++', n_clusters=3).fit_predict(X_blob) > y_blob_pred = KMeans(init=
'k-means++', n_clusters=2).fit_predict(X_circle) > y_circle_pred = KMeans(init=
'k-means++', n_clusters=2).fit_predict(X_moon) > y_moon_pred = KMeans(init=
131) > plt.subplot(
<matplotlib.axes._subplots.AxesSubplot object at 0x00000180AFDECB88>
'团状簇') > plt.title(
Text(0.5, 1.0, '团状簇')
:,0], X_blob[:,1], c=y_blob_pred) > plt.scatter(X_blob[
<matplotlib.collections.PathCollection object at 0x00000180C495DF08>
132) > plt.subplot(
<matplotlib.axes._subplots.AxesSubplot object at 0x00000180C493FA08>
'环状簇') > plt.title(
Text(0.5, 1.0, '环状簇')
:,0], X_circle[:,1], c=y_circle_pred) > plt.scatter(X_circle[
<matplotlib.collections.PathCollection object at 0x00000180C499B888>
133) > plt.subplot(
<matplotlib.axes._subplots.AxesSubplot object at 0x00000180C4981188>
'新月簇') > plt.title(
Text(0.5, 1.0, '新月簇')
:,0], X_moon[:,1], c=y_moon_pred) > plt.scatter(X_moon[
<matplotlib.collections.PathCollection object at 0x00000180C49DD1C8>
> plt.show()
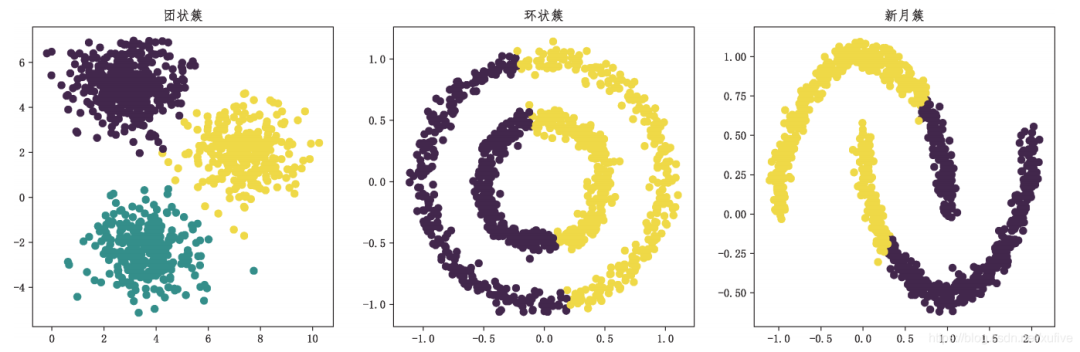
> from sklearn import datasets as dss
> from sklearn.cluster import DBSCAN
> import matplotlib.pyplot as plt
'font.sans-serif'] = ['FangSong'] > plt.rcParams[
'axes.unicode_minus'] = False > plt.rcParams[
1000, noise=0.05) > X, y = dss.make_moons(n_samples=
# 默认核心样本半径0.5,核心样本邻居5个 > dbs_1 = DBSCAN()
0.2) # 核心样本半径0.2,核心样本邻居5个 > dbs_2 = DBSCAN(eps=
0.1) # 核心样本半径0.1,核心样本邻居5个 > dbs_3 = DBSCAN(eps=
> dbs_1.fit(X)
DBSCAN(algorithm='auto', eps=0.5, leaf_size=30, metric='euclidean',
metric_params=None, min_samples=5, n_jobs=None, p=None)
> dbs_2.fit(X)
DBSCAN(algorithm='auto', eps=0.2, leaf_size=30, metric='euclidean',
metric_params=None, min_samples=5, n_jobs=None, p=None)
> dbs_3.fit(X)
DBSCAN(algorithm='auto', eps=0.1, leaf_size=30, metric='euclidean',
metric_params=None, min_samples=5, n_jobs=None, p=None)
131) > plt.subplot(
<matplotlib.axes._subplots.AxesSubplot object at 0x00000180C4C5D708>
'eps=0.5') > plt.title(
Text(0.5, 1.0, 'eps=0.5')
:,0], X[:,1], c=dbs_1.labels_) > plt.scatter(X[
<matplotlib.collections.PathCollection object at 0x00000180C4C46348>
132) > plt.subplot(
<matplotlib.axes._subplots.AxesSubplot object at 0x00000180C4C462C8>
'eps=0.2') > plt.title(
Text(0.5, 1.0, 'eps=0.2')
:,0], X[:,1], c=dbs_2.labels_) > plt.scatter(X[
<matplotlib.collections.PathCollection object at 0x00000180C49FC8C8>
133) > plt.subplot(
<matplotlib.axes._subplots.AxesSubplot object at 0x00000180C49FCC08>
'eps=0.1') > plt.title(
Text(0.5, 1.0, 'eps=0.1')
:,0], X[:,1], c=dbs_3.labels_) > plt.scatter(X[
<matplotlib.collections.PathCollection object at 0x00000180C49FC4C8>
> plt.show()
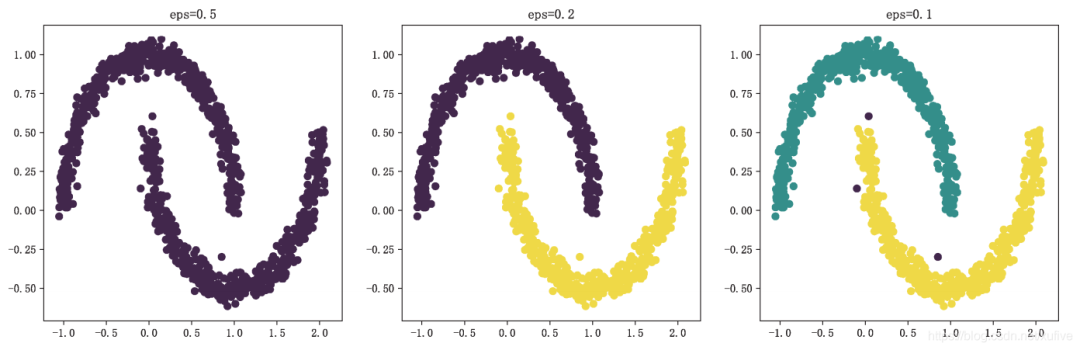
import datasets as dss sklearn
import PCA sklearn.decomposition
ds = dss.load_iris()
# 150个样本,4个特征维 ds.data.shape
(150, 4)
# 使用默认参数实例化PCA类,n_components=None m = PCA()
m.fit(ds.data)
PCA(copy=True, iterated_power='auto', n_components=None, random_state=None,
svd_solver='auto', tol=0.0, whiten=False)
# 正交变换后各成分的方差值 m.explained_variance_
array([4.22824171, 0.24267075, 0.0782095 , 0.02383509])
# 正交变换后各成分的方差值占总方差值的比例 m.explained_variance_ratio_
array([0.92461872, 0.05306648, 0.01710261, 0.00521218])
对鸢尾花数据集的主成分分析结果显示:存在一个明显的成分,其方差值占总方差值的比例超过92% ;存在一个方差值很小的成分,其方差值占总方差值的比例只有0.52% ;前两个成分贡献的方差占比超过97.7%,数据集特征列可以从4个降至2个而不至于损失太多有效信息。
0.97) > m = PCA(n_components=
> m.fit(ds.data)
PCA(copy=True, iterated_power='auto', n_components=0.97, random_state=None,
svd_solver='auto', tol=0.0, whiten=False)
_ > m.explained_variance
array([4.22824171, 0.24267075])
_ > m.explained_variance_ratio
array([0.92461872, 0.05306648])
> d = m.transform(ds.data)
> d.shape
(150, 2)
> import matplotlib.pyplot as plt
:,0], d[:,1], c=ds.target) > plt.scatter(d[
<matplotlib.collections.PathCollection object at 0x0000016FBF243CC8>
> plt.show()
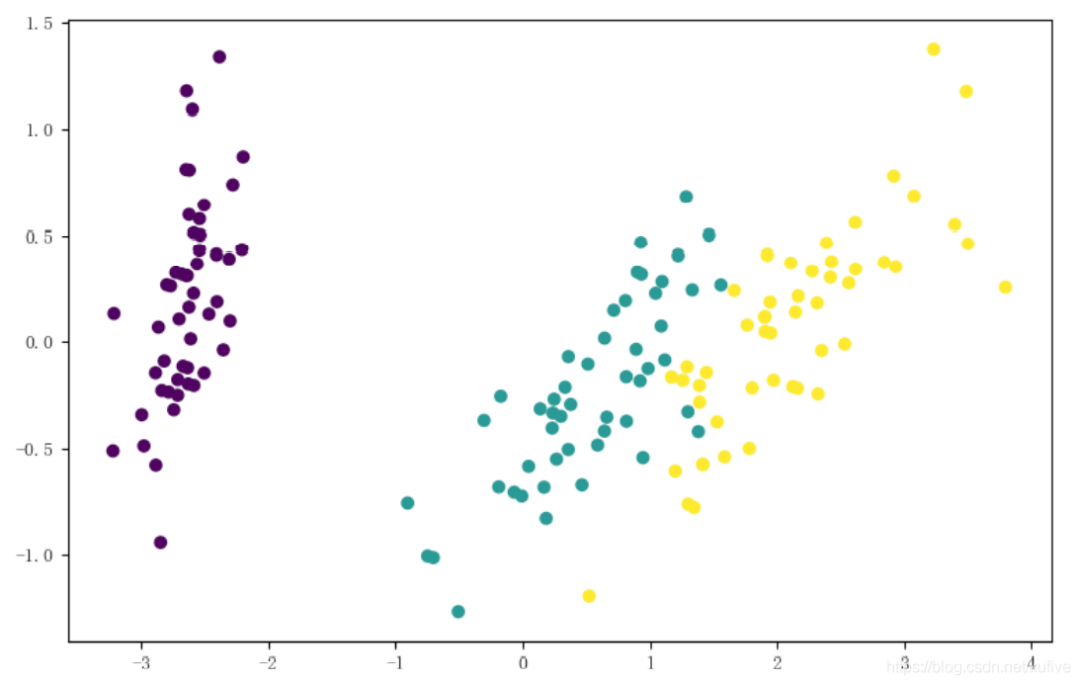
可能是全网最全的速查表:Python机器学习ChatGPT线性代数微积分概率统计
评论