盘点 23 款神经网络的设计和可视化工具
极市平台
共 8486字,需浏览 17分钟
·
2020-09-25 03:01
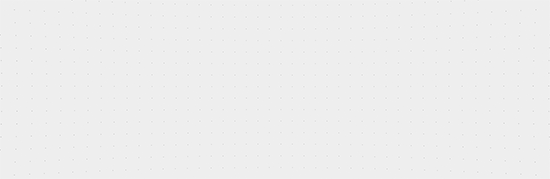
极市导读
本文汇总了23个令人眼前一亮的神经网络的设计和可视化工具,并对其进行了简要介绍。>>加入极市CV技术交流群,走在计算机视觉的最前沿
前言
draw_convnet NNSVG PlotNeuralNet TensorBoard Caffe Matlab Keras.js Keras-sequential-ascii Netron DotNet Graphviz Keras Visualization Conx ENNUI NNet GraphCore Neataptic TensorSpace Netscope CNN Analyzer Monial Texample Quiver Net2Vis
工具
1. draw_convnet
2. NNSVG
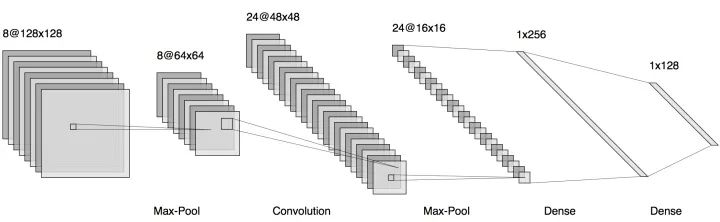
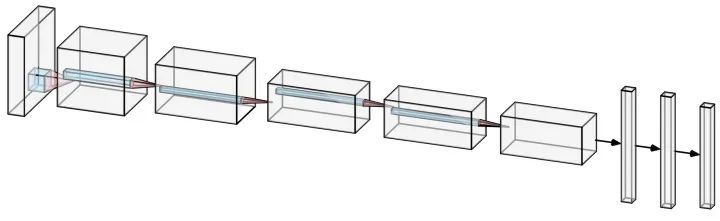
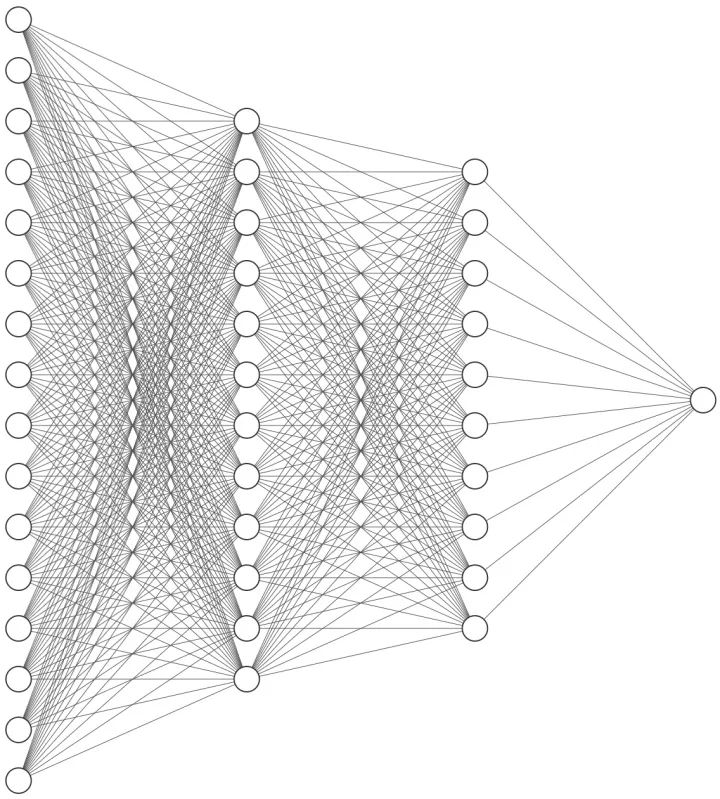
3. PlotNeuralNet
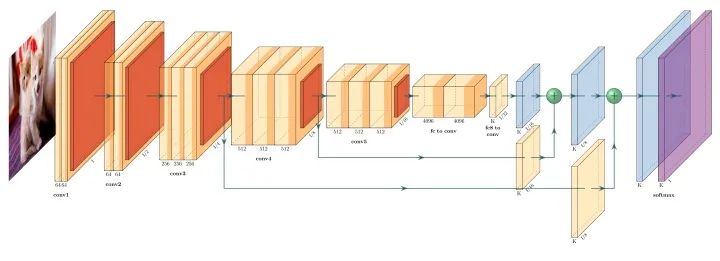
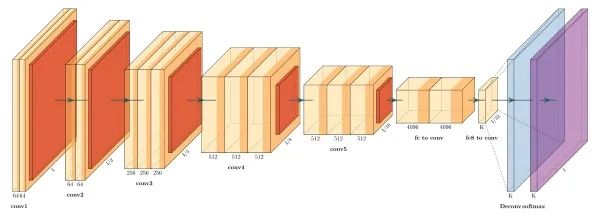
安装
sudo apt-get install texlive-latex-extra
sudo apt-get install texlive-latex-base
sudo apt-get install texlive-fonts-recommended
sudo apt-get install texlive-fonts-extra
sudo apt-get install texlive-latex-extra
首先下载并安装 MikTex,下载网站:https://miktex.org/download 其次,下载并安装 windows 的 bash 运行器,推荐这两个:
Git:https://git-scm.com/download/win Cygwin:https://www.cygwin.com/
使用例子
cd pyexamples/
bash ../tikzmake.sh test_simple
先创建新的文件夹,并生成一个新的 python 代码文件:
mkdir my_project
cd my_project
vim my_arch.py
然后在新的代码文件 my_arch.py
中添加这段代码,用于定义你的网络结构,主要是不同类型网络层的参数,包括输入输出数量、卷积核数量等
import sys
sys.path.append('../')
from pycore.tikzeng import *
# defined your arch
arch = [
to_head( '..' ),
to_cor(),
to_begin(),
to_Conv("conv1", 512, 64, offset="(0,0,0)", to="(0,0,0)", height=64, depth=64, width=2 ),
to_Pool("pool1", offset="(0,0,0)", to="(conv1-east)"),
to_Conv("conv2", 128, 64, offset="(1,0,0)", to="(pool1-east)", height=32, depth=32, width=2 ),
to_connection( "pool1", "conv2"),
to_Pool("pool2", offset="(0,0,0)", to="(conv2-east)", height=28, depth=28, width=1),
to_SoftMax("soft1", 10 ,"(3,0,0)", "(pool1-east)", caption="SOFT" ),
to_connection("pool2", "soft1"),
to_end()
]
def main():
namefile = str(sys.argv[0]).split('.')[0]
to_generate(arch, namefile + '.tex' )
if __name__ == '__main__':
main()
bash ../tikzmake.sh my_arch
4. TensorBoard
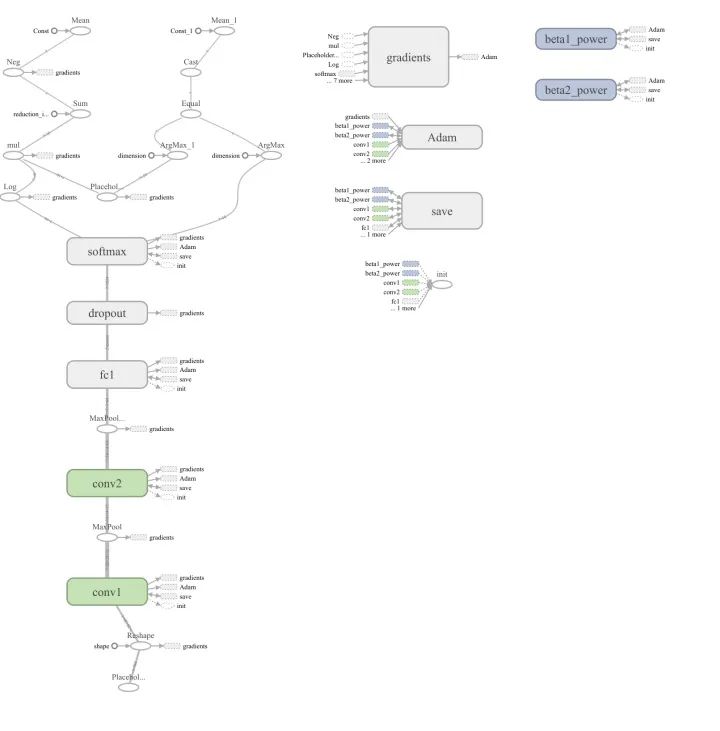
5. Caffe
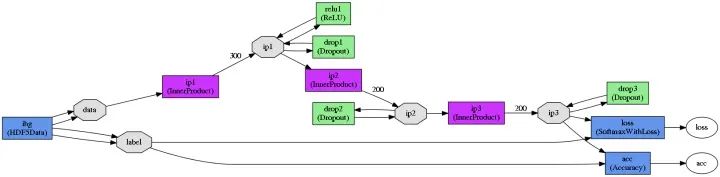
6. Matlab
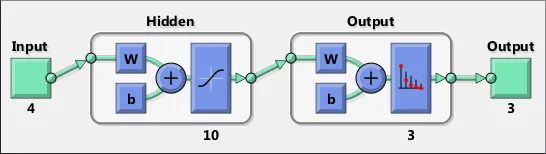
7. Keras.js
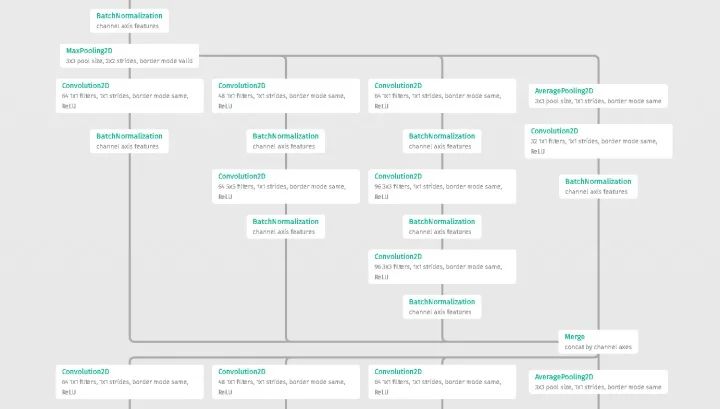
8. keras-sequential-ascii
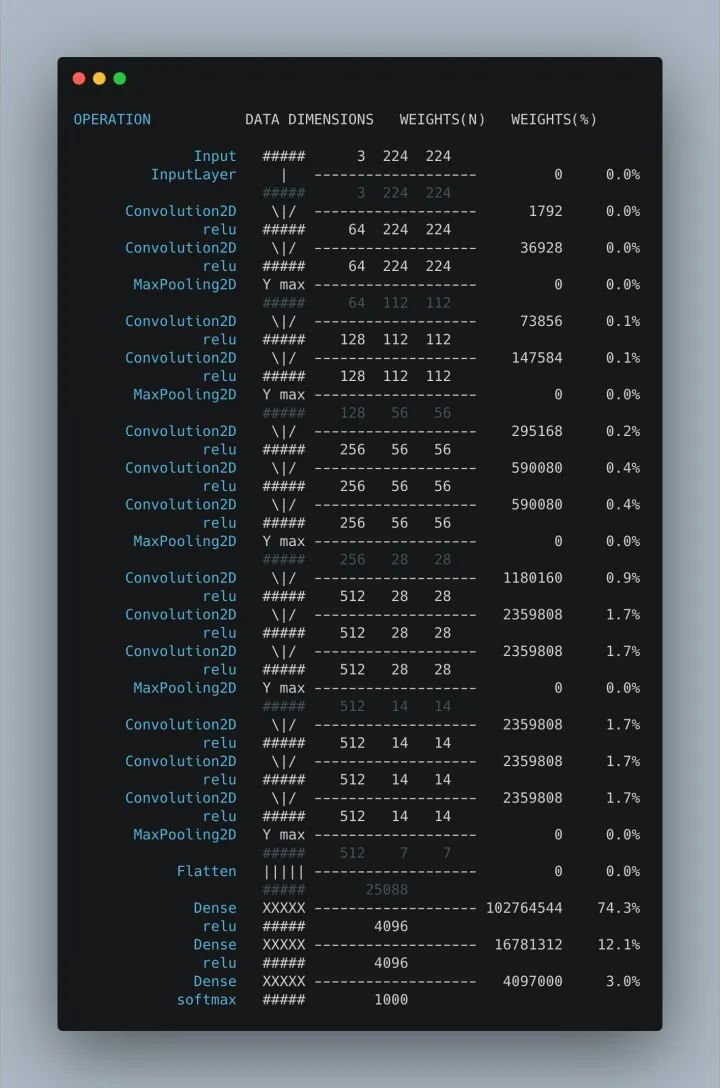
安装
pip install keras_sequential_ascii
pip install git+git://github.com/stared/keras-sequential-ascii.git
使用例子
from keras_sequential_ascii import keras2ascii
keras2ascii(model)
9. Netron
简介
ONNX: .onnx, .pb, .pbtxt
文件Keras: .h5,.keras
文件Core ML: .mlmodel
Caffe: .caffemodel, .prototxt
Caffe2: predict_net.pb, predict_net.pbtxt
Darknet: .cfg
MXNet: .model, -symbol.json
ncnn: .param
TensorFlow Lite: .tflite
TorchScript: .pt, .pth
PyTorch: .pt, .pth
Torch: .t7
Arm NN: .armnn
Barracuda: .nn
BigDL .bigdl
,.model
Chainer : .npz
,.h5
CNTK : .model
,.cntk
Deeplearning4j: .zip
MediaPipe: .pbtxt
http://ML.NET: .zip
MNN: .mnn
OpenVINO : .xml
PaddlePaddle : .zip
,__model__
scikit-learn : .pkl
Tengine : .tmfile
TensorFlow.js : model.json
,.pb
TensorFlow : .pb
,.meta
,.pbtxt
,.ckpt
,.index
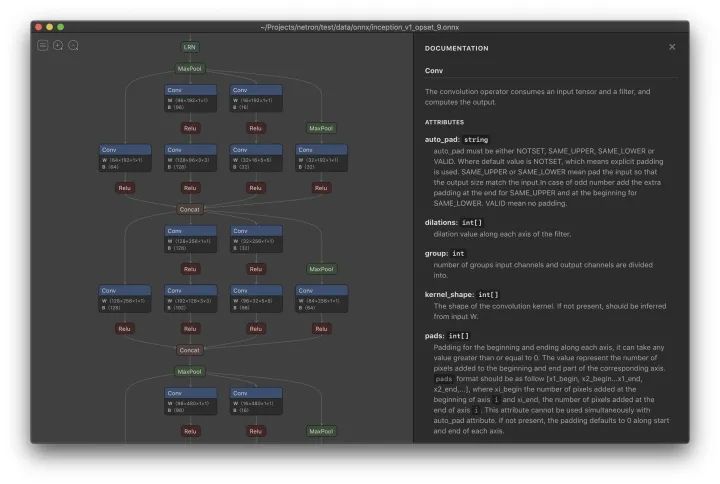
安装
下载 .dmg
文件,地址:https://github.com/lutzroeder/netron/releases/latest运行命令 brew cask install netron
下载 .AppImage
文件,下载地址:https://github.com/lutzroeder/netron/releases/latest运行命令 snap install netron
下载 .exe
文件,下载地址:https://github.com/lutzroeder/netron/releases/latest运行命令 winget install netron
pip install netron
,然后使用方法有两种:命令行,运行 netron [文件路径]
.py
代码中加入
import netron;
netron.start('文件路径')
10. DotNet
Graphviz
生成神经网络的图片。主要参考了文章:https://tgmstat.wordpress.com/2013/06/12/draw-neural-network-diagrams-graphviz/python dotnets.py | dot -Tpng | open -f -a /Applications/Preview.app
python dotnets.py | dot -Tpdf > test.pdf
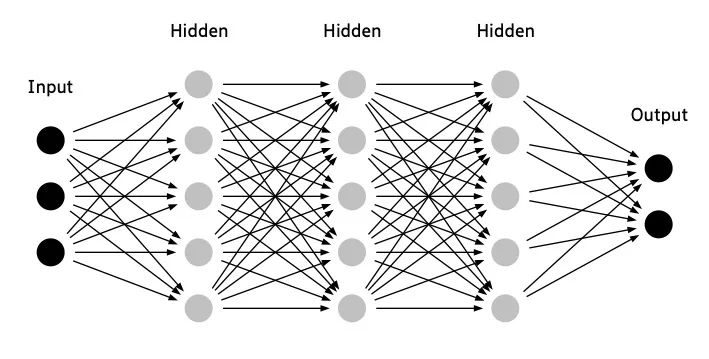
11. Graphviz
Graphviz
是一个开源的图可视化软件,它可以用抽象的图形和网络图来表示结构化信息。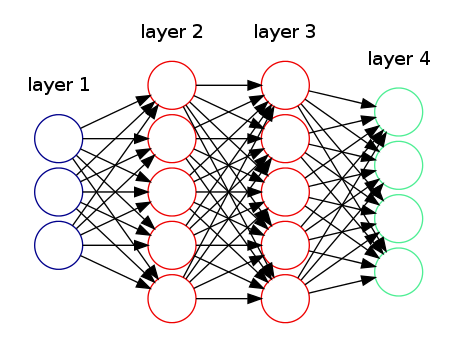
12. Keras Visualization
keras.utils.vis_utils
提供的绘制 Keras 网络模型(使用的是 graphviz
)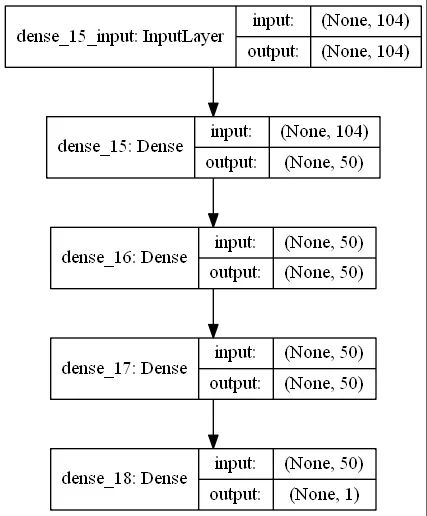
13. Conx
conx
可以通过函数net.picture()
来实现对带有激活函数网络的可视化,可以输出图片格式包括 SVG, PNG 或者是 PIL。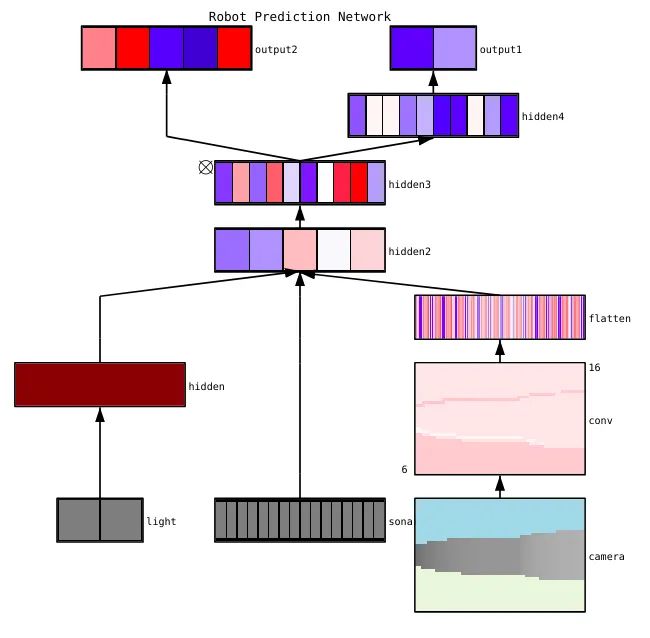
14. ENNUI
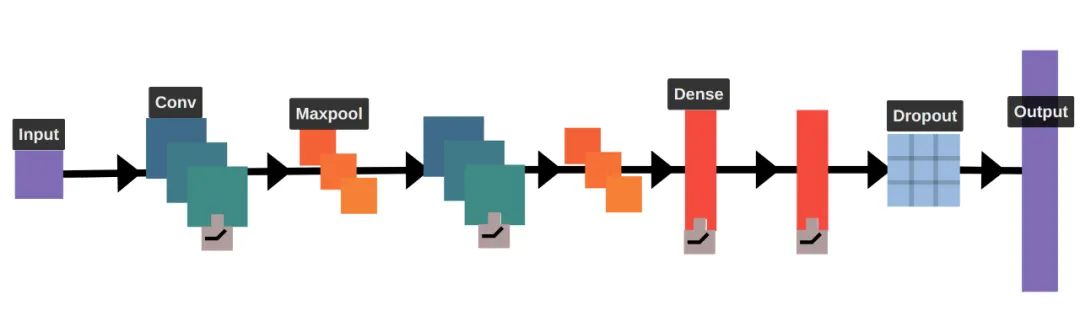
15. NNet
data(infert, package="datasets")
plot(neuralnet(case~parity+induced+spontaneous, infert))
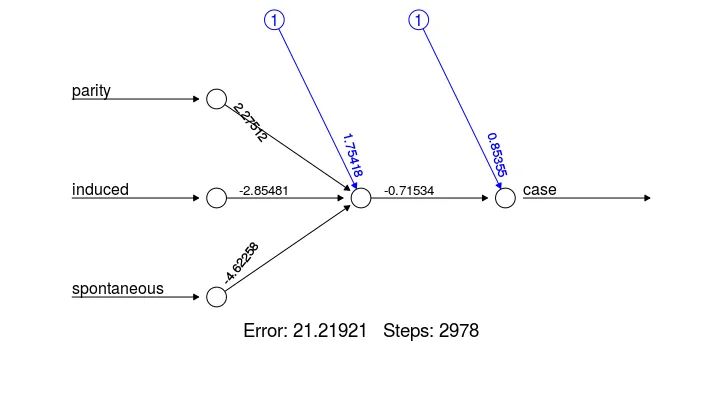
16. GraphCore
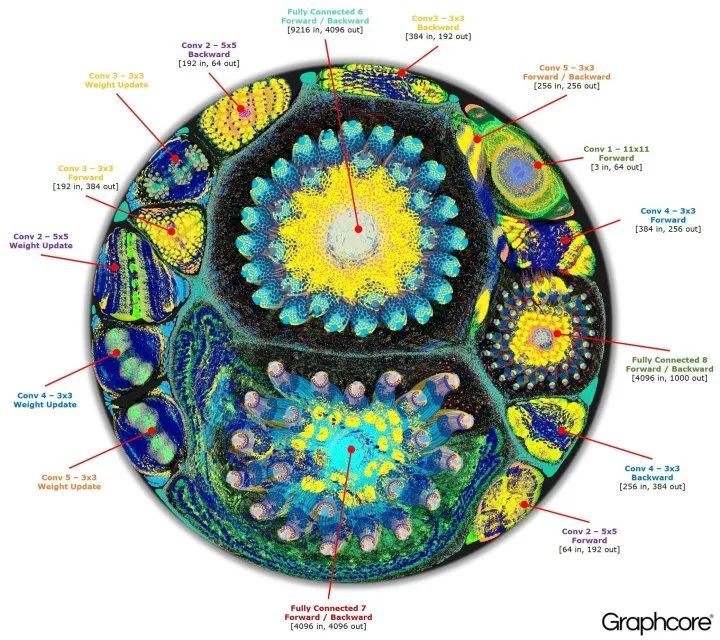
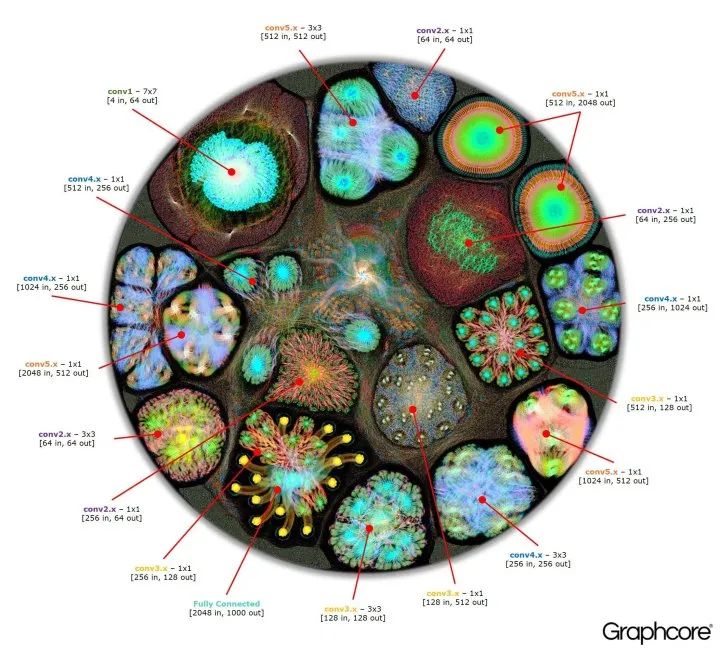
17. Neataptic
神经元和突触可以通过一行代码进行删除; 没有规定神经网络的结构必须包含哪些内容
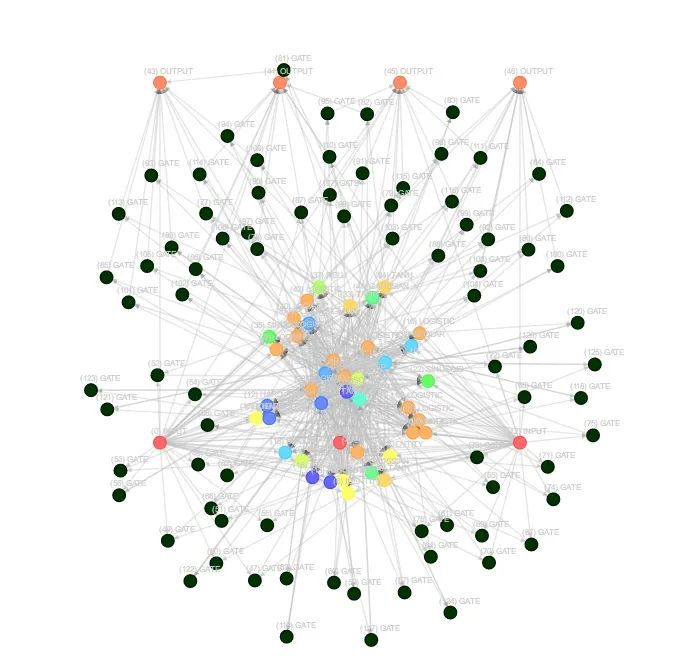
18. TensorSpace
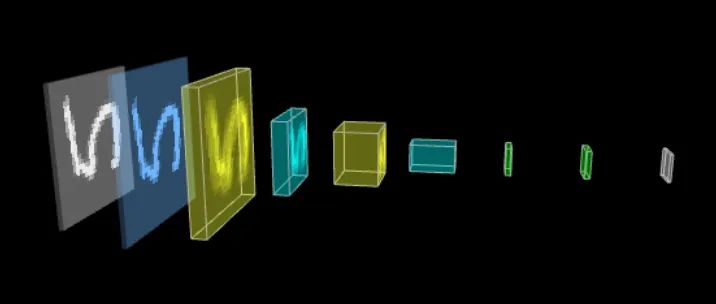
19. Netscope CNN Analyzer
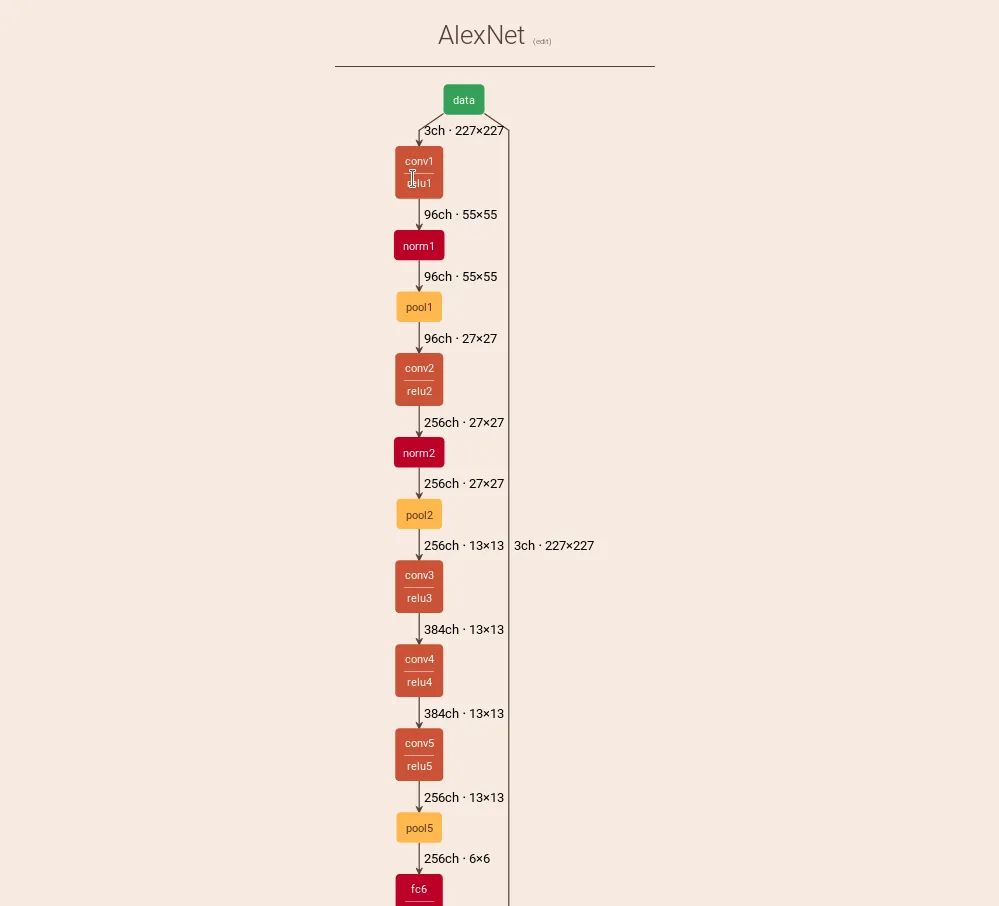
20. Monial
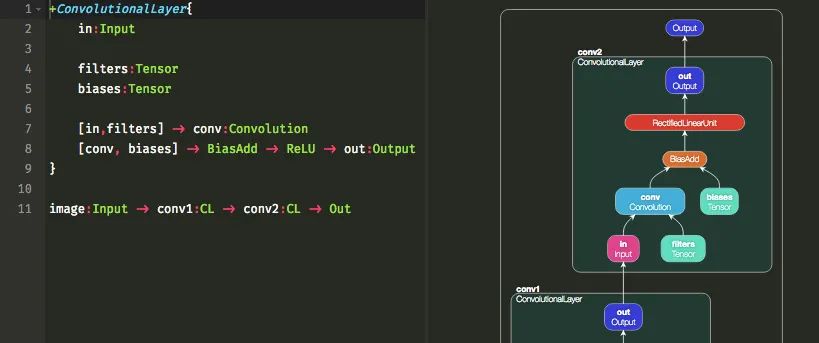
21. Texample
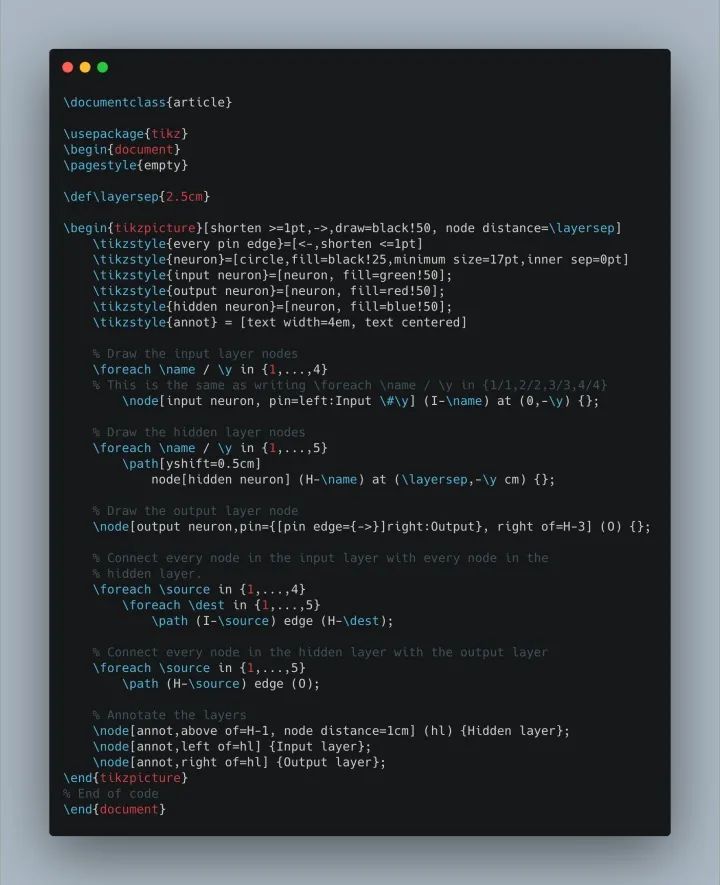
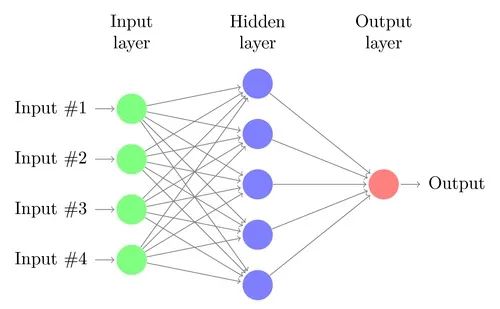
22. Quiver
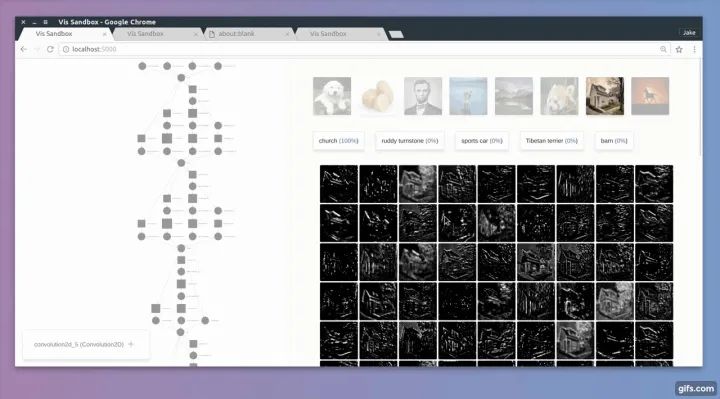
安装方式
pip
pip install quiver_engine
pip install git+git://github.com/keplr-io/quiver.git
使用例子
model = Model(...)
quiver_engine.server.launch(model, classes=['cat','dog'], input_folder='./imgs')
from quiver_engine import server
server.launch(model)
localhost:5000

克隆这个 github 项目:
git clone https://github.com/viscom-ulm/Net2Vis
# 1.进入后端的文件夹内
cd backend
# 2. 安装依赖包
pip3 install -r requirements.txt
# 3. 安装 docker容器
docker build --force-rm -t tf_plus_keras .
# 4. 根据你的系统安装对应的 python 的 cairo 包,比如Debian 的 python-cairosvg
# 5. 开启服务
python server.py
# 1. 进入文件夹
cd net2vis
# 2. 安装 JavaScript 的依赖包
npm install
# 3. 开启应用
npm start
https://datascience.stackexchange.com/questions/12851/how-do-you-visualize-neural-network-architectures https://datascience.stackexchange.com/questions/2670/visualizing-deep-neural-network-training
小结
推荐阅读
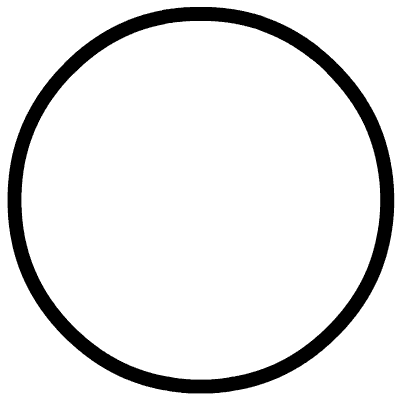
评论