【硬刚大数据】从零到大数据专家面试篇之SparkSQL篇
本文是对《【硬刚大数据之学习路线篇】2021年从零到大数据专家的学习指南(全面升级版)》的面试部分补充。
部分文章:
1.谈谈你对Spark SQL的理解
2.谈谈你对DataSet/DataFrame的理解
1.加载外部数据 以加载json和mysql为例:
val ds = sparkSession.read.json("/路径/people.json")
val ds = sparkSession.read.format("jdbc")
.options(Map("url" -> "jdbc:mysql://ip:port/db",
"driver" -> "com.mysql.jdbc.Driver",
"dbtable" -> "tableName", "user" -> "root", "root" -> "123")).load()
2.RDD转换为DataSet 通过RDD转化创建DataSet,关键在于为RDD指定schema,通常有两种方式(伪代码):
1.定义一个case class,利用反射机制来推断
1) 从HDFS中加载文件为普通RDD
val lineRDD = sparkContext.textFile("hdfs://ip:port/person.txt").map(_.split(" "))
2) 定义case class(相当于表的schema)
case class Person(id:Int, name:String, age:Int)
3) 将RDD和case class关联
val personRDD = lineRDD.map(x => Person(x(0).toInt, x(1), x(2).toInt))
4) 将RDD转换成DataFrame
val ds= personRDD.toDF
2.手动定义一个schema StructType,直接指定在RDD上
val schemaString ="name age"
val schema = StructType(schemaString.split(" ").map(fieldName => StructField(fieldName, StringType, true)))
val rowRdd = peopleRdd.map(p=>Row(p(0),p(1)))
val ds = sparkSession.createDataFrame(rowRdd,schema)
personDS.select(col("name"))
personDS.select(col("name"), col("age"))
personDS.select(col("name"), col("age"), col("salary") + 1000)
personDS.select(personDS("name"), personDS("age"), personDS("salary") + 1000)
personDS.filter(col("age") > 18)
personDS.groupBy("age").count()
personDS.registerTempTable("person")
//查询年龄最大的前两名
val result = sparkSession.sql("select * from person order by age desc limit 2")
//保存结果为json文件。注意:如果不指定存储格式,则默认存储为parquet
result.write.format("json").save("hdfs://ip:port/res2")
3.说说Spark SQL的几种使用方式
1.sparksql-shell交互式查询
2.编程
val spark = SparkSession.builder()
.appName("example").master("local[*]").getOrCreate();
val df = sparkSession.read.format("parquet").load("/路径/parquet文件")
3.Thriftserver
Class.forName("org.apache.hive.jdbc.HiveDriver")
val conn = DriverManager.getConnection("jdbc:hive2://ip:port", "root", "123");
try {
val stat = conn.createStatement()
val res = stat.executeQuery("select * from people limit 1")
while (res.next()) {
println(res.getString("name"))
}
} catch {
case e: Exception => e.printStackTrace()
} finally{
if(conn!=null) conn.close()
}
4.说说Spark SQL 获取Hive数据的方式
$HIVE_HOME/conf/hive-site.xml
,增加如下内容:<property>
<name>hive.metastore.uris</name>
<value>thrift://ip:port</value>
</property>
val spark = SparkSession.builder()
.config(sparkConf).enableHiveSupport().getOrCreate()
5.分别说明UDF、UDAF、Aggregator
UDF UDF是最基础的用户自定义函数,以自定义一个求字符串长度的udf为例:
val udf_str_length = udf{(str:String) => str.length}
spark.udf.register("str_length",udf_str_length)
val ds =sparkSession.read.json("路径/people.json")
ds.createOrReplaceTempView("people")
sparkSession.sql("select str_length(address) from people")UDAF 定义UDAF,需要继承抽象类UserDefinedAggregateFunction,它是弱类型的,下面的aggregator是强类型的。以求平均数为例:
import org.apache.spark.sql.{Row, SparkSession}
import org.apache.spark.sql.expressions.MutableAggregationBuffer
import org.apache.spark.sql.expressions.UserDefinedAggregateFunction
import org.apache.spark.sql.types._
object MyAverage extends UserDefinedAggregateFunction {
// Data types of input arguments of this aggregate function
def inputSchema: StructType = StructType(StructField("inputColumn", LongType) :: Nil)
// Data types of values in the aggregation buffer
def bufferSchema: StructType = {
StructType(StructField("sum", LongType) :: StructField("count", LongType) :: Nil)
}
// The data type of the returned value
def dataType: DataType = DoubleType
// Whether this function always returns the same output on the identical input
def deterministic: Boolean = true
// Initializes the given aggregation buffer. The buffer itself is a `Row` that in addition to
// standard methods like retrieving a value at an index (e.g., get(), getBoolean()), provides
// the opportunity to update its values. Note that arrays and maps inside the buffer are still
// immutable.
def initialize(buffer: MutableAggregationBuffer): Unit = {
buffer(0) = 0L
buffer(1) = 0L
}
// Updates the given aggregation buffer `buffer` with new input data from `input`
def update(buffer: MutableAggregationBuffer, input: Row): Unit = {
if (!input.isNullAt(0)) {
buffer(0) = buffer.getLong(0) + input.getLong(0)
buffer(1) = buffer.getLong(1) + 1
}
}
// Merges two aggregation buffers and stores the updated buffer values back to `buffer1`
def merge(buffer1: MutableAggregationBuffer, buffer2: Row): Unit = {
buffer1(0) = buffer1.getLong(0) + buffer2.getLong(0)
buffer1(1) = buffer1.getLong(1) + buffer2.getLong(1)
}
// Calculates the final result
def evaluate(buffer: Row): Double = buffer.getLong(0).toDouble / buffer.getLong(1)
}
// Register the function to access it
spark.udf.register("myAverage", MyAverage)
val df = spark.read.json("examples/src/main/resources/employees.json")
df.createOrReplaceTempView("employees")
df.show()
val result = spark.sql("SELECT myAverage(salary) as average_salary FROM employees")
result.show()
Aggregator
import org.apache.spark.sql.{Encoder, Encoders, SparkSession}
import org.apache.spark.sql.expressions.Aggregator
case class Employee(name: String, salary: Long)
case class Average(var sum: Long, var count: Long)
object MyAverage extends Aggregator[Employee, Average, Double] {
// A zero value for this aggregation. Should satisfy the property that any b + zero = b
def zero: Average = Average(0L, 0L)
// Combine two values to produce a new value. For performance, the function may modify `buffer`
// and return it instead of constructing a new object
def reduce(buffer: Average, employee: Employee): Average = {
buffer.sum += employee.salary
buffer.count += 1
buffer
}
// Merge two intermediate values
def merge(b1: Average, b2: Average): Average = {
b1.sum += b2.sum
b1.count += b2.count
b1
}
// Transform the output of the reduction
def finish(reduction: Average): Double = reduction.sum.toDouble / reduction.count
// Specifies the Encoder for the intermediate value type
def bufferEncoder: Encoder[Average] = Encoders.product
// Specifies the Encoder for the final output value type
def outputEncoder: Encoder[Double] = Encoders.scalaDouble
}
val ds = spark.read.json("examples/src/main/resources/employees.json").as[Employee]
ds.show()
// Convert the function to a `TypedColumn` and give it a name
val averageSalary = MyAverage.toColumn.name("average_salary")
val result = ds.select(averageSalary)
result.show()
6.对比一下Spark SQL与HiveSQL
7.说说Spark SQL解析查询parquet格式Hive表如何获取分区字段和查询条件
sparkSession.read.format("parquet").load(s"${hive_path}")
,hive_path为Hive分区表在HDFS上的存储路径。1.hive_path为"/spark/dw/test.db/test_partition/dt=20200101"
2.hive_path为"/spark/dw/test.db/test_partition/*"
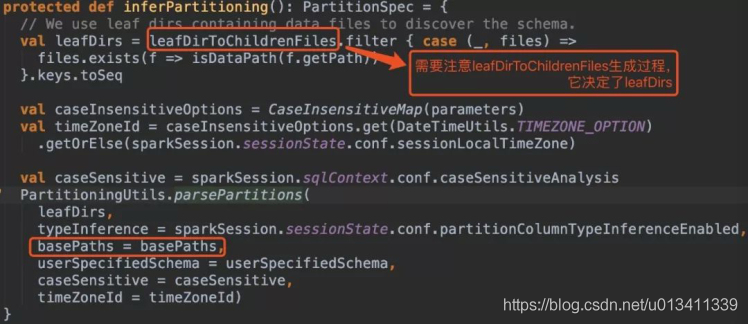
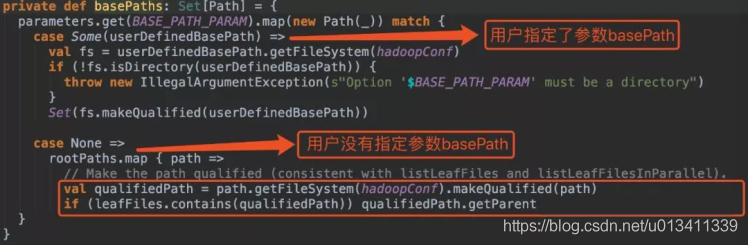
sparksql底层处理后得到的basePaths: Set(new Path(“/spark/dw/test.db/test_partition/dt=20200101”))【伪代码】
leafDirs: Seq(new Path(“/spark/dw/test.db/test_partition/dt=20200101”))【伪代码】
sparksql底层处理后得到的basePaths: Set(new Path(“/spark/dw/test.db/test_partition/dt=20200101”),new Path(“/spark/dw/test.db/test_partition/dt=20200102”))【伪代码】
leafDirs: Seq(new Path(“/spark/dw/test.db/test_partition/dt=20200101”),new Path(“/spark/dw/test.db/test_partition/dt=20200102”))【伪代码】
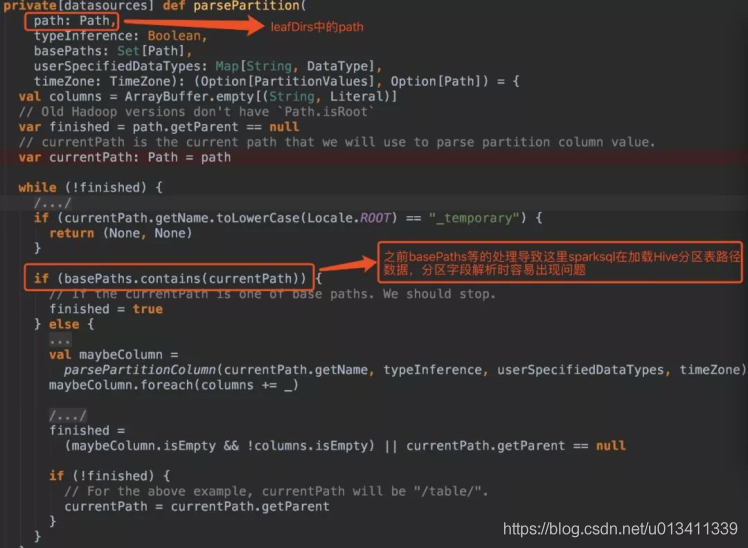
1.在Spark SQL加载Hive表数据路径时,指定参数basePath,如 sparkSession.read.option("basePath","/spark/dw/test.db/test_partition")
2.主要重写basePaths方法和parsePartition方法中的处理逻辑,同时需要修改其他涉及的代码。由于涉及需要改写的代码比较多,可以封装成工具
8.说说你对Spark SQL 小文件问题处理的理解
Caused by: org.apache.spark.SparkException: Job aborted due to stage failure: Total size of serialized results of 478 tasks (2026.0 MB) is bigger than spark.driver.maxResultSize (1024.0 MB)
1.通过repartition或coalesce算子控制最后的DataSet的分区数 注意repartition和coalesce的区别
2.将Hive风格的Coalesce and Repartition Hint 应用到Spark SQL 需要注意这种方式对Spark的版本有要求,建议在Spark2.4.X及以上版本使用,示例:
INSERT ... SELECT /*+ COALESCE(numPartitions) */ ...
INSERT ... SELECT /*+ REPARTITION(numPartitions) */ ...3.小文件定期合并可以定时通过异步的方式针对Hive分区表的每一个分区中的小文件进行合并操作
9.SparkSQL读写Hive metastore Parquet遇到过什么问题吗?
// 第一种方式应用的比较多
1. sparkSession.catalog.refreshTable(s"${dbName.tableName}")
2. sparkSession.catalog.refreshByPath(s"${path}")
10.说说Spark SQL如何选择join策略
1. build table的选择
/* 左表作为build table的条件,join类型需满足:
1. InnerLike:实现目前包括inner join和cross join
2. RightOuter:right outer join
*/
private def canBuildLeft(joinType: JoinType): Boolean = joinType match {
case _: InnerLike | RightOuter => true
case _ => false
}
/* 右表作为build table的条件,join类型需满足(第1种是在业务开发中写的SQL主要适配的):
1. InnerLike、LeftOuter(left outer join)、LeftSemi(left semi join)、LeftAnti(left anti join)
2. ExistenceJoin:only used in the end of optimizer and physical plans, we will not generate SQL for this join type
*/
private def canBuildRight(joinType: JoinType): Boolean = joinType match {
case _: InnerLike | LeftOuter | LeftSemi | LeftAnti | _: ExistenceJoin => true
case _ => false
}
2. 满足什么条件的表才能被广播
private def canBroadcastBySizes(joinType: JoinType, left: LogicalPlan, right: LogicalPlan)
: Boolean = {
val buildLeft = canBuildLeft(joinType) && canBroadcast(left)
val buildRight = canBuildRight(joinType) && canBroadcast(right)
buildLeft || buildRight
}
private def canBroadcast(plan: LogicalPlan): Boolean = {
plan.stats.sizeInBytes >= 0 && plan.stats.sizeInBytes <= conf.autoBroadcastJoinThreshold
}
private def broadcastSideBySizes(joinType: JoinType, left: LogicalPlan, right: LogicalPlan)
: BuildSide = {
val buildLeft = canBuildLeft(joinType) && canBroadcast(left)
val buildRight = canBuildRight(joinType) && canBroadcast(right)
// 最终会调用broadcastSide
broadcastSide(buildLeft, buildRight, left, right)
}
private def canBroadcastByHints(joinType: JoinType, left: LogicalPlan, right: LogicalPlan)
: Boolean = {
val buildLeft = canBuildLeft(joinType) && left.stats.hints.broadcast
val buildRight = canBuildRight(joinType) && right.stats.hints.broadcast
buildLeft || buildRight
}
private def broadcastSideByHints(joinType: JoinType, left: LogicalPlan, right: LogicalPlan)
: BuildSide = {
val buildLeft = canBuildLeft(joinType) && left.stats.hints.broadcast
val buildRight = canBuildRight(joinType) && right.stats.hints.broadcast
// 最终会调用broadcastSide
broadcastSide(buildLeft, buildRight, left, right)
}
private def broadcastSide(
canBuildLeft: Boolean,
canBuildRight: Boolean,
left: LogicalPlan,
right: LogicalPlan): BuildSide = {
def smallerSide =
if (right.stats.sizeInBytes <= left.stats.sizeInBytes) BuildRight else BuildLeft
if (canBuildRight && canBuildLeft) {
// 如果左表和右表都能作为build table,则将根据表的统计信息,确定physical size较小的表作为build table(即使两个表都被指定了hint)
smallerSide
} else if (canBuildRight) {
// 上述条件不满足,优先判断右表是否满足build条件,满足则广播右表。否则,接着判断左表是否满足build条件
BuildRight
} else if (canBuildLeft) {
BuildLeft
} else {
// 如果左表和右表都不能作为build table,则将根据表的统计信息,确定physical size较小的表作为build table。目前主要用于broadcast nested loop join
smallerSide
}
}
3. 是否可构造本地HashMap
// 逻辑计划的单个分区足够小到构建一个hash表
// 注意:要求分区数是固定的。如果分区数是动态的,还需满足其他条件
private def canBuildLocalHashMap(plan: LogicalPlan): Boolean = {
// 逻辑计划的physical size小于spark.sql.autoBroadcastJoinThreshold * spark.sql.shuffle.partitions(默认200)时,即可构造本地HashMap
plan.stats.sizeInBytes < conf.autoBroadcastJoinThreshold * conf.numShufflePartitions
}
case ExtractEquiJoinKeys(joinType, leftKeys, rightKeys, condition, left, right)
if canBroadcastByHints(joinType, left, right) =>
val buildSide = broadcastSideByHints(joinType, left, right)
Seq(joins.BroadcastHashJoinExec(
leftKeys, rightKeys, joinType, buildSide, condition, planLater(left), planLater(right)))
// broadcast hints were not specified, so need to infer it from size and configuration.
case ExtractEquiJoinKeys(joinType, leftKeys, rightKeys, condition, left, right)
if canBroadcastBySizes(joinType, left, right) =>
val buildSide = broadcastSideBySizes(joinType, left, right)
Seq(joins.BroadcastHashJoinExec(
leftKeys, rightKeys, joinType, buildSide, condition, planLater(left), planLater(right)))
spark.sql.join.preferSortMergeJoin为false,即Shuffle Hash Join优先于Sort Merge Join
右表或左表是否能够作为build table
是否能构建本地HashMap
以右表为例,它的逻辑计划大小要远小于左表大小(默认3倍)
case ExtractEquiJoinKeys(joinType, leftKeys, rightKeys, condition, left, right)
if !conf.preferSortMergeJoin && canBuildRight(joinType) && canBuildLocalHashMap(right)
&& muchSmaller(right, left) ||
!RowOrdering.isOrderable(leftKeys) =>
Seq(joins.ShuffledHashJoinExec(
leftKeys, rightKeys, joinType, BuildRight, condition, planLater(left), planLater(right)))
case ExtractEquiJoinKeys(joinType, leftKeys, rightKeys, condition, left, right)
if !conf.preferSortMergeJoin && canBuildLeft(joinType) && uildLocalHashMap(left)
&& muchSmaller(left, right) ||
!RowOrdering.isOrderable(leftKeys) =>
Seq(joins.ShuffledHashJoinExec(
leftKeys, rightKeys, joinType, BuildLeft, condition, planLater(left), planLater(right)))
private def muchSmaller(a: LogicalPlan, b: LogicalPlan): Boolean = {
a.stats.sizeInBytes * 3 <= b.stats.sizeInBytes
}
!RowOrdering.isOrderable(leftKeys)
def isOrderable(exprs: Seq[Expression]): Boolean = exprs.forall(e => isOrderable(e.dataType))
case ExtractEquiJoinKeys(joinType, leftKeys, rightKeys, condition, left, right)
if RowOrdering.isOrderable(leftKeys) =>
joins.SortMergeJoinExec(
leftKeys, rightKeys, joinType, condition, planLater(left), planLater(right)) :: Nil
// Pick BroadcastNestedLoopJoin if one side could be broadcast
case j @ logical.Join(left, right, joinType, condition)
if canBroadcastByHints(joinType, left, right) =>
val buildSide = broadcastSideByHints(joinType, left, right)
joins.BroadcastNestedLoopJoinExec(
planLater(left), planLater(right), buildSide, joinType, condition) :: Nil
case j @ logical.Join(left, right, joinType, condition)
if canBroadcastBySizes(joinType, left, right) =>
val buildSide = broadcastSideBySizes(joinType, left, right)
joins.BroadcastNestedLoopJoinExec(
planLater(left), planLater(right), buildSide, joinType, condition) :: Nil
若join类型InnerLike(关于InnerLike上面已有介绍)对量表直接进行笛卡尔积处理若
上述情况都不满足,最终方案是选择两个表中physical size较小的表进行广播,join策略仍为BNLJ
// Pick CartesianProduct for InnerJoin
case logical.Join(left, right, _: InnerLike, condition) =>
joins.CartesianProductExec(planLater(left), planLater(right), condition) :: Nil
case logical.Join(left, right, joinType, condition) =>
val buildSide = broadcastSide(
left.stats.hints.broadcast, right.stats.hints.broadcast, left, right)
// This join could be very slow or OOM
joins.BroadcastNestedLoopJoinExec(
planLater(left), planLater(right), buildSide, joinType, condition) :: Nil
11.讲讲Spark SQL中Not in Subquery为何低效以及如何规避
// test_partition1 和 test_partition2为Hive外部分区表
select * from test_partition1 t1 where t1.id not in (select id from test_partition2);
== Parsed Logical Plan ==
'Project [*]
+- 'Filter NOT 't1.id IN (list#3 [])
: +- 'Project ['id]
: +- 'UnresolvedRelation `test_partition2`
+- 'SubqueryAlias `t1`
+- 'UnresolvedRelation `test_partition1`
== Analyzed Logical Plan ==
id: string, name: string, dt: string
Project [id#4, name#5, dt#6]
+- Filter NOT id#4 IN (list#3 [])
: +- Project [id#7]
: +- SubqueryAlias `default`.`test_partition2`
: +- HiveTableRelation `default`.`test_partition2`, org.apache.hadoop.hive.ql.io.parquet.serde.ParquetHiveSerDe, [id#7, name#8], [dt#9]
+- SubqueryAlias `t1`
+- SubqueryAlias `default`.`test_partition1`
+- HiveTableRelation `default`.`test_partition1`, org.apache.hadoop.hive.ql.io.parquet.serde.ParquetHiveSerDe, [id#4, name#5], [dt#6]
== Optimized Logical Plan ==
Join LeftAnti, ((id#4 = id#7) || isnull((id#4 = id#7)))
:- HiveTableRelation `default`.`test_partition1`, org.apache.hadoop.hive.ql.io.parquet.serde.ParquetHiveSerDe, [id#4, name#5], [dt#6]
+- Project [id#7]
+- HiveTableRelation `default`.`test_partition2`, org.apache.hadoop.hive.ql.io.parquet.serde.ParquetHiveSerDe, [id#7, name#8], [dt#9]
== Physical Plan ==
BroadcastNestedLoopJoin BuildRight, LeftAnti, ((id#4 = id#7) || isnull((id#4 = id#7)))
:- Scan hive default.test_partition1 [id#4, name#5, dt#6], HiveTableRelation `default`.`test_partition1`, org.apache.hadoop.hive.ql.io.parquet.serde.ParquetHiveSerDe, [id#4, name#5], [dt#6]
+- BroadcastExchange IdentityBroadcastMode
+- Scan hive default.test_partition2 [id#7], HiveTableRelation `default
private def leftExistenceJoin(
// 广播的数据
relation: Broadcast[Array[InternalRow]],
exists: Boolean): RDD[InternalRow] = {
assert(buildSide == BuildRight)
/* streamed对应物理计划中:
Scan hive default.test_partition1 [id#4, name#5, dt#6], HiveTableRelation `default`.`test_partition1`, org.apache.hadoop.hive.ql.io.parquet.serde.ParquetHiveSerDe, [id#4, name#5], [dt#6]
*/
streamed.execute().mapPartitionsInternal { streamedIter =>
val buildRows = relation.value
val joinedRow = new JoinedRow
// 条件是否定义。此处为Some(((id#4 = id#7) || isnull((id#4 = id#7))))
if (condition.isDefined) {
streamedIter.filter(l =>
// exists主要是为了根据joinType来进一步条件判断数据的返回与否,此处joinType为LeftAnti
buildRows.exists(r => boundCondition(joinedRow(l, r))) == exists
)
// else
} else if (buildRows.nonEmpty == exists) {
streamedIter
} else {
Iterator.empty
}
}
}
12.说说SparkSQL中产生笛卡尔积的几种典型场景以及处理策略
join语句中不指定on条件
select * from test_partition1 join test_partition2;
join语句中指定不等值连接
select * from test_partition1 t1 inner join test_partition2 t2 on t1.name <> t2.name;
join语句on中用or指定连接条件
select * from test_partition1 t1 join test_partition2 t2 on t1.id = t2.id or t1.name = t2.name;
join语句on中用||指定连接条件
select * from test_partition1 t1 join test_partition2 t2 on t1.id = t2.id || t1.name = t2.name;
除了上述举的几个典型例子,实际业务开发中产生笛卡尔积的原因多种多样。
--在Spark SQL内部优化过程中针对join策略的选择,最终会通过SortMergeJoin进行处理。
select * from test_partition1 t1 join test_partition2 t2 on t1.id = t2.i
-- Spark SQL内部优化过程中选择了SortMergeJoin方式进行处理
select * from test_partition1 t1 cross join test_partition2 t2 on t1.id = t2.id;
-- test_partition1和test_partition2是Hive分区表
select * from test_partition1 join test_partition2;
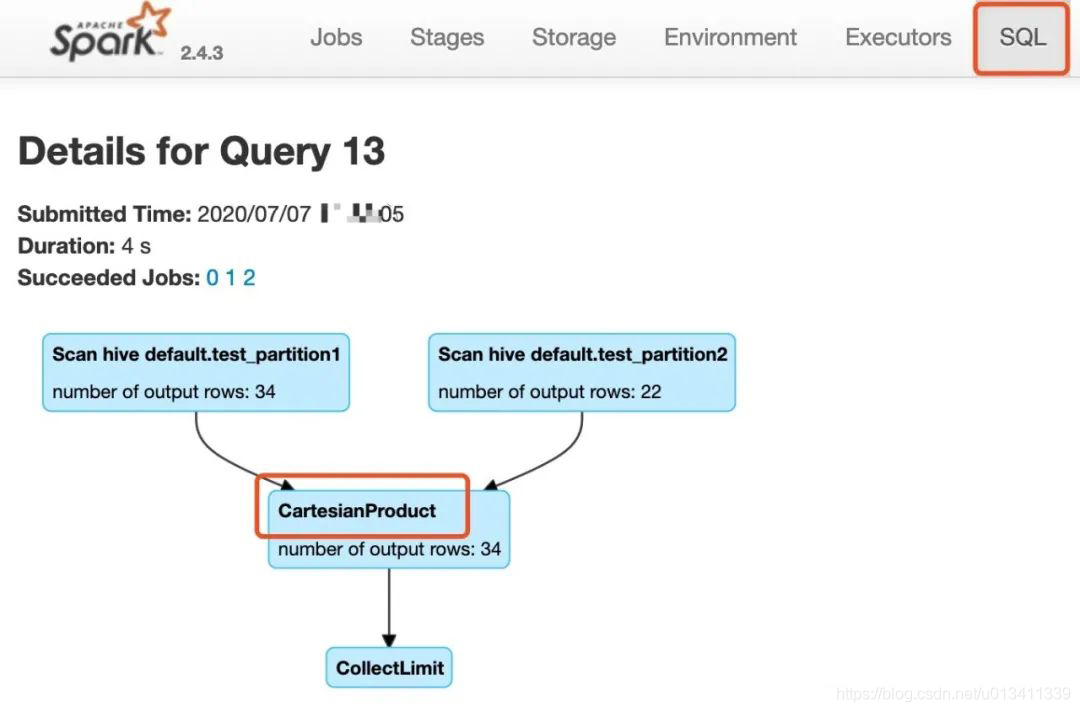
== Parsed Logical Plan ==
'GlobalLimit 1000
+- 'LocalLimit 1000
+- 'Project [*]
+- 'UnresolvedRelation `t`
== Analyzed Logical Plan ==
id: string, name: string, dt: string, id: string, name: string, dt: string
GlobalLimit 1000
+- LocalLimit 1000
+- Project [id#84, name#85, dt#86, id#87, name#88, dt#89]
+- SubqueryAlias `t`
+- Project [id#84, name#85, dt#86, id#87, name#88, dt#89]
+- Join Inner
:- SubqueryAlias `default`.`test_partition1`
: +- HiveTableRelation `default`.`test_partition1`, org.apache.hadoop.hive.ql.io.parquet.serde.ParquetHiveSerDe, [id#84, name#85], [dt#86]
+- SubqueryAlias `default`.`test_partition2`
+- HiveTableRelation `default`.`test_partition2`, org.apache.hadoop.hive.ql.io.parquet.serde.ParquetHiveSerDe, [id#87, name#88], [dt#89]
== Optimized Logical Plan ==
GlobalLimit 1000
+- LocalLimit 1000
+- Join Inner
:- HiveTableRelation `default`.`test_partition1`, org.apache.hadoop.hive.ql.io.parquet.serde.ParquetHiveSerDe, [id#84, name#85], [dt#86]
+- HiveTableRelation `default`.`test_partition2`, org.apache.hadoop.hive.ql.io.parquet.serde.ParquetHiveSerDe, [id#87, name#88], [dt#89]
== Physical Plan ==
CollectLimit 1000
+- CartesianProduct
:- Scan hive default.test_partition1 [id#84, name#85, dt#86], HiveTableRelation `default`.`test_partition1`, org.apache.hadoop.hive.ql.io.parquet.serde.ParquetHiveSerDe, [id#84, name#85], [dt#86]
+- Scan hive default.test_partition2 [id#87, name#88, dt#89], HiveTableRelation `default`.`test_partition2`, org.apache.hadoop.hive.ql.io.parquet.serde.ParquetHiveSerDe, [id#87, name#88], [dt#89]
在利用Spark SQL执行SQL任务时,通过查看SQL的执行图来分析是否产生了笛卡尔积。如果产生笛卡尔积,则将任务杀死,进行任务优化避免笛卡尔积。【不推荐。用户需要到Spark UI上查看执行图,并且需要对Spark UI界面功能等要了解,需要一定的专业性。(注意:这里之所以这样说,是因为Spark SQL是计算引擎,面向的用户角色不同,用户不一定对Spark本身了解透彻,但熟悉SQL。对于做平台的小伙伴儿,想必深有感触)】
分析Spark SQL的逻辑计划和物理计划,通过程序解析计划推断SQL最终是否选择了笛卡尔积执行策略。如果是,及时提示风险。具体可以参考Spark SQL join策略选择的源码:
def apply(plan: LogicalPlan): Seq[SparkPlan] = plan match {
// --- BroadcastHashJoin --------------------------------------------------------------------
// broadcast hints were specified
case ExtractEquiJoinKeys(joinType, leftKeys, rightKeys, condition, left, right)
if canBroadcastByHints(joinType, left, right) =>
val buildSide = broadcastSideByHints(joinType, left, right)
Seq(joins.BroadcastHashJoinExec(
leftKeys, rightKeys, joinType, buildSide, condition, planLater(left), planLater(right)))
// broadcast hints were not specified, so need to infer it from size and configuration.
case ExtractEquiJoinKeys(joinType, leftKeys, rightKeys, condition, left, right)
if canBroadcastBySizes(joinType, left, right) =>
val buildSide = broadcastSideBySizes(joinType, left, right)
Seq(joins.BroadcastHashJoinExec(
leftKeys, rightKeys, joinType, buildSide, condition, planLater(left), planLater(right)))
// --- ShuffledHashJoin ---------------------------------------------------------------------
case ExtractEquiJoinKeys(joinType, leftKeys, rightKeys, condition, left, right)
if !conf.preferSortMergeJoin && canBuildRight(joinType) && canBuildLocalHashMap(right)
&& muchSmaller(right, left) ||
!RowOrdering.isOrderable(leftKeys) =>
Seq(joins.ShuffledHashJoinExec(
leftKeys, rightKeys, joinType, BuildRight, condition, planLater(left), planLater(right)))
case ExtractEquiJoinKeys(joinType, leftKeys, rightKeys, condition, left, right)
if !conf.preferSortMergeJoin && canBuildLeft(joinType) && canBuildLocalHashMap(left)
&& muchSmaller(left, right) ||
!RowOrdering.isOrderable(leftKeys) =>
Seq(joins.ShuffledHashJoinExec(
leftKeys, rightKeys, joinType, BuildLeft, condition, planLater(left), planLater(right)))
// --- SortMergeJoin ------------------------------------------------------------
case ExtractEquiJoinKeys(joinType, leftKeys, rightKeys, condition, left, right)
if RowOrdering.isOrderable(leftKeys) =>
joins.SortMergeJoinExec(
leftKeys, rightKeys, joinType, condition, planLater(left), planLater(right)) :: Nil
// --- Without joining keys ------------------------------------------------------------
// Pick BroadcastNestedLoopJoin if one side could be broadcast
case j @ logical.Join(left, right, joinType, condition)
if canBroadcastByHints(joinType, left, right) =>
val buildSide = broadcastSideByHints(joinType, left, right)
joins.BroadcastNestedLoopJoinExec(
planLater(left), planLater(right), buildSide, joinType, condition) :: Nil
case j @ logical.Join(left, right, joinType, condition)
if canBroadcastBySizes(joinType, left, right) =>
val buildSide = broadcastSideBySizes(joinType, left, right)
joins.BroadcastNestedLoopJoinExec(
planLater(left), planLater(right), buildSide, joinType, condition) :: Nil
// Pick CartesianProduct for InnerJoin
case logical.Join(left, right, _: InnerLike, condition) =>
joins.CartesianProductExec(planLater(left), planLater(right), condition) :: Nil
case logical.Join(left, right, joinType, condition) =>
val buildSide = broadcastSide(
left.stats.hints.broadcast, right.stats.hints.broadcast, left, right)
// This join could be very slow or OOM
joins.BroadcastNestedLoopJoinExec(
planLater(left), planLater(right), buildSide, joinType, condition) :: Nil
// --- Cases where this strategy does not apply ---------------------------------------------
case _ => Nil
}
13.具体讲讲Spark SQL/Hive中的一些实用函数
字符串函数
-- return the concatenation of str1、str2、..., strN
-- SparkSQL
select concat('Spark', 'SQL');
-- return the concatenation of the strings separated by sep
-- Spark-SQL
select concat_ws("-", "Spark", "SQL");
-- encode the first argument using the second argument character set
select encode("HIVE", "UTF-8");
-- decode the first argument using the second argument character set
select decode(encode("HIVE", "UTF-8"), "UTF-8");
-- returns a formatted string from printf-style format strings
select format_string("Spark SQL %d %s", 100, "days");
-- Spark Sql
select initcap("spaRk sql");
-- SPARK SQL
select upper("sPark sql");
-- spark sql
select lower("Spark Sql");
-- 返回4
select length("Hive");
-- vehi
select lpad("hi", 4, "ve");
-- hive
select rpad("hi", 4, "ve");
-- spar
select lpad("spark", 4, "ve");
-- hive
select trim(" hive ");
-- arkSQLS
SELECT ltrim("Sp", "SSparkSQLS") as tmp;
-- 2000
select regexp_extract("1000-2000", "(\\d+)-(\\d+)", 2);
-- r-r
select regexp_replace("100-200", "(\\d+)", "r");
-- aa
select repeat("a", 2);
-- returns the (1-based) index of the first occurrence of substr in str.
-- 6
select instr("SparkSQL", "SQL");
-- 0
select locate("A", "fruit");
select concat(space(2), "A");
-- ["one","two"]
select split("one two", " ");
-- k SQL
select substr("Spark SQL", 5);
-- 从后面开始截取,返回SQL
select substr("Spark SQL", -3);
-- k
select substr("Spark SQL", 5, 1);
-- org.apache。注意:如果参数3为负值,则从右边取值
select substring_index("org.apache.spark", ".", 2);
-- The translate will happen when any character in the string matches the character in the `matchingString`
-- A1B2C3
select translate("AaBbCc", "abc", "123");
get_json_object
-- v2
select get_json_object('{"k1": "v1", "k2": "v2"}', '$.k2');
from_json
select tmp.k from (
select from_json('{"k": "fruit", "v": "apple"}','k STRING, v STRING', map("","")) as tmp
);
to_json
-- 可以把所有字段转化为json字符串,然后表示成value字段
select to_json(struct(*)) AS value;
current_date / current_timestamp 获取当前时间
select current_date;
select current_timestamp;
从日期时间中提取字段/格式化时间 1)year、month、day、dayofmonth、hour、minute、second
-- 20
select day("2020-12-20");
-- 7
select dayofweek("2020-12-12");
/**
* Extracts the week number as an integer from a given date/timestamp/string.
*
* A week is considered to start on a Monday and week 1 is the first week with more than 3 days,
* as defined by ISO 8601
*
* @return An integer, or null if the input was a string that could not be cast to a date
* @group datetime_funcs
* @since 1.5.0
*/
def weekofyear(e: Column): Column = withExpr { WeekOfYear(e.expr) }
-- 50
select weekofyear("2020-12-12");
-- 2020-01-01
select trunc("2020-12-12", "YEAR");
-- 2020-12-01
select trunc("2020-12-12", "MM");
-- 2012-12-12 09:00:00
select date_trunc("HOUR" ,"2012-12-12T09:32:05.359");
-- 2020-12-12
select date_format("2020-12-12 12:12:12", "yyyy-MM-dd");
select unix_timestamp();
-- 1609257600
select unix_timestamp("2020-12-30", "yyyy-MM-dd");
select from_unixtime(1609257600, "yyyy-MM-dd HH:mm:ss");
-- 1609257600
select to_unix_timestamp("2020-12-30", "yyyy-MM-dd");
-- 2020-12-30
select to_date("2020-12-30 12:30:00");
select date("2020-12-30");
select to_timestamp("2020-12-30 12:30:00");
-- 4
select quarter("2020-12-30");
-- 3.94959677
select months_between("1997-02-28 10:30:00", "1996-10-30");
-- 2020-12-28
select add_months("2020-11-28", 1);
-- 2020-12-31
select last_day("2020-12-01");
-- 2020-12-07
select next_day("2020-12-01", "Mon");
-- 2020-12-02
select date_add("2020-12-01", 1);
-- 3
select datediff("2020-12-01", "2020-11-28");
-- to_utc_timestamp(timestamp, timezone) - Given a timestamp like '2017-07-14 02:40:00.0', interprets it as a time in the given time zone, and renders that time as a timestamp in UTC. For example, 'GMT+1' would yield '2017-07-14 01:40:00.0'.
select to_utc_timestamp("2020-12-01", "Asia/Seoul") ;
-- from_utc_timestamp(timestamp, timezone) - Given a timestamp like '2017-07-14 02:40:00.0', interprets it as a time in UTC, and renders that time as a timestamp in the given time zone. For example, 'GMT+1' would yield '2017-07-14 03:40:00.0'.
select from_utc_timestamp("2020-12-01", "Asia/Seoul");
cume_dist
lead(value_expr[,offset[,default]])
lag(value_expr[,offset[,default]])
first_value
last_value
rank
dense_rank
SUM/AVG/MIN/MAX
id time pv
1 2015-04-10 1
1 2015-04-11 3
1 2015-04-12 6
1 2015-04-13 3
1 2015-04-14 2
2 2015-05-15 8
2 2015-05-16 6
SELECT id,
time,
pv,
SUM(pv) OVER(PARTITION BY id ORDER BY time) AS pv1, -- 默认为从起点到当前行
SUM(pv) OVER(PARTITION BY id ORDER BY time ROWS BETWEEN UNBOUNDED PRECEDING AND CURRENT ROW) AS pv2, --从起点到当前行,结果同pv1
SUM(pv) OVER(PARTITION BY id) AS pv3, --分组内所有行
SUM(pv) OVER(PARTITION BY id ORDER BY time ROWS BETWEEN 3 PRECEDING AND CURRENT ROW) AS pv4, --当前行+往前3行
SUM(pv) OVER(PARTITION BY id ORDER BY time ROWS BETWEEN 3 PRECEDING AND 1 FOLLOWING) AS pv5, --当前行+往前3行+往后1行
SUM(pv) OVER(PARTITION BY id ORDER BY time ROWS BETWEEN CURRENT ROW AND UNBOUNDED FOLLOWING) AS pv6 ---当前行+往后所有行
FROM data;
NTILE
ROW_NUMBER

你好,我是王知无,一个大数据领域的硬核原创作者。
做过后端架构、数据中间件、数据平台&架构&、算法工程化。
专注大数据领域实时动态&技术提升&个人成长&职场进阶,欢迎关注。