在不平衡数据上使用AUPRC替代ROC-AUC
数据派THU
共 2895字,需浏览 6分钟
·
2022-06-26 23:45

来源:DeepHub IMBA 本文约2000字,建议阅读7分钟
尽管 ROC-AUC 包含了许多有用的评估信息,但它并不是一个万能的衡量标准。
预备知识——计算曲线
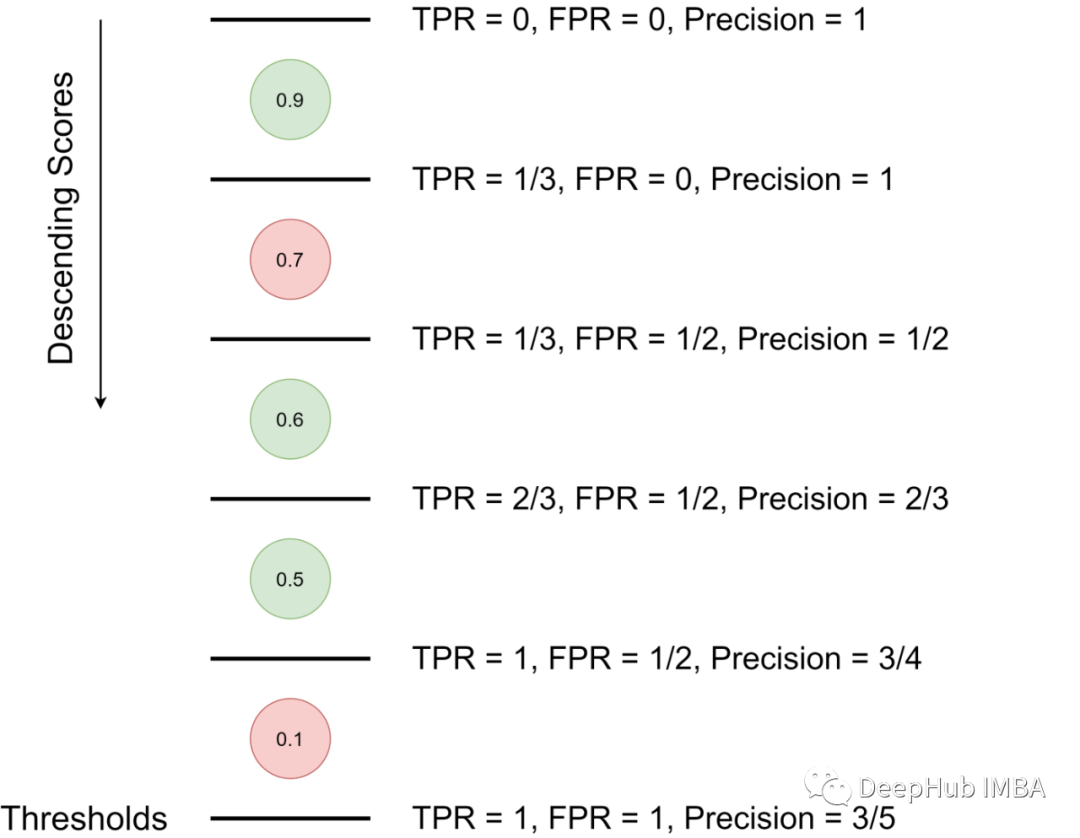
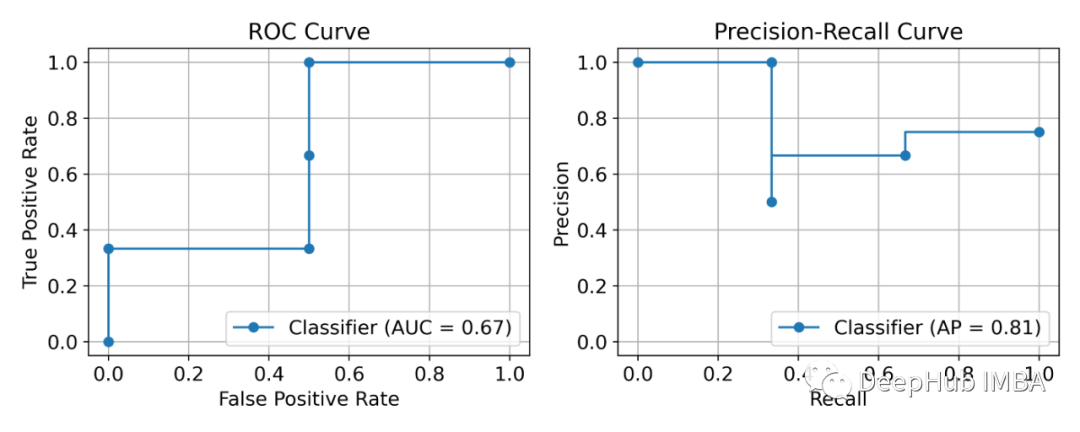
比较 ROC-AUC 和 AUPRC
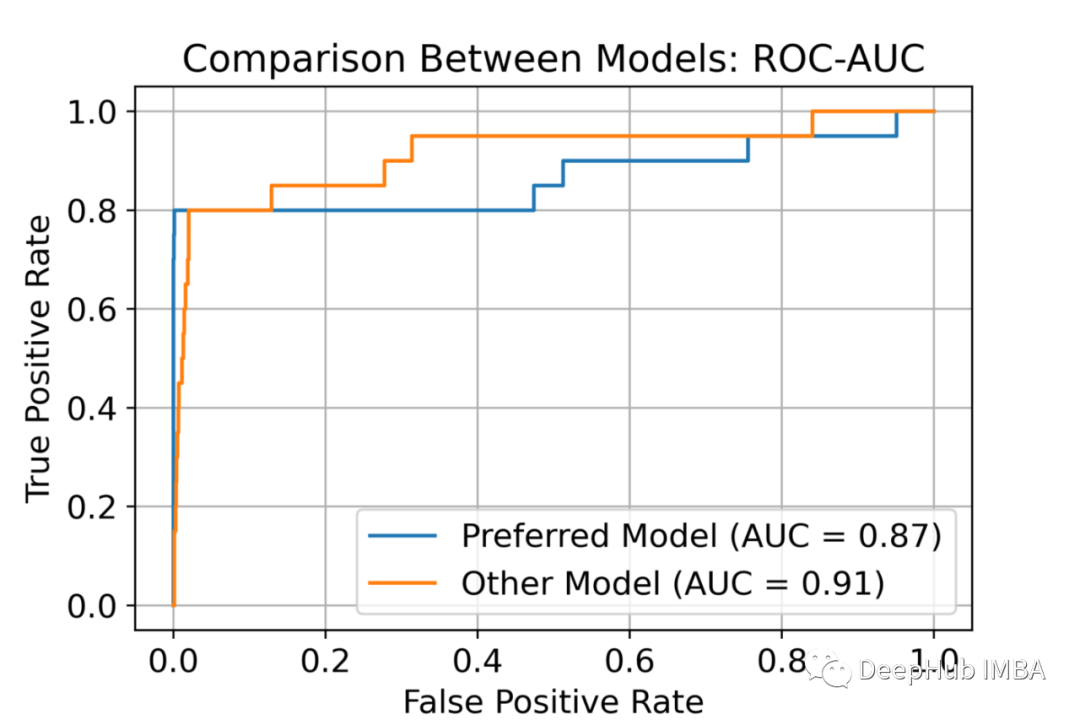
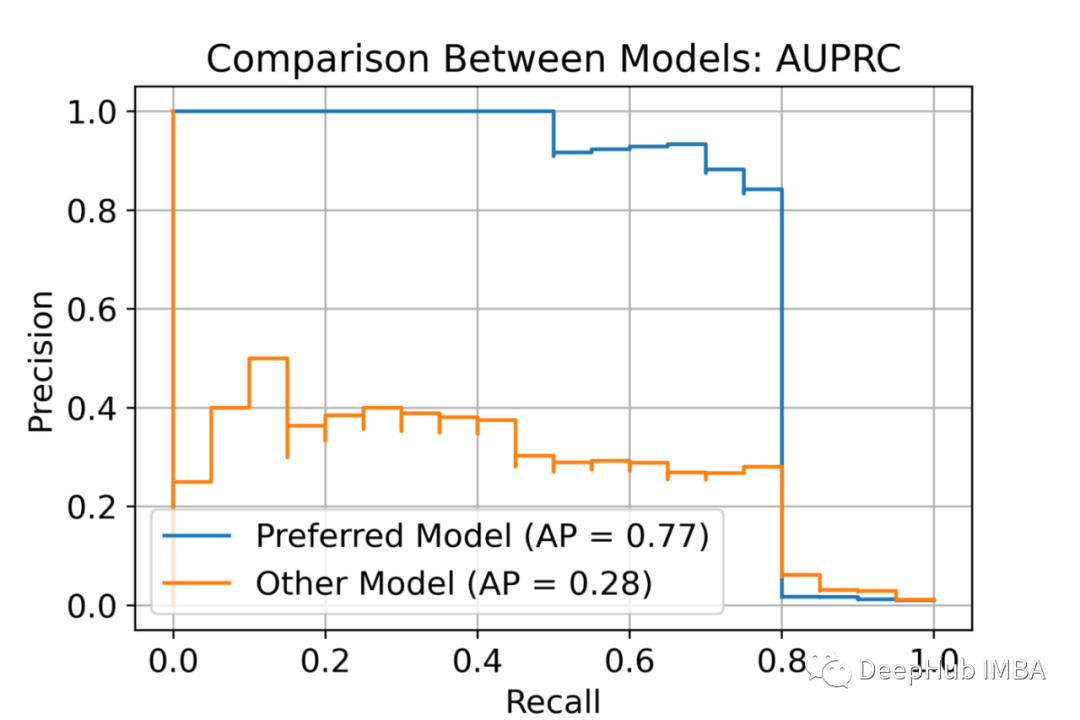
20个正例 2000个负例
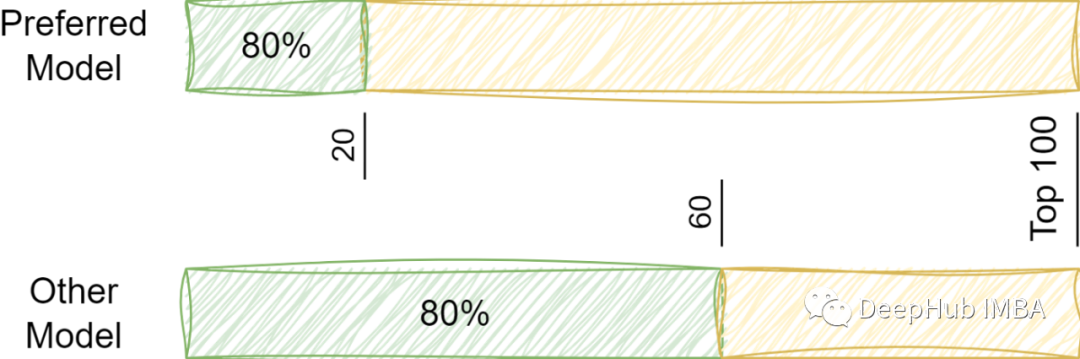
解释差异

总结
编辑:黄继彦
评论
AndroidLame在android上使用lame
lamelame官网 本项目主要方便大家在android上使用lame。Android使用AudioRecord录音时不支持mp3格式,需要先录制为raw格式,然后再使用lame转换成mp3引用方式M
AndroidLame在android上使用lame
0
AndroidLame在android上使用lame
lamelame 官网 本项目主要方便大家在android上使用lame。Android使用Audi
AndroidLame在android上使用lame
0