使用Pytorch实现风格迁移(Neural-Transfer)
共 9642字,需浏览 20分钟
·
2021-08-26 22:10
# 前言
本文主要向大家分享一个小编刚刚学习的神经网络应用的实例:风格迁移(Neural-Transfer)。这是一个由 Leon A. Gatys,Alexander S. Ecker和Matthias Bethge提出的算法。通过这个算法,我们可以用一种新的风格对指定图片进行重构,更通俗一点即:风格图片+内容图片=输出图片,即:
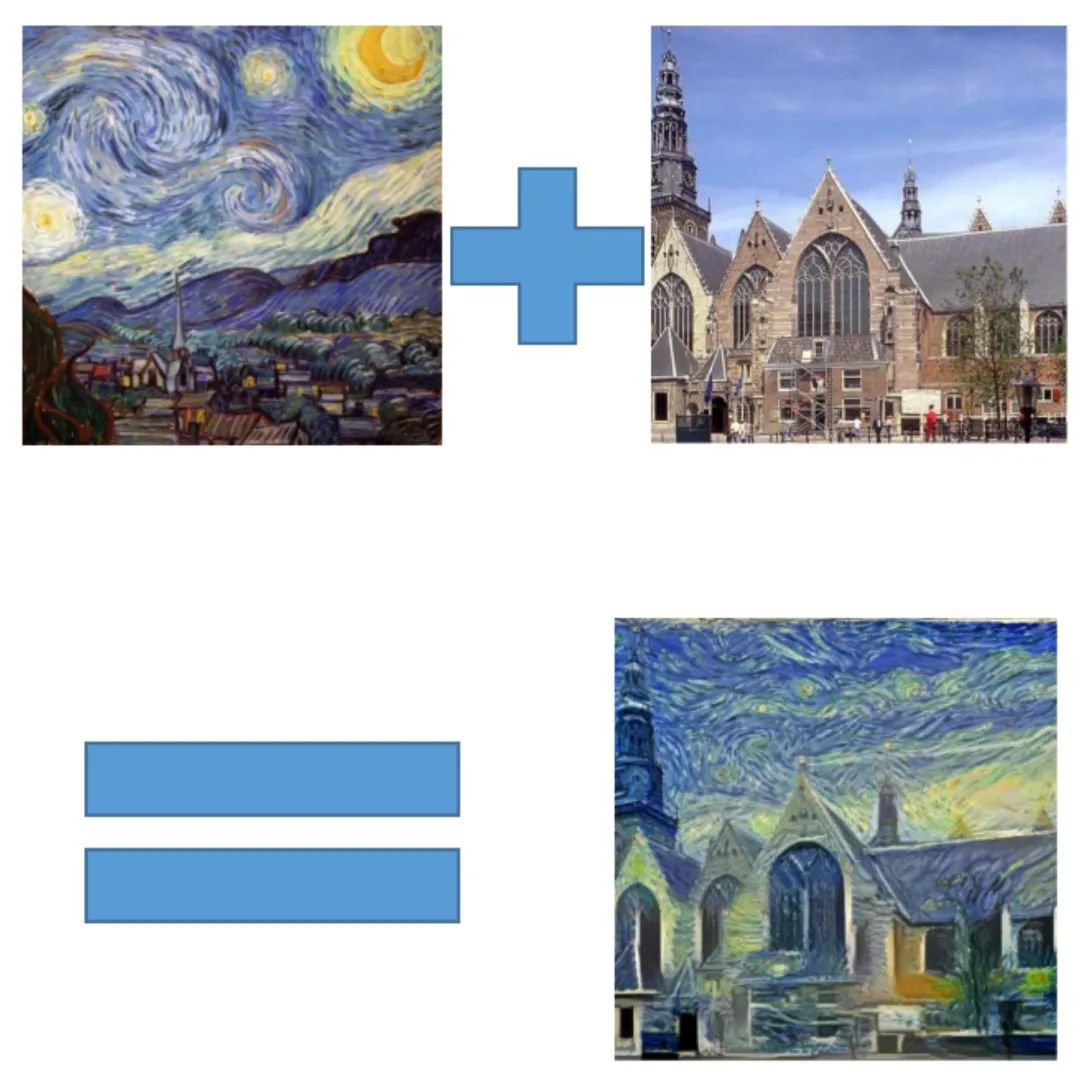
如上图所示,神经风格迁移可以将内容图像的内容、风格图像的风格混合在一起,使得输出的图片看起来像内容图像,但采用了风格图像的风格。这就生成了一个带有《星空》风格的风景图,是不是很有趣呢?下面让我们一起来看看它是怎么实现的吧!
01
基本原理
原理很简单:我们定义了两个距离,一个为内容D_c和一个用于样式D_s。D_c 测量两个图像之间的内容有多大不同,而 D_s衡量两个图像之间风格的差异。然后,我们生成第三张图片,并利用D_c和D_s来优化这张图片,使其与内容图片的内容差别和风格图片的风格差别最小化。当然,我们的第一步是利用卷积神经网络提取出图片的特征,如图所示:
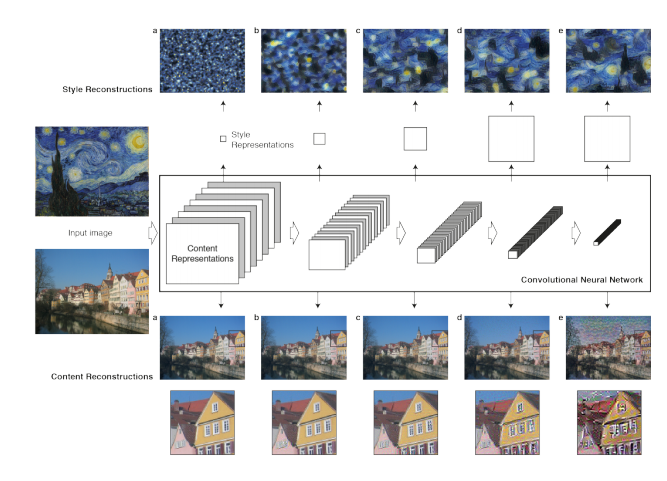
02
读入图片
现在我们导入content和style的原图,我们需要使用PIL中的Image来读取内存中的图片(PS:opencv也可以,但PIL的效果更好一点),再用torchvision中的transforms将读入图片转化为tensor以便之后的操作。另外,我们还需要定义一个用于输出图像的函数,以便输出最终所得到的图片,该函数会将tensor转化为图像。
代码如下:
# load_img模块
import PIL.Image as Image
import torch
import torchvision.transforms as transforms
img_size = 512 if torch.cuda.is_available() else 128#根据设备选择改变后项数大小
def load_img(img_path):#图像读入
img = Image.open(img_path).convert('RGB')#将图像读入并转换成RGB形式
img = img.resize(img_size, img_size)#调整读入图像像素大小
img = transforms.ToTensor()(img)#将图像转化为tensor
img = img.unsqueeze(0)#在0维上增加一个维度
return img
def show_img(img):#图像输出
img = img.squeeze(0)#将多余的0维通道删去
img = transforms.ToPILImage()(img)#将tensor转化为图像
img.show()
03
损失函数
下一步是定义我们的损失函数,为了实现神经风格迁移,我们需要定义一个关于生成图像(Generated image)G的损失函数,用于评价生成图像的好坏。通过最小化损失函数的方式,来生成所要的图像。损失函数需要分成两部分,一个是内容损失函数,它是关于生成图像G与内容图像C的函数,用于衡量生成图像与内容图像在内容上有多相似;一个是风格损失函数,关于生成图像G与风格图像S的函数,用于衡量生成图像与风格图像在风格上的相似度。最后需要用两个超参数α和β来确定两个函数之间的权重,我们的总损失是它们两个的加权和,即

那么我们的关键就在于先单独求出内容损失和风格损失,计算它们的损失也很简单,首先我们看一下内容损失,我们使用最简单的均方误差:
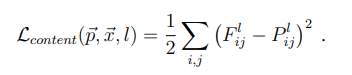
上式是内容损失函数的定义。其中l代表第l层的特征表示,p是原始内容图片特征图,x是生成图片特征图。公式的含义就是对于每一层,原始图片的特征图(feature map)和生成图片的特征图的一一对应做差值平方和。
然后我们需要对损失梯度下降求导来优化参数
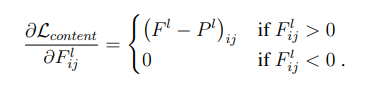
对于风格损失我们还需要引入Gram矩阵来帮助我们表示图像的风格特征,我们读入图像卷积层的输出形状为C × H × W ,C是卷积核的通道数,每个卷积核学习图像不同特征,每个卷积核输出H × W 代表这张图像的一个feature map,读入RGB图像的三色通道相当于三个feature map,我们用Gram矩阵来计算feature map间的相似性,得到图像的风格特征。
关于Gram矩阵
由于计算风格损失需要Gram矩阵,所以我们先来了解一下它吧。
Gram矩阵的定义:
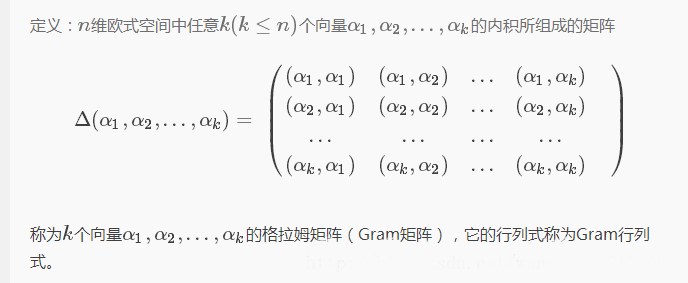
Gram矩阵计算公式:
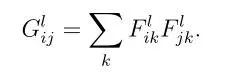
F表示生成图像的feature map。上面式子的含义:第l层的Gram矩阵第i行,第j列的数值等于把生成图像在第l层的第i个feature map与第j个feature map分别拉成一维后相乘求和,即Gram矩阵中的每个值都是每个通道 i 的feature map与每个通道 j 的feature map的内积,由于内积可以判断两个向量之间的夹角和方向关系(如:若a·b>0,则二者方向相同,夹角在0°到90°之间),所以Gram矩阵中的值可以反映出两个feature map之间的某种关系。
Gram矩阵可以看是feature之间的偏心协方差矩阵,feature map中的每个数字都来自于一个特定滤波器在特定位置的卷积,因此每个数字代表一个特征的强度,而Gram计算的实际上是两两特征之间的相关性。Gram的对角线元素提供了不同特征图各自的信息,而其余元素提供了不同特征图之间的相关信息,因此,Gram有助于把握整个图像的大体风格。有了表示风格的Gram矩阵,要度量两个图像风格的差异,只需比较他们Gram矩阵的差异即可。
然后我们就可以利用Gram矩阵提取的风格特征计算损失了,这里我们仍然使用均方误差,并进行归一化操作。
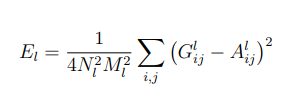
上面是第l层的风格损失函数,N是指生成图feature map数量,M是图片宽乘高,G是生成图像的Gram矩阵,A是风格图像的Gram矩阵。
然后是梯度下降:

最终的风格损失函数为每一层的风格损失加权求和可得。式中,a是指风格图像,x是指生成图像,w是指权重:
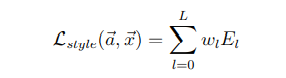
下面我们看看如何用代码实现它:
# loss板块
import torch.nn as nn
import torch
class Content_Loss(nn.Module):#内容损失
def __init__(self, target, weight):
super(Content_Loss, self).__init__()#继承父类的初始化
self.weight = weight
self.target = target.detach() * self.weight
# 必须要用detach来分离出target,这时候target不再是一个Variable,这是为了动态计算梯度,否则forward会出错,不能向前传播
self.criterion = nn.MSELoss()#利用均方误差计算损失
def forward(self, input):#向前计算损失
self.loss = self.criterion(input * self.weight, self.target)
out = input.clone()
return out
def backward(self, retain_graph=True):#反向求导
self.loss.backward(retain_graph=retain_graph)
return self.loss
class Gram(nn.Module):#定义Gram矩阵
def __init__(self):
super(Gram, self).__init__()
def forward(self, input):#向前计算Gram矩阵
a, b, c, d = input.size()#a为批量大小,b为feature map的数量,c*d为feature map的大小
feature = input.view(a * b, c * d)
gram = torch.mm(feature, feature.t())
gram /= (a * b * c * d)
return gram
class Style_Loss(nn.Module):#风格损失
def __init__(self, target, weight):
super(Style_Loss, self).__init__()
self.weight = weight
self.target = target.detach() * self.weight
self.gram = Gram()
self.criterion = nn.MSELoss()
def forward(self, input):
G = self.gram(input) * self.weight
self.loss = self.criterion(G, self.target)
out = input.clone()
return out
def backward(self, retain_graph=True):
self.loss.backward(retain_graph=retain_graph)
return self.loss
04
模型构建
接下来就该构建我们的模型啦!虽然我们用的是已经预训练好的vgg框架,但我们还需要对它做一些“改造”:把我们之前构造好的损失函数加进去。毕竟“白嫖”也是有限度的嘛!话不多说,上代码!
# build_model模块
import torch.nn as nn
import torch
import torchvision.models as models
import loss # 指的是上文中已经写好的loss模块
device = torch.device("cuda" if torch.cuda.is_available() else "cpu")#选择运行设备,如果你的电脑有gpu就在gpu上运行,否则在cpu上运行
vgg = models.vgg19(pretrained=True).features.to(device)#这里我们使用预训练好的vgg19模型
'''所需的深度层来计算风格/内容损失:'''
content_layers_default = ['conv_4']
style_layers_default = ['conv_1', 'conv_2', 'conv_3', 'conv_4', 'conv_5']
def get_style_model_and_loss(style_img,
content_img,
cnn=vgg,
style_weight=1000,
content_weight=1,
content_layers=content_layers_default,
style_layers=style_layers_default):
content_loss_list = [] #内容损失
style_loss_list = [] #风格损失
model = nn.Sequential() #创建一个model,按顺序放入layer
model = model.to(device)
gram = loss.Gram().to(device)
'''把vgg19中的layer、content_loss以及style_loss按顺序加入到model中:'''
i = 1
for layer in cnn:
if isinstance(layer, nn.Conv2d):
name = 'conv_' + str(i)
model.add_module(name, layer)
if name in content_layers_default:
target = model(content_img)
content_loss = loss.Content_Loss(target, content_weight)
model.add_module('content_loss_' + str(i), content_loss)
content_loss_list.append(content_loss)
if name in style_layers_default:
target = model(style_img)
target = gram(target)
style_loss = loss.Style_Loss(target, style_weight)
model.add_module('style_loss_' + str(i), style_loss)
style_loss_list.append(style_loss)
i += 1
if isinstance(layer, nn.MaxPool2d):
name = 'pool_' + str(i)
model.add_module(name, layer)
if isinstance(layer, nn.ReLU):
name = 'relu' + str(i)
model.add_module(name, layer)
return model, style_loss_list, content_loss_list
05
执行
一切工作准备就绪,我们就可以开始定义我们的run_code模块了,经历过这么多操作终于走到了最后一步,是不是有点小激动呢?我们的执行模块也很简单,先定义一个LBFGS优化器,然后就可以开始我们一次一次的训练了。这里我们定义每训练50次输出一次我们的损失来评估学习效果。
# run_code模块
import torch.nn as nn
import torch.optim as optim
from build_model import get_style_model_and_loss
def get_input_param_optimier(input_img):
"""input_img is a Variable"""
input_param = nn.Parameter(input_img.data)#获取参数
optimizer = optim.LBFGS([input_param])#用LBFGS优化参数
return input_param, optimizer
def run_style_transfer(content_img, style_img, input_img, num_epoches=300):
print('Building the style transfer model..')
model, style_loss_list, content_loss_list = get_style_model_and_loss(
style_img, content_img)
input_param, optimizer = get_input_param_optimier(input_img)
print('Opimizing...')
epoch = [0]
while epoch[0] < num_epoches:#每隔50次输出一次loss
def closure():
input_param.data.clamp_(0, 1)#修正输入图像的值
model(input_param)
style_score = 0
content_score = 0
optimizer.zero_grad()
for sl in style_loss_list:
style_score += sl.backward()
for cl in content_loss_list:
content_score += cl.backward()
epoch[0] += 1
if epoch[0] % 50 == 0:
print('run {}'.format(epoch))
print('Style Loss: {:.4f} Content Loss: {:.4f}'.format(
style_score.item(), content_score.item()))
print()
return style_score + content_score
optimizer.step(closure)
input_param.data.clamp_(0, 1)#再次修正
return input_param.data
06
运行
最最最最最激动人心的时刻就要到了!敲了这么多代码,现在我们就可以开始验证我们的成果了!现在我们只需要挑选一张中意的图片,再为它寻找一个你想要的风格图片,只需短短几分钟(甚至几十秒),你,就创造出了属于自己的艺术画作(其实一般)!!!
# start模块
from torch.autograd import Variable
from torchvision import transforms
import torch
from run_code import run_style_transfer
from load_img import load_img, show_img
device = torch.device("cuda" if torch.cuda.is_available() else "cpu")
style_img = load_img('./picture/style1.png')#风格图片地址
style_img = Variable(style_img).to(device)
content_img = load_img('./picture/content5.png')#内容图片地址
content_img = Variable(content_img).to(device)
input_img = content_img.clone()
out = run_style_transfer(content_img, style_img, input_img, num_epoches=200)#进行200次训练
save_pic = transforms.ToPILImage()(out.cpu().squeeze(0))
save_pic.save('./picture/result4.png')#选择你要保存的地址
save_pic.show()
下面我们做几组演示:
输入:
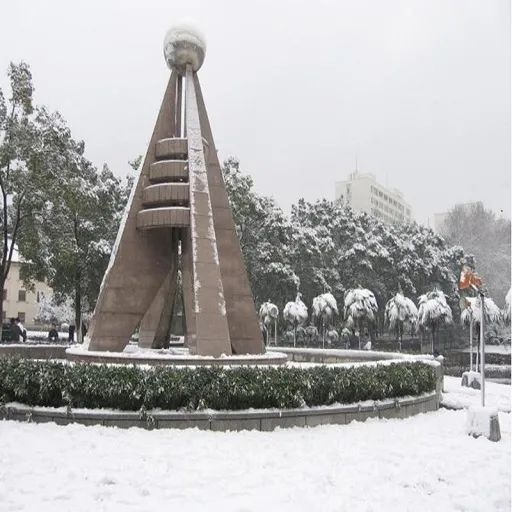
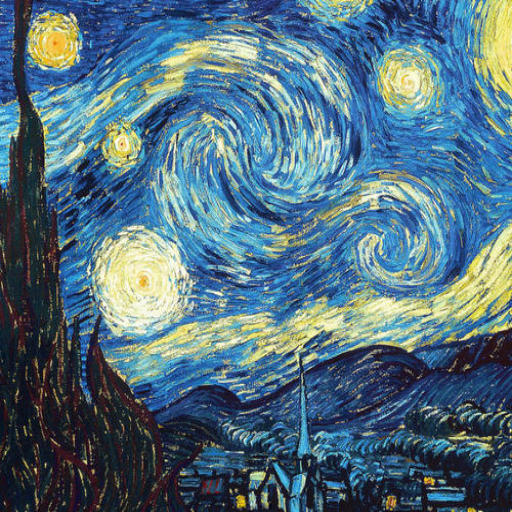
输出:
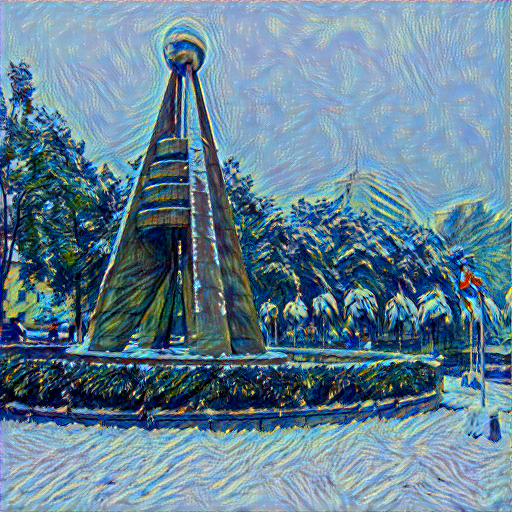
输入:
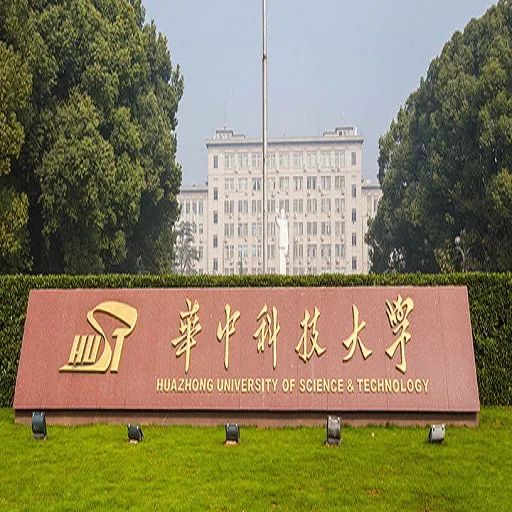
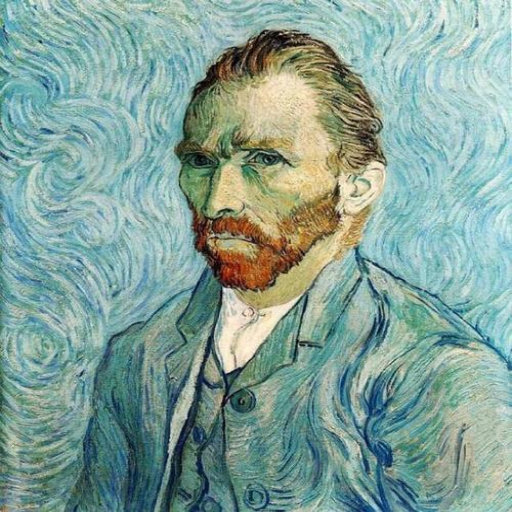
输出:
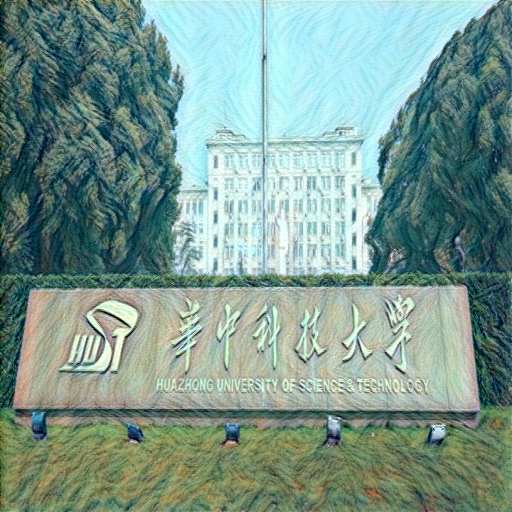
最后我们试一试人像:
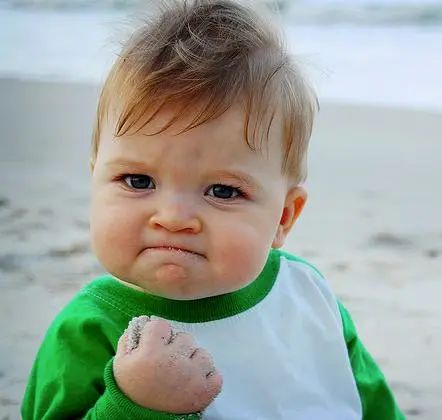
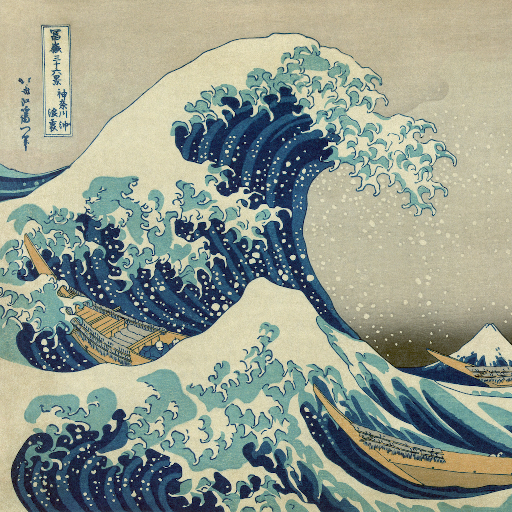
输出:
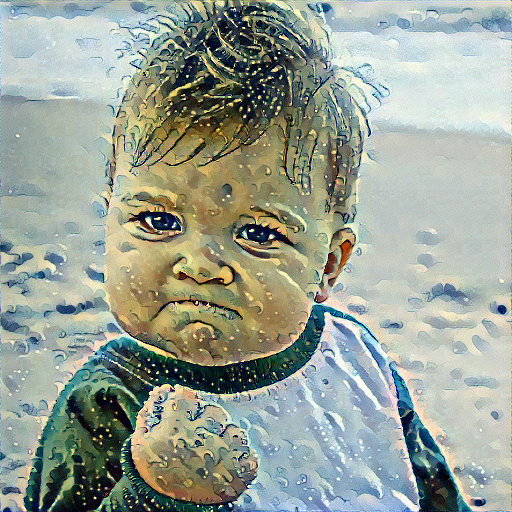
下面是其中一组loss的输出:
Opimizing...
run [50]
Style Loss: 0.7299 Content Loss: 3.7480
run [100]
Style Loss: 0.3702 Content Loss: 3.3560
run [150]
Style Loss: 0.2747 Content Loss: 3.1843
run [200]
Style Loss: 0.2058 Content Loss: 3.0981
我们可以看出内容损失还是挺大的,损失的大小不仅跟我们的模型有关,我们选择的图片也会对损失有很大的影响,不得不说有的图片特征确实难以捕获,小编曾经还有过训练1000次仍然有40多损失的惨痛经历,这种情况就不是单纯增加训练次数就能解决的了,我们需要更强大的模型去捕获信息,大家也可以自己试试改进一下模型,也许会有意外的收获呦!
reference
1] Gatys L A, Ecker A S, Bethge M. A neural algorithm of artistic style[J]. arXiv preprint arXiv:1508.06576, 2015.
2] https://pytorch.org/tutorials/advanced/neural_style_tutorial.html#importing-packages-and-selecting-a-device
1
END
1
文案&排版:王心怡(华中科技大学管理学院本科一年级)
潘云飞(华中科技大学管理学院本科一年级)
指导老师:秦虎老师(华中科技大学管理学院)
审稿:张宇(华中科技大学管理学院本科二年级)
如对文中内容有疑问,欢迎交流。PS:部分资料来自网络。
如有需求,可以联系:
秦虎老师(华中科技大学管理学院:tigerqin1980@qq.com)
王心怡(华中科技大学管理学院本科一年级:3348619379@qq.com)
潘云飞(华中科技大学管理学院本科一年级:1401914932@qq.com)
张宇(华中科技大学管理学院本科二年级:8611452@qq.com)