Kafka Consumer Rebalance详解
共 18805字,需浏览 38分钟
·
2022-11-18 17:55
文章目录
- Kafka版本
- rebalance
- rebalance策略
- rebalance generation
- rebalance协议
- rebalance流程
- rebalance监听器
1. Kafka版本
kafka版本1.1.1,可能绝大部分也适用于kafka 0.10.x及以上版本。
2. rebalance
-
ConsumerGroup(消费组)
里的Consumer(消费者)
共同读取topic(主题)
的partition(分区)
,一个新的Consumer(消费者)
加入ConsumerGroup(消费组)
时,读取的是原本由其他Consumer(消费者)
读取的消息。当一个Consumer(消费者)
被关闭或发生奔溃时,它就离开ConsumerGroup(消费组)
,原本由它读取的分区将有ConsumerGroup(消费组)
的其他Consumer(消费者)
来读取。在topic
发生变化时(比如添加了新的分区),会发生Partition
重分配,Partition
的所有权从一个Consumer(消费者)
转移到另一个Consumer(消费者)
的行为被称为rebalance(再均衡)
。rebalance(再均衡)
本质上是一种协议,规定了ConsumerGroup(消费组)
中所有Consumer(消费者)
如何达成一致来消费topic(主题)
下的partition(分区)
-
rebalance(再均衡)
为ConsumerGroup(消费组)
带来了高可用性和伸缩性(可以安全的添加或移除消费者),在rebalance(再均衡)
期间,Consumer(消费者)
无法读取消息,造成整个Consumer(消费者)
一段时间的不可用 -
Consumer(消费者)
通过向GroupCoordinator(群组协调器)
(不同的ConsumerGroup(消费组)
可以有不同的)发送心跳来维持它们与群组的从属关系以及它们对分区的所有权关系。Consumer(消费者)
会在轮询消息或者提交偏移量时发送心跳(kafka0.10.1
之前的版本),在kafka0.10.1
版本里,心跳线程是独立的 -
分配分区的过程
-
Consumer(消费者)
加入ConsumerGroup(消费组)
时,会向GroupCoordinator(群组协调器)
发送一个JoinGroup
请求,第一个加入群组的Consumer(消费者)
将会成为群主,群主从GroupCoordinator(群组协调器)
获得ConsumerGroup(消费组)
的成员列表(此列表包含所有最新正常发送心跳的活跃的Consumer(消费者)
),并负责给每一个Consumer(消费者)
分配分区(PartitionAssignor
的实现类来决定哪个分区被分配给哪个Consumer(消费者)
) -
群主把分配情况列表发送给
GroupCoordinator(群组协调器)
,GroupCoordinator(群组协调器)
再把这些信息发送给ConsumerGroup(消费组)
里所有的Consumer(消费者)
。每个Consumer(消费者)
只能看到自己的分配信息,只有群主知道ConsumerGroup(消费组)
里所有消费者的分配信息。
rebalance(再均衡)
触发条件
-
ConsumerGroup(消费组)
里的Consumer(消费者)
发生变更(主动加入、主动离开、崩溃),崩溃不一定就是指 consumer进程"挂掉"、 consumer进程所在的机器宕机、长时间GC、网络延迟,当 consumer无法在指定的时间内完成消息的处理,那么coordinator就认为该 consumer已经崩溃,从而引发新一轮 rebalance -
订阅
topic(主题)
的数量发生变更(比如使用正则表达式的方式订阅),当匹配正则表达式的新topic被创建时则会触发 rebalance。 -
订阅
topic(主题)
的partition(分区)
数量发生变更,比如使用命令行脚本增加了订阅 topic 的分区数
3. rebalance策略
-
分配策略:决定订阅topic的每个分区会被分配给哪个consumer。默认提供了 3 种分配策略,分别是 range 策略、round-robin策略和 sticky策略,可以通过
partition.assignment.strategy
参数指定。kafka1.1.x
默认使用range策略 -
range策略:将单个 topic 的所有分区按照顺序排列,然后把这些分区划分成固定大小的分区段并依次分配给每个 consumer。假设有ConsumerA和ConsumerB分别处理三个分区的数据,当ConsumerC加入时,触发rebalance后,ConsumerA、ConsumerB、ConsumerC每个都处理2个分区的数据。
-
round-robin 策略:把所有 topic 的所有分区顺序摆开,然后轮询式地分配给各个 consumer
-
sticky策略(0.11.0.0后引入):有效地避免了上述两种策略完全无视历史分配方案的缺陷。采用了"有黏性"的策略对所有 consumer 实例进行分配,可以规避极端情况下的数据倾斜并且在两次 rebalance间最大限度地维持了之前的分配方案
4. rebalance generation
-
rebalance generation 用于标识某次 rebalance,在 consumer中它是一个整数,通常从 0开始
-
consumer generation主要是防止无效 offset提交。比如上一届的 consumer成员由于某些原因延迟提交了 offset(已经被踢出group),但 rebalance 之后该 group 产生了新一届的 group成员,而延迟的offset提交携带的是旧的 generation信息,因此这次提交会被 consumer group拒绝,使用 consumer时经常碰到的 ILLEGAL_GENERATION异常就是这个原因导致的
-
每个 group进行 rebalance之后, generation号都会加 1,表示 group进入了 一个新的版本
5. rebalance协议
-
rebalance本质是一组协议
-
JoinGroup
: consumer请求加入组 -
SyncGroup
:group leader把分配方案同步更新到组内所有成员 -
Heartbeat
:consumer定期向coordinator发送心跳表示自己存活 -
LeaveGroup
:consumer主动通知coordinator该consumer即将离组 -
DescribeGroup
:查看组的所有信息,包括成员信息、协议信息、分配方案以及订阅信息。该请求类型主要供管理员使用 。coordinator不使用该请求执行 rebalance
在 rebalance过程中,coordinator主要处理 consumer发过来的JoinGroup
和 SyncGroup
请求
当 consumer主动离组时会发送LeaveGroup
请求给 coordinator
在成功 rebalance之后,组内所有 consumer都需要定期地向 coordinator发送Heartbeat
请求。每个 consumer 也是根据Heartbeat
请求的响应中是否包含 REBALANCE_IN_PROGRESS 来判断当前group是否开启了新一轮 rebalance
6. rebalance流程
- 第一步确认coordinator所在的broker,并建立socket连接
-
计算Math.abs(groupId.hashcode)%offset.topic.num.partitions(默认50),假设是10
-
查找
__consumer_offsets分区10的leader副本所在的broker,该broker即为这个consumer group的coordinator
- 第二步执行rebalance,rebalance分为两步
-
加入组
-
组内所有consumer向coordinator发送
JoinGroup
请求 - coordinator选择一个consumer担任组的leader,并把所有成员信息以及订阅信息发送给leader
-
组内所有consumer向coordinator发送
-
同步更新方案
- leader根据rebalance的分配策略为 group中所有成员制定分配方案,决定每个 consumer都负责哪些 topic 的哪些分区。
-
分配完成后,leader通过
SyncGroup
请求将分配方案发送给coordinator。组内所有的consumer成员也会发送SyncGroup
给coordinator -
coordinator把每个consumer的方案抽取出来作为
SyncGroup
请求的response返回给各自的consumer
-
为什么consumer group的分配方案在consumer端执行?
- 这样做可以有更好的灵活性。比如同一个机架上的分区数据被分配给相同机架上的 consumer减少网络传输的开销。即使以后分区策略发生了变更,也只需要重启 consumer 应用即可,不必重启 Kafka服务器
加入组流程
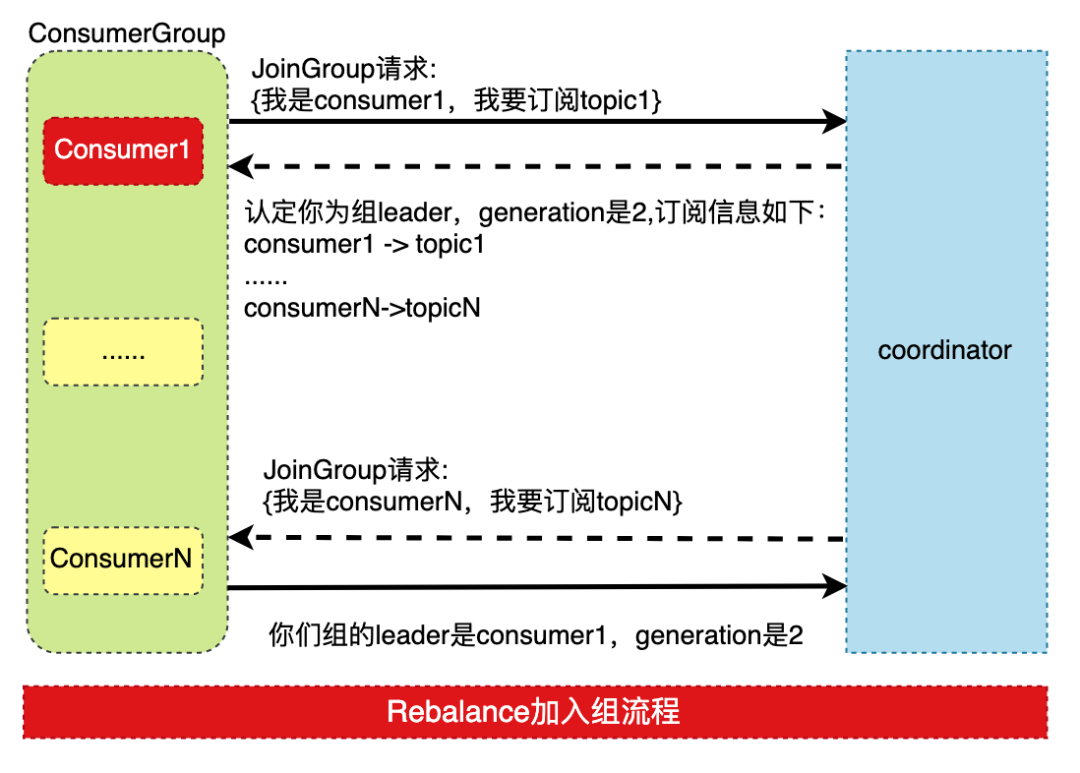
- 同步分配方案
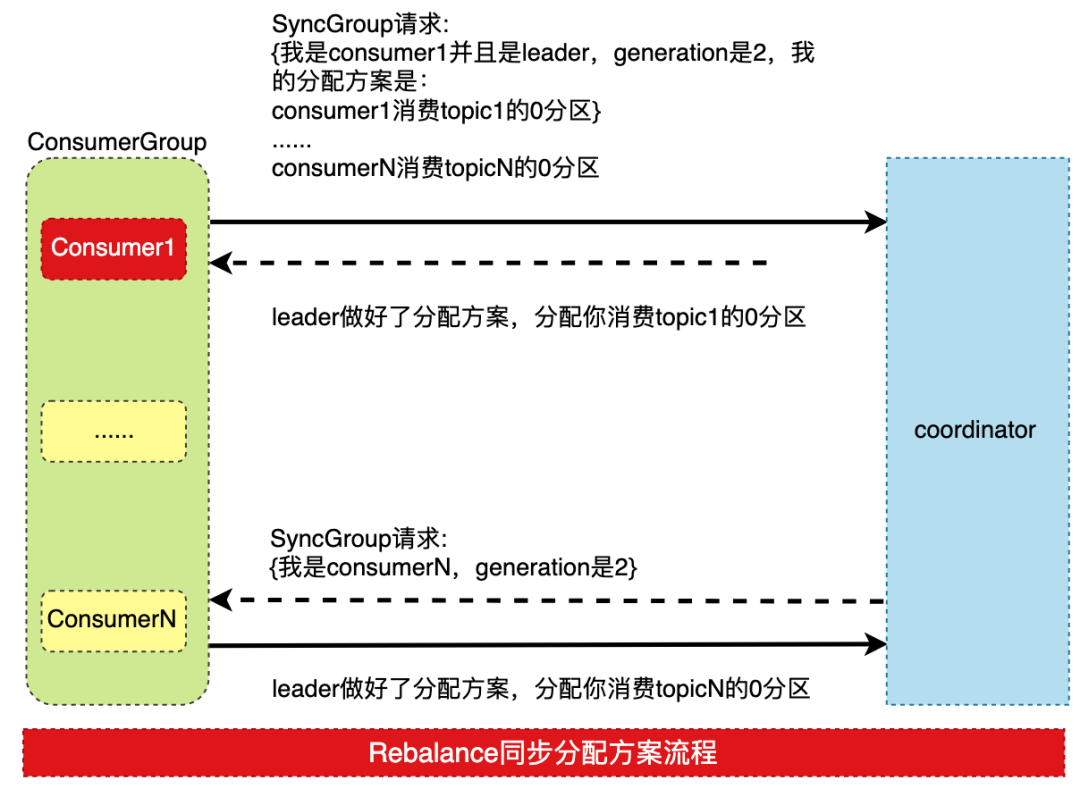
6. kafka为consumer group定义了5种状态
-
Empty
:表示group下没有任何激活的consumer,但可能包含offset信息。- 每个group创建时处于Empty状态
- 所有consumer都离开group时处于Empty状态
- 由于可能包含offset信息,所以此状态下的group可以响应 OffsetFetch请求,即返回 clients端对应的位移信息
-
PreparingRebalance
:表示group 正在准备进行 group rebalance。此状态表示group已经接收到部分JoinGroup的请求,同时在等待其他成员发送JoinGroup请求,知道所有成员都成功加入组或者超时(Kafka 0.10.1.0之后超时时间由consumer端参数max.poll.interval.ms指定)- 该状态下的 group 依然可能保存有offset信息,因此 clients 依然可以发起 OffsetFetch 请求去获取offset,甚 至还可以发起OffsetCommit请求去提交位移
-
AwaitingSync
:所有成员都已经加入组并等待 leader consumer 发送分区分配方案。同样地,此时依然可以获取位移,但若提交位移, coordinator 将会抛出REBALANCE_IN_PROGRESS
异常来表明该 group 正在进行 rebalance -
Stable
:表明 group 开始正常消费 。此时 group 必须响应 clients 发送过来的任何请求,比如位移提交请求、位移获取请求、心跳请求等 -
Dead
: 表明 group 已经彻底废弃, group 内没有任何激活consumer并且 group 的所有元数据信息都己被删除。处于此状态的 group 不会响应任何请求。严格来说, coordinator会返回UNKNOWN_MEMBER_ID
异常
7. rebalance监听器
-
如果要实现将offset存储在外部存储中,需要使用rebalance。使用 rebalance 监听器的前提是必须使用 consumer group。如果使用的是独立 consumer或是直接手动分配分区,那么 rebalance监听器是无效的
-
rebalance 监听器最常见的用法就是手动提交位移到第三方存储以及在 rebalance 前后执行一些必要的审计操作
-
自动提交位移是不需要在 rebalance监听器中再提交位移的,consumer 每次 rebalance 时会检查用户是否启用了自动提交位移,如果是,它会帮用户执行提交
-
鉴于 consumer 通常都要求 rebalance 在很短的时间内完成,用户千万不要在 rebalance 监听器 的两个方法中放入执行时间很长的逻辑,特别是一些长时间阻塞方法
-
代码案例
public class ConsumerOffsetSaveDB {
private final static Logger logger = LoggerFactory.getLogger("kafka-consumer");
@Test
public void testConsumerOffsetSaveDB() {
Properties props = new Properties();
props.put(ConsumerConfig.BOOTSTRAP_SERVERS_CONFIG, "kafka-master:9092,kafka-slave1:9093,kafka-slave2:9094");
props.put(ConsumerConfig.MAX_POLL_RECORDS_CONFIG, 10);
props.put(ConsumerConfig.ENABLE_AUTO_COMMIT_CONFIG, "false");
props.put(ConsumerConfig.AUTO_COMMIT_INTERVAL_MS_CONFIG, "1000");
props.put(ConsumerConfig.SESSION_TIMEOUT_MS_CONFIG, "30000");
props.put(ConsumerConfig.KEY_DESERIALIZER_CLASS_CONFIG, "org.apache.kafka.common.serialization.StringDeserializer");
props.put(ConsumerConfig.VALUE_DESERIALIZER_CLASS_CONFIG, "org.apache.kafka.common.serialization.StringDeserializer");
props.put(ConsumerConfig.AUTO_OFFSET_RESET_CONFIG, "earliest");
String groupId = "test_group_offset_db11";
props.put(ConsumerConfig.GROUP_ID_CONFIG, groupId);
KafkaConsumer<String, String> consumer = new KafkaConsumer<>(props);
String topic = "testTopic";
Map<TopicPartition, OffsetAndMetadata> offsetAndMetadataMap = new HashMap<>();
consumer.subscribe(Collections.singletonList(topic), new SaveOffsetsRebalance(consumer, offsetAndMetadataMap, groupId));
consumer.poll(0);
OffsetService offsetService = new OffsetService();
for (TopicPartition partition : consumer.assignment()) {
// 从数据库获取当前分区的偏移量
Offset offset = offsetService.getOffset(groupId, partition.topic(), partition.partition());
if (offset != null && offset.getOffset() != null) {
consumer.seek(partition, offset.getOffset());
} else {
logger.info("初始时库没有值");
}
}
try {
while (true) {
ConsumerRecords<String, String> records = consumer.poll(1000);
for (ConsumerRecord<String, String> record : records) {
//模拟异常
/* if (record.value().equals("hello world 10")) {
throw new RuntimeException(String.format("hello world 10处理异常,offset:%s,partition:%s",record.offset(),record.partition()));
}*/
/**
*1. 消息处理(要考虑去重,如果消息成功,但是存储偏移量失败或者宕机,此时数据库存储的消息偏移量不是最新的)
*2. 如果消息处理是数据库,最好将消息处理与存储offset放在一个事务当中
*/
logger.info("key:{},value:{},offset:{}", record.key(), record.value(), record.offset());
offsetAndMetadataMap.put(
new TopicPartition(record.topic(), record.partition()),
new OffsetAndMetadata(record.offset() + 1, "")
);
// 2.存储偏移量到DB,这里采用单次更新,当然也可以批量
offsetService.insertOffset(groupId, record.topic(), record.partition(), record.offset() + 1);
}
}
} catch (WakeupException e) {
// 不处理异常
} catch (Exception e) {
logger.error(e.getMessage(), e);
} finally {
consumer.close();
}
}
}
- rebalance代码
public class SaveOffsetsRebalance implements ConsumerRebalanceListener {
private Logger logger = LoggerFactory.getLogger(SaveOffsetsRebalance.class);
private Consumer consumer;
private Map<TopicPartition, OffsetAndMetadata> map;
private String groupId;
OffsetService offsetService = new OffsetService();
public SaveOffsetsRebalance(Consumer consumer, Map<TopicPartition, OffsetAndMetadata> map, String groupId) {
this.consumer = consumer;
this.map = map;
this.groupId = groupId;
}
/**
* 此方法在rebalance操作之前调用,用于我们提交消费者偏移,
* 这里不提交消费偏移,因为consumer中每处理一次消息就保存一次偏移,
* consumer去做重复消费处理
*/
@Override
public void onPartitionsRevoked(Collection<TopicPartition> partitions) {
int size = partitions.size();
logger.info("rebalance 之前触发.....{}", size);
Iterator<TopicPartition> iterator = partitions.iterator();
while (iterator.hasNext()) {
TopicPartition topicPartition = iterator.next();
long position = consumer.position(topicPartition);
OffsetAndMetadata offsetAndMetadata = map.get(topicPartition);
if (offsetAndMetadata != null) {
long offset = offsetAndMetadata.offset();
logger.info("position:{},offset:{}", position, offset);
} else {
logger.info("position:{},offset:{}", position, null);
}
}
}
/**
* 此方法在rebalance操作之后调用,用于我们拉取新的分配区的偏移量。
*/
@Override
public void onPartitionsAssigned(Collection<TopicPartition> partitions) {
logger.info("rebalance 之后触发.....");
for (TopicPartition partition : partitions) {
//从数据库获取当前分区的偏移量
Offset offset = offsetService.getOffset(this.groupId, partition.topic(), partition.partition());
if (offset != null && offset.getOffset() != null) {
consumer.seek(partition, offset.getOffset());
}
}
}
}
- 数据库sql
DROP TABLE IF EXISTS `t_offset`;
CREATE TABLE `t_offset` (
`id` bigint(20) NOT NULL AUTO_INCREMENT COMMENT '主键',
`group_id` varchar(50) CHARACTER SET latin1 NOT NULL,
`topic` varchar(50) CHARACTER SET latin1 NOT NULL COMMENT 'topic',
`partition` int(11) NOT NULL,
`offset` bigint(20) DEFAULT NULL,
PRIMARY KEY (`id`),
UNIQUE KEY `unique_gtp` (`group_id`,`topic`,`partition`) USING BTREE
) ENGINE=InnoDB AUTO_INCREMENT=1 DEFAULT CHARSET=utf8 COLLATE=utf8_bin COMMENT='偏移量保存';
- 添加依赖
compile group: 'commons-dbutils', name: 'commons-dbutils', version: '1.7'
compile group: 'mysql', name: 'mysql-connector-java', version: '5.1.30'
compile group: 'org.aeonbits.owner', name: 'owner', version: '1.0.9'
- 数据库工具
public class JdbcUtils {
public static Connection getConnection(String driverClass, String url, String username, String password) throws SQLException, ClassNotFoundException {
Class.forName(driverClass);
return DriverManager.getConnection(url, username, password);
}
}
@Sources({"classpath:config.properties"})
public interface MysqlJdbcConfig extends Reloadable {
@Key("config.mysql.url")
public String url();
@Key("config.mysql.username")
public String username();
@Key("config.mysql.password")
public String password();
@Key("config.mysql.driverClass")
public String driverClass();
public final static class ServerConfigInner {
public final static MysqlJdbcConfig config = ConfigFactory.create(MysqlJdbcConfig.class);
}
public static final MysqlJdbcConfig instance = ServerConfigInner.config;
}
-
配置
config.properties
config.mysql.url=jdbc:mysql://jannal.mac.com:3306/test
config.mysql.username=root
config.mysql.password=root
config.mysql.driverClass=com.mysql.jdbc.Driver
- 偏移量处理类
public class Offset {
private Long id;
private String groupId;
private String topic;
private String partition;
private Long offset;
...省略getter setter toString..
}
public class OffsetService {
public Offset getOffset(String groupId, String topic, int partition) {
QueryRunner queryRunner = new QueryRunner();
Offset offset = null;
Connection connection = null;
try {
connection = JdbcUtils.getConnection(MysqlJdbcConfig.instance.driverClass(), //
MysqlJdbcConfig.instance.url(),//
MysqlJdbcConfig.instance.username(),//
MysqlJdbcConfig.instance.password());//
String sql = "select `topic`,`partition`,`offset` from `t_offset` where `group_id`=? and `topic` = ? and `partition` = ?";
offset = queryRunner.query(connection, sql, new BeanHandler<Offset>(Offset.class), new Object[]{groupId, topic, partition});
} catch (Exception e) {
throw new RuntimeException(e.getMessage(), e);
} finally {
if (connection != null) {
try {
connection.close();
} catch (SQLException e) {
e.printStackTrace();
}
}
}
return offset;
}
public void insertOffset(String groupId, String topic, int partition, long offset) {
QueryRunner queryRunner = new QueryRunner();
Connection connection = null;
try {
connection = JdbcUtils.getConnection(MysqlJdbcConfig.instance.driverClass(), //
MysqlJdbcConfig.instance.url(),//
MysqlJdbcConfig.instance.username(),//
MysqlJdbcConfig.instance.password());//
String sql = " insert into `t_offset`( `group_id`,`topic`, `partition`,`offset`) values ( ?,?, ?,?) on duplicate key update `offset` =VALUES(offset);";
Object[] params = {groupId, topic, partition, offset};
connection.setAutoCommit(false);
queryRunner.update(connection, sql, params);
connection.commit();
} catch (Exception e) {
try {
if (connection != null) {
connection.rollback();
}
} catch (SQLException e1) {
throw new RuntimeException(e.getMessage(), e);
}
throw new RuntimeException(e.getMessage(), e);
} finally {
if (connection != null) {
try {
connection.close();
} catch (SQLException e) {
e.printStackTrace();
}
}
}
}
public void batchInsertOffset(Object[][] params) {
QueryRunner queryRunner = new QueryRunner();
Connection connection = null;
try {
connection = JdbcUtils.getConnection(MysqlJdbcConfig.instance.driverClass(), //
MysqlJdbcConfig.instance.url(),//
MysqlJdbcConfig.instance.username(),//
MysqlJdbcConfig.instance.password());//
String sql = " insert into `t_offset`(`group_id`, `topic`, `partition`,`offset`) values (?, ?, ?,?) on duplicate key update `offset`=VALUES(offset);";
connection.setAutoCommit(false);
queryRunner.batch(connection, sql, params);
connection.commit();
} catch (Exception e) {
try {
if (connection != null) {
connection.rollback();
}
} catch (SQLException e1) {
throw new RuntimeException(e.getMessage(), e);
}
throw new RuntimeException(e.getMessage(), e);
} finally {
if (connection != null) {
try {
connection.close();
} catch (SQLException e) {
e.printStackTrace();
}
}
}
}
}
如果这个文章对你有帮助,不要忘记
「在看」 「点赞」 「收藏」
三连啊喂!

我在B站读大学,大数据专业
我们在学习Flink的时候,到底在学习什么? 193篇文章暴揍Flink,这个合集你需要关注一下 Flink生产环境TOP难题与优化,阿里巴巴藏经阁YYDS Flink CDC我吃定了耶稣也留不住他!| Flink CDC线上问题小盘点 我们在学习Spark的时候,到底在学习什么? 在所有Spark模块中,我愿称SparkSQL为最强! 硬刚Hive | 4万字基础调优面试小总结 数据治理方法论和实践小百科全书
标签体系下的用户画像建设小指南
4万字长文 | ClickHouse基础&实践&调优全视角解析
【面试&个人成长】2021年过半,社招和校招的经验之谈 大数据方向另一个十年开启 |《硬刚系列》第一版完结 我写过的关于成长/面试/职场进阶的文章 当我们在学习Hive的时候在学习什么?「硬刚Hive续集」