Swin Transformer的继任者
点蓝色字关注“机器学习算法工程师”
设为星标,干货直达!
近期,随着PVT和Swin Transformer的成功,让我们看到了将ViT应用在dense prediction的backbone的巨大前景。PVT的核心是金字塔结构,同时通过对attention的keys和values进行downsample来进一步减少计算量,但是其计算复杂度依然和图像大小()的平成正比。而Swin Transformer在金字塔结构基础上提出了window attention,这其实本质上是一种local attention,并通过shifted window来建立cross-window的关系,其计算复杂度和图像大小()成正比。基于local attention的模型计算复杂低,但是也丧失了global attention的全局感受野建模能力。近期,在Swin Transformer之后也有一些基于local attention的工作,它们从不同的方面来提升模型的全局建模能力。
Twins
美团提出的Twins思路比较简单,那就是将local attention和global attention结合在一起。Twins主体也采用金字塔结构,但是每个stage中交替地采用LSA(Locally-grouped self-attention)和GSA(Global sub-sampled attention),这里的LSA其实就是Swin Transformer中的window attention,而GSA就是PVT中采用的对keys和values进行subsapmle的MSA。LSA用来提取局部特征,而GSA用来实现全局感受野:
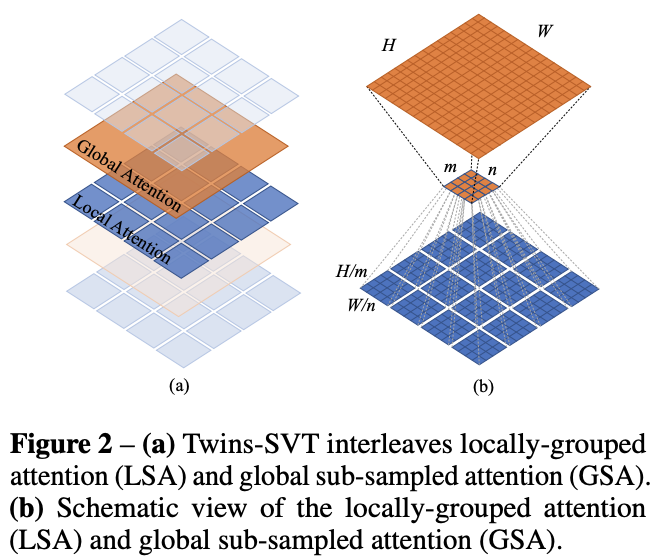
此外,Twins还引入了美团之前论文CPVT提出的PEG(position encoding generator)来进行位置编码,具体是在每个stage的第一个transfomer encoder后插入一个PEG(具体实现上是一个3x3的depth-wise conv)。如果将PVT中的位置编码用PEG替换(称为Twins-PCPVT),那么模型效果也有一个明显的提升。
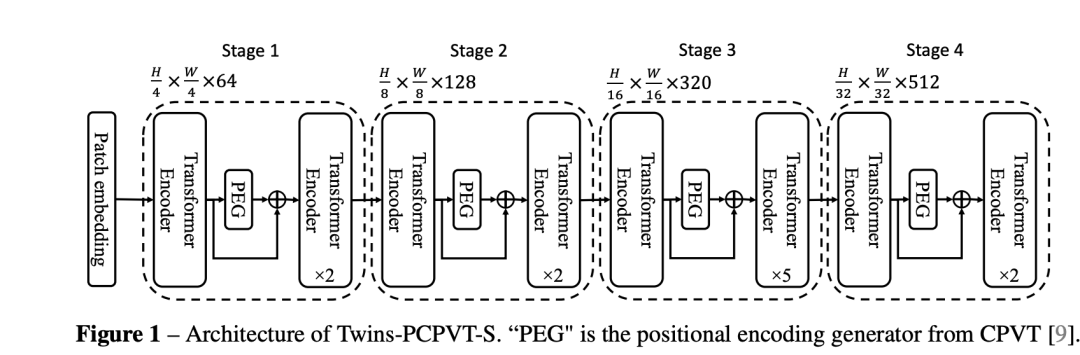
同样地,用了PEG后,可以将window attention中的相对位置编码也去掉了(相比Swin Transformer),最终的模型称为Twins-SVT。在224x224输入的ImageNet数据集上,可以看到Twins-SVT分类效果超过了Swin,而且模型参数和计算量均更低。
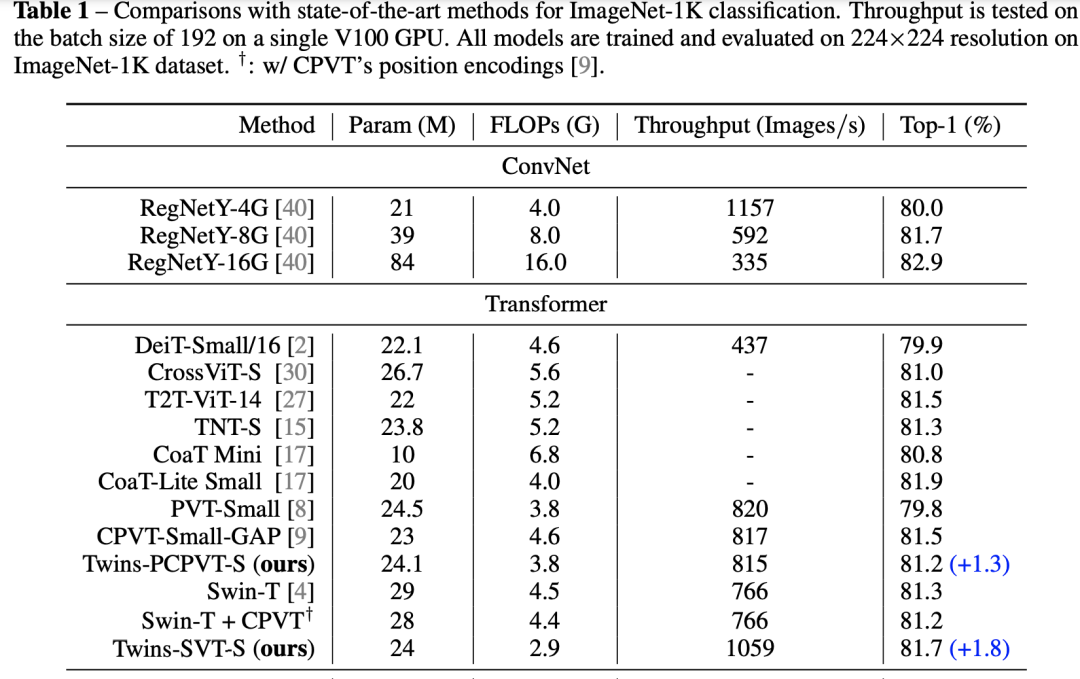
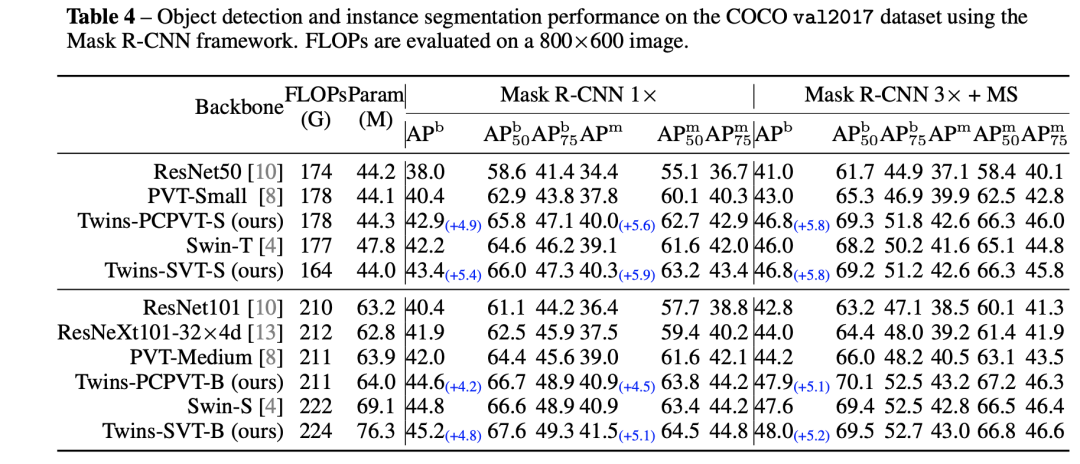
MSG-Transformer
华为提出的MSG-Transformer主要思路是为每个window增加一个信使token(messenger token, MSG),这个不同的windows通过MSG token来建立联系,具体的操作是对MSG token进行shuffle。下图中图像共分为个windows(绿色线条),而每个windows组成一个shuffle region;每个Window都包含一个MSG token,经过window attention之后,同一个shuffle region的MSG token将先进行shuffle,最后才送入MLP中。
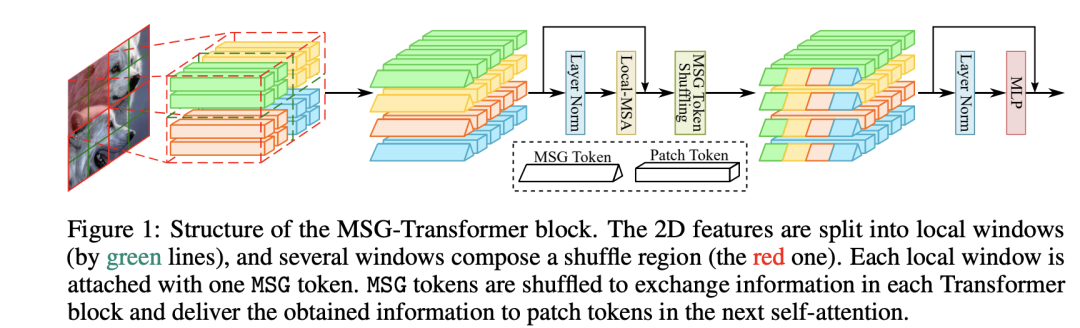
对于一个shuffle region,这里记其大小为,其MSG tokens组合在一起记为,这里是特征维度大小。MSG token的shuffle可以通过reshape->transpose->reshape来实现:
其实就是对MSG tokens的特征进行shuffle,这样shuffle后每个window的MSG token将包含其它windows的部分MSG token特征,从而完成不同windows之间的消息传递:
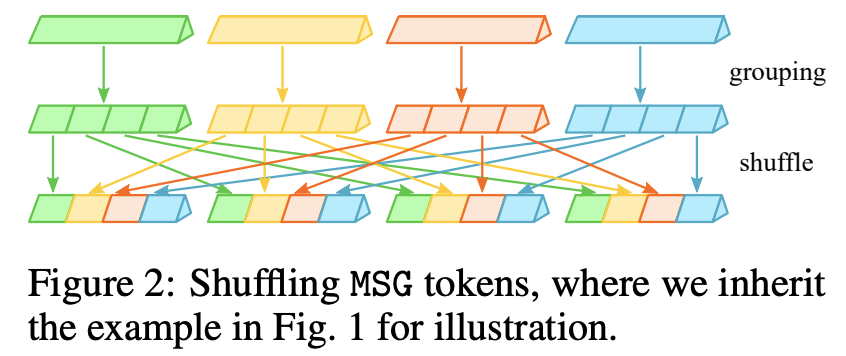
而MSG Transformer主体也采用金字塔结构,不同的stage的取值不同,对于分类任务,各个stage的分别为4,4,2,1。在实现上,我们可以将同一个shuffle region区域放在维度1,而总的shuffle regions和Batch放在第一个维度,这样就非常实现MSG tokens的shuffle:
def window_partition(x, window_size, shuf_size):
"""
Args:
x: (B, H, W, C)
window_size (int): window size
shuf_size (int): shuffle region size
Returns:
windows: (B*num_region, shuf_size**2, window_size**2, C)
"""
B, H, W, C = x.shape
x = x.view(B, H // window_size // shuf_size, shuf_size, window_size,
W // window_size // shuf_size, shuf_size, window_size, C)
windows = x.permute(0, 1, 4, 2, 5, 3, 6, 7).contiguous().view(-1, shuf_size**2, window_size**2, C)
return windows
def shuffel_msg(x):
# (B, G, win**2+1, C)
B, G, N, C = x.shape
if G == 1:
return x
msges = x[:, :, 0] # (B, G, C)
assert C % G == 0
msges = msges.view(-1, G, G, C//G).transpose(1, 2).reshape(B, G, 1, C)
x = torch.cat((msges, x[:, :, 1:]), dim=2)
return x
MSG Transformer的window attention和Swin Transformer一样也采用相对位置编码,但是多了一个MSG token,所以相对位置编码多了两个参数(其它patch tokens相对MSG token,MSG token相对其它patch tokens)。另外在每个stage开始的token merging操作,对MSG token也采取类似的处理:2x2个windows的MSG token进行concat,并进行线性变换。
MSG Transformer引入的MSG token对计算量和模型参数都影响不大,所以其和Swin Transformer一样其计算复杂度线性于图像大小。在ImageNet上,其模型效果和Swin接近,但其在CPU上速度较快:
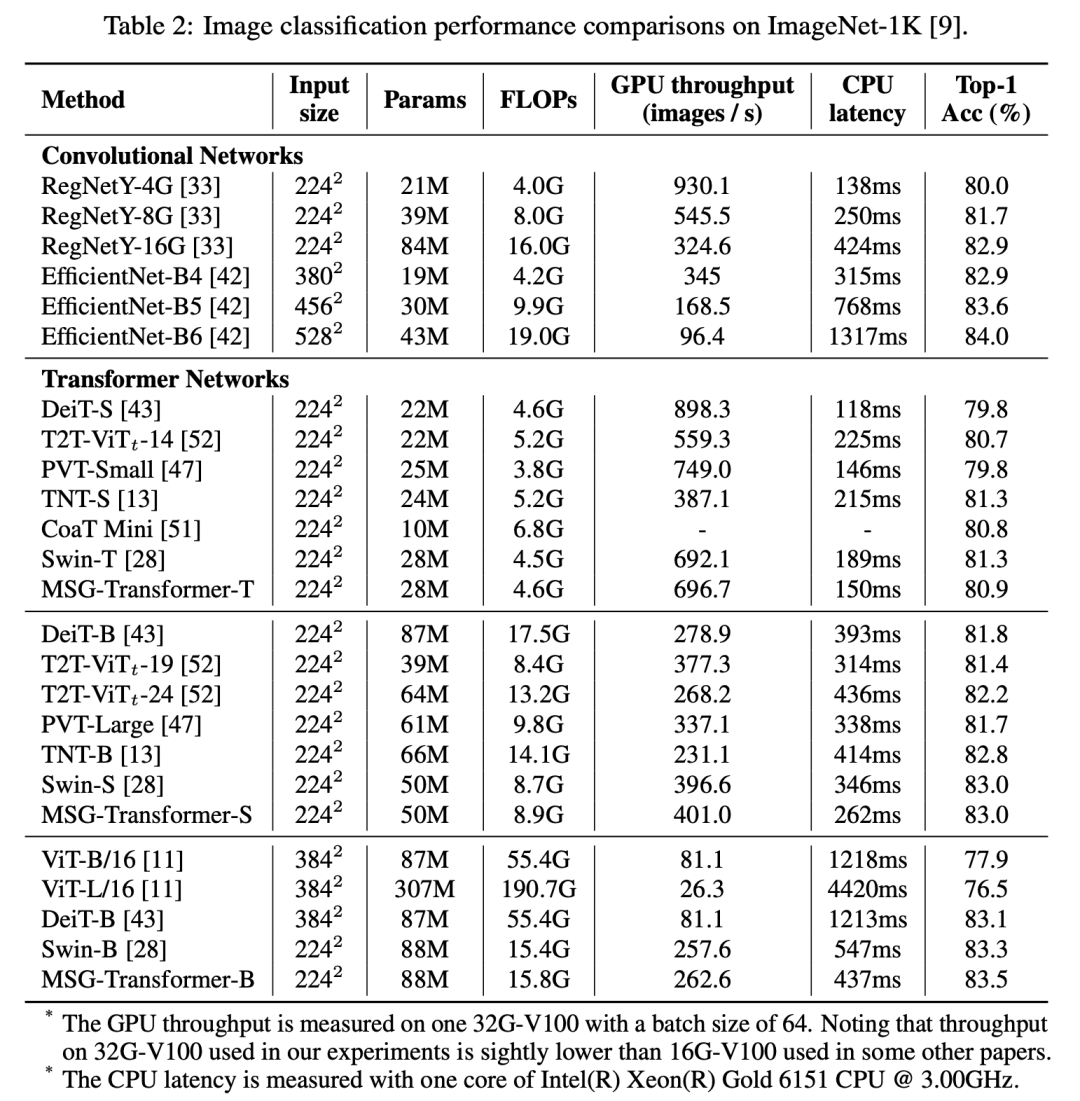
在COCO数据集上,基于Mask R-CNN模型,也可以和Swin模型取得类似的效果:
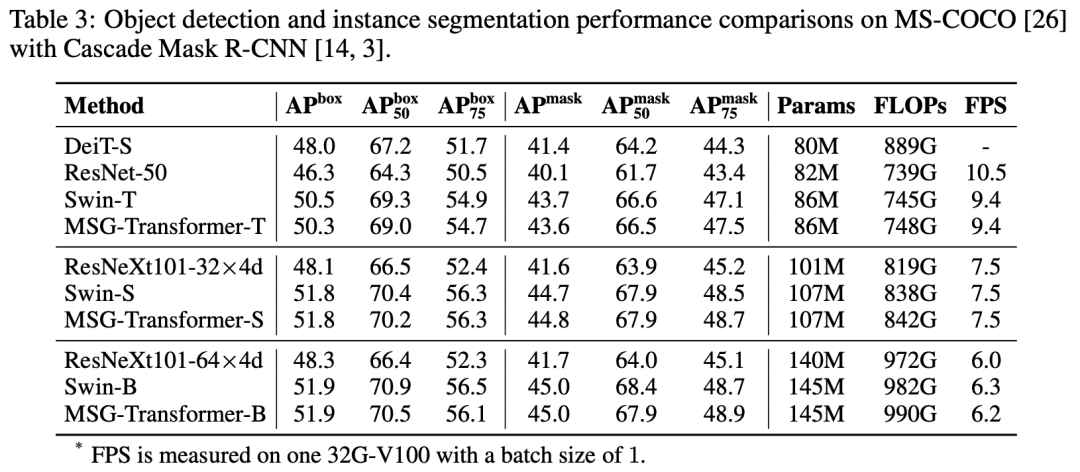
GG-Transformer
上海交大提出的GG Transformer其主要思路是改变window的划分方式,window不再局限于一个local region,而是来自全局。这里提出的一个操作是AdaptivelyDilatedSplitting
,即window的token是通过以一定的adaptive dilation rate 来采样获得,下面是一个实例(2x2个windows):
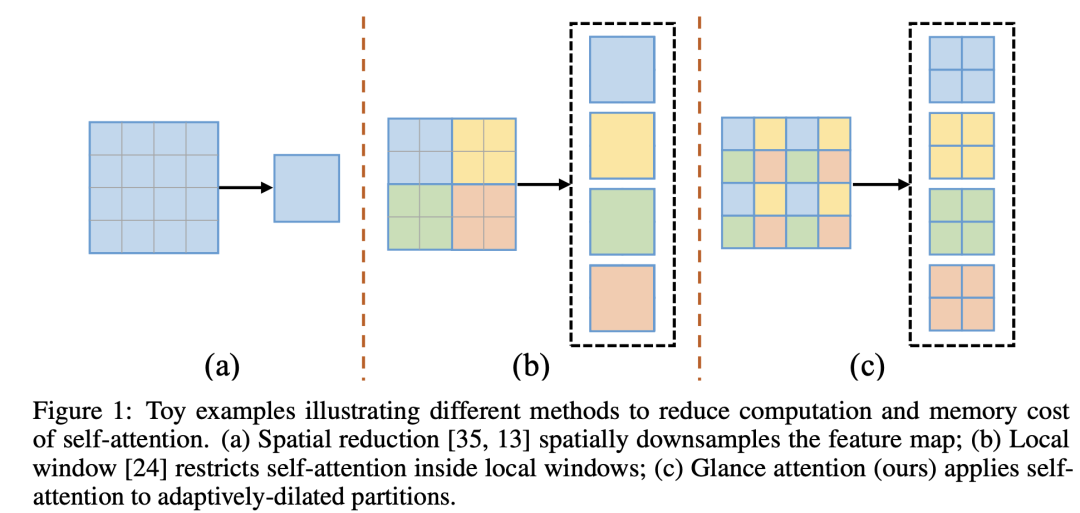
如果这样划分window,那么window attention将具有全局视野,但是相邻的patchs之间缺乏交互,所以GG Transformer又增加了一个额外的Gaze分支:先将attention中的values进行Merging
操作,其实就是AdaptivelyDilatedSplitting
的逆变换,那么将得到正常的tokens排列,然后通过一个depth-wise conv来提取局部信息,再通过AdaptivelyDilatedSplitting
操作得到和attention一样的windows,再加上attention后的特征即可:
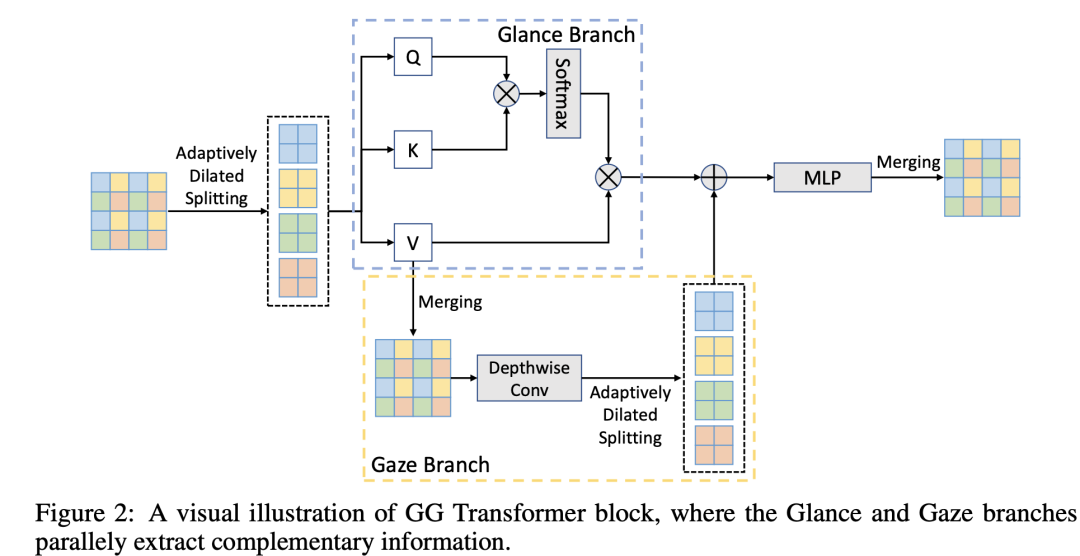
论文里将这种结构分成Glance
和Gaze
两个分支,分别用来提取全局和局部信息,类比人类的Glance and Gaze行为。这里的AdaptivelyDilatedSplitting
其实可以通过前面说的shuffle操作来实现,后面要讲的Shuffle Transformer也是一样的原理。论文中也没有提到位置编码,估计Gaze
分支的卷积可以隐式地编码位置信息。
在ImageNet上,GG-Transformer在同样的参数和算力下,其模型效果要优于Swin模型:
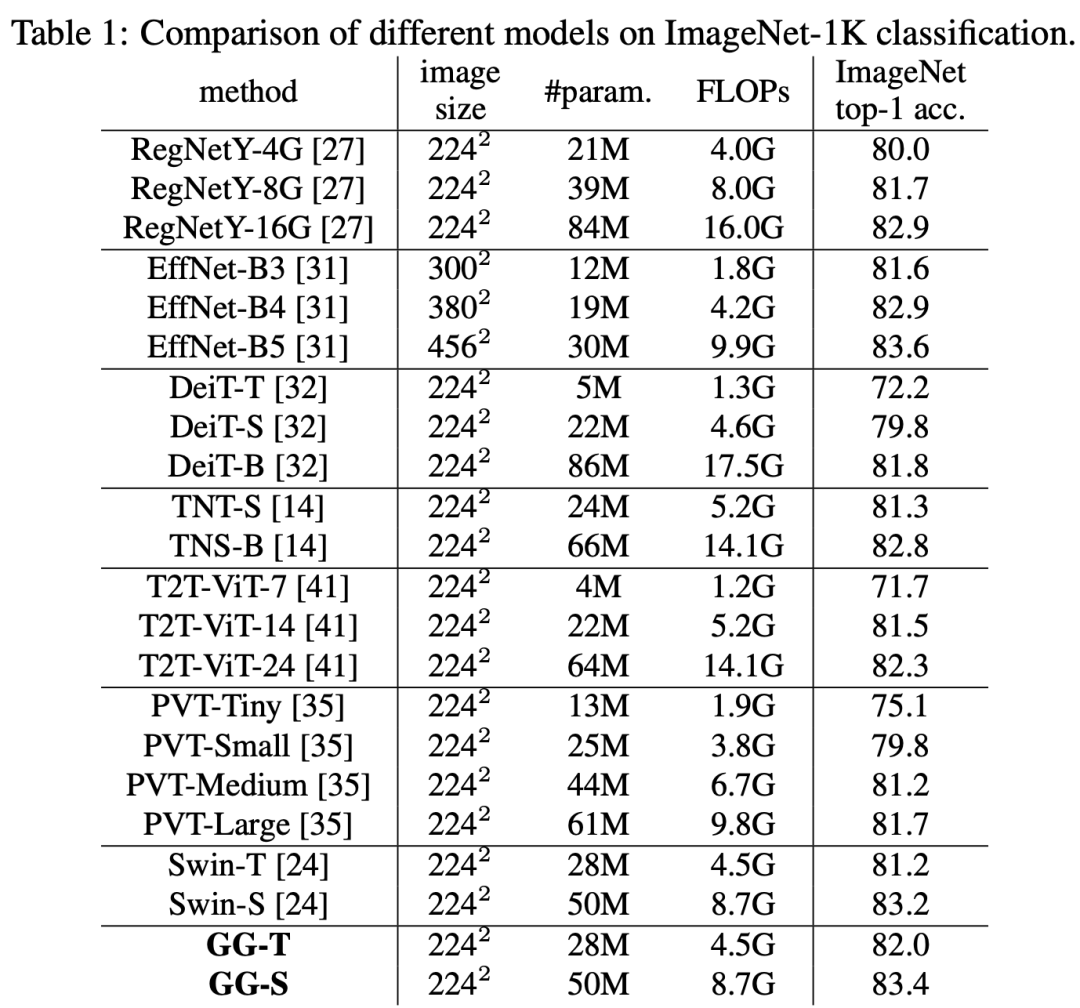
在COCO数据集上,基于Mask R-CNN,其模型效果也要优于Swin:
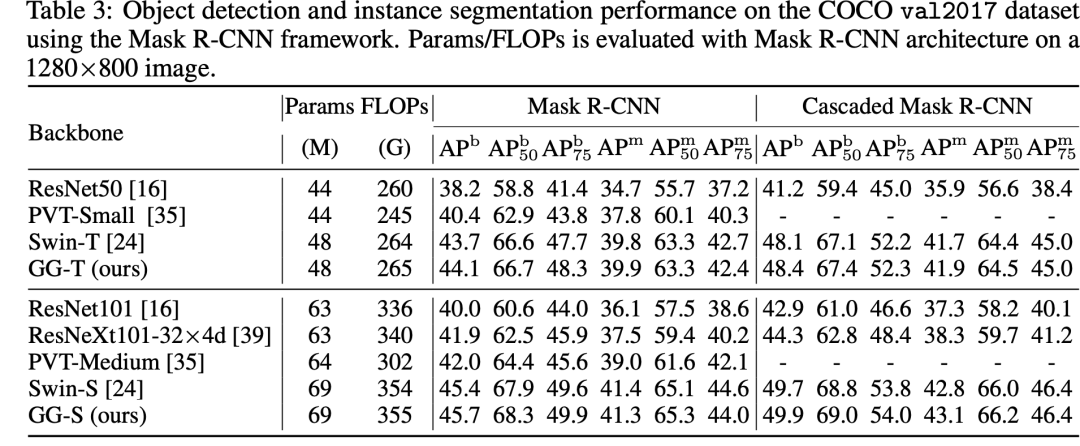
Shuffle Transformer
腾讯提出的Shuffle Transformer其核心思路是通过spatial shuffle来建立cross-window之间联系。这里的spatial shuffle和ShuffleNet中的channel shuffle类似,通过spatial shuffle可以将来自不同windows的token组成新的window:
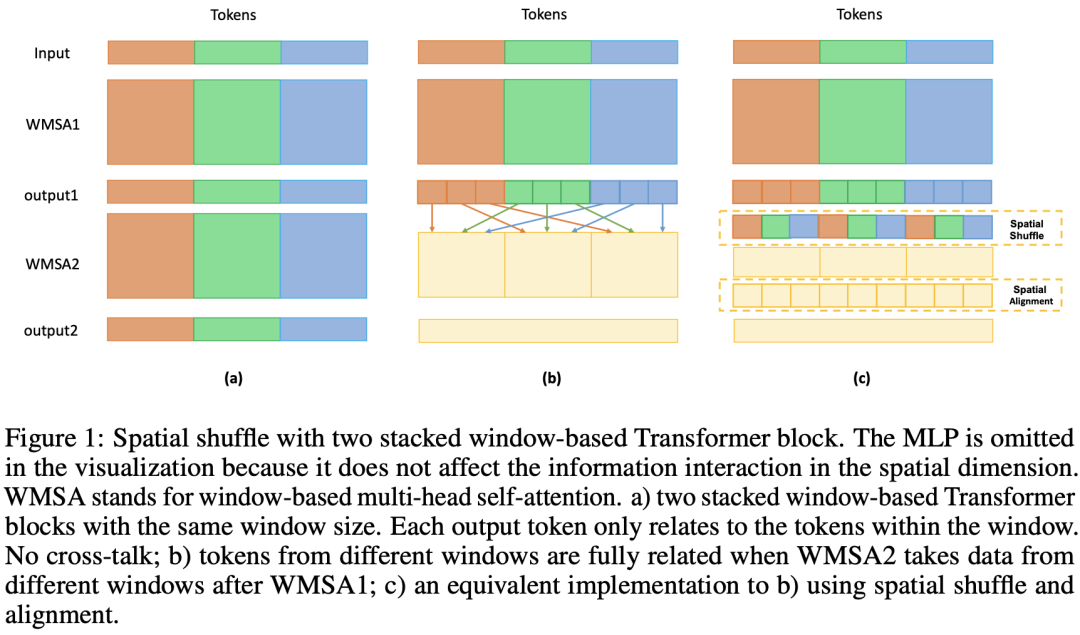
这个实现上应该是和AdaptivelyDilatedSplitting
等价的,另外MSG Transfomer也是通过MSG tokens的channel shuffle来建立不同windows间的联系。它们的实现都是类似的:reshape->transpose->reshape。开源代码也给出了具体实现:
if self.shuffle:
q, k, v = rearrange(qkv, 'b (qkv h d) (ws1 hh) (ws2 ww) -> qkv (b hh ww) h (ws1 ws2) d', h=self.num_heads, qkv=3, ws1=self.ws, ws2=self.ws)
# 这里其实是三种操作
# reshape: qkv = qkv.reshape(b, 3, h, d, ws1, hh, ws2, ww)
# transpose:qkv = qkv.transpose(1, 0, 5, 7, 2, 4, 6, 3)
# reshape: q, k, v = qkv.reshape(3, b*hh*ww, h, ws1*ws2, d)
else:
q, k, v = rearrange(qkv, 'b (qkv h d) (hh ws1) (ww ws2) -> qkv (b hh ww) h (ws1 ws2) d', h=self.num_heads, qkv=3, ws1=self.ws, ws2=self.ws)
# 注意正常window split与shuffle版本的区别,第一步reshape有区别
与Swin Transformer模型类似,Shuffle Transformer交替地采用标准的WMSA和shuffle SWMSA:
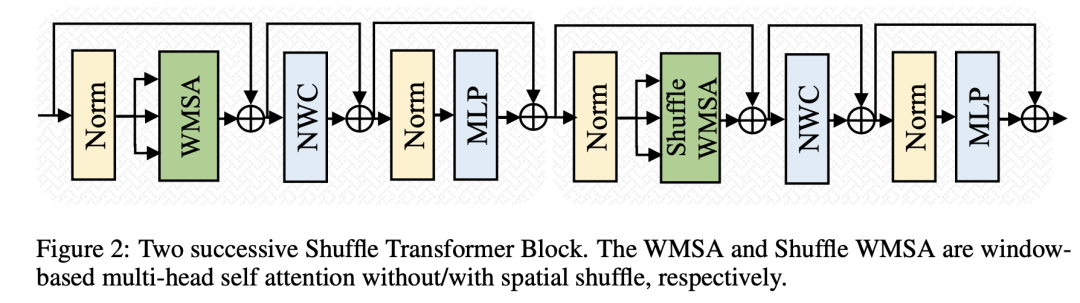
可以看到,Shuffle Transformer在WMSA操作后增加了一个NWC操作,这个其实是一个depthwise conv,其kernel size和window size一样,用于增强Neighbor-Window Connection。
class Block(nn.Module):
def __init__(self, dim, out_dim, num_heads, window_size=1, shuffle=False, mlp_ratio=4., qkv_bias=False, qk_scale=None, drop=0., attn_drop=0.,
drop_path=0., act_layer=nn.ReLU6, norm_layer=nn.BatchNorm2d, stride=False, relative_pos_embedding=False):
super().__init__()
self.norm1 = norm_layer(dim)
self.attn = Attention(
dim, num_heads=num_heads, window_size=window_size, shuffle=shuffle, qkv_bias=qkv_bias, qk_scale=qk_scale,
attn_drop=attn_drop, proj_drop=drop, relative_pos_embedding=relative_pos_embedding)
# NWC
self.local = nn.Conv2d(dim, dim, window_size, 1, window_size//2, groups=dim, bias=qkv_bias)
self.drop_path = DropPath(drop_path) if drop_path > 0. else nn.Identity()
self.norm2 = norm_layer(dim)
mlp_hidden_dim = int(dim * mlp_ratio)
self.mlp = Mlp(in_features=dim, hidden_features=mlp_hidden_dim, out_features=out_dim, act_layer=act_layer, drop=drop, stride=stride)
self.norm3 = norm_layer(dim)
print("input dim={}, output dim={}, stride={}, expand={}, num_heads={}".format(dim, out_dim, stride, shuffle, num_heads))
def forward(self, x):
x = x + self.drop_path(self.attn(self.norm1(x)))
x = x + self.local(self.norm2(x)) # local connection
x = x + self.drop_path(self.mlp(self.norm3(x)))
return x
从结构上看,Shuffle Transformer几乎和Swin Transformer一样。在ImageNet数据集上,同等条件上Shuffle Transformer相比Swin提升明显:
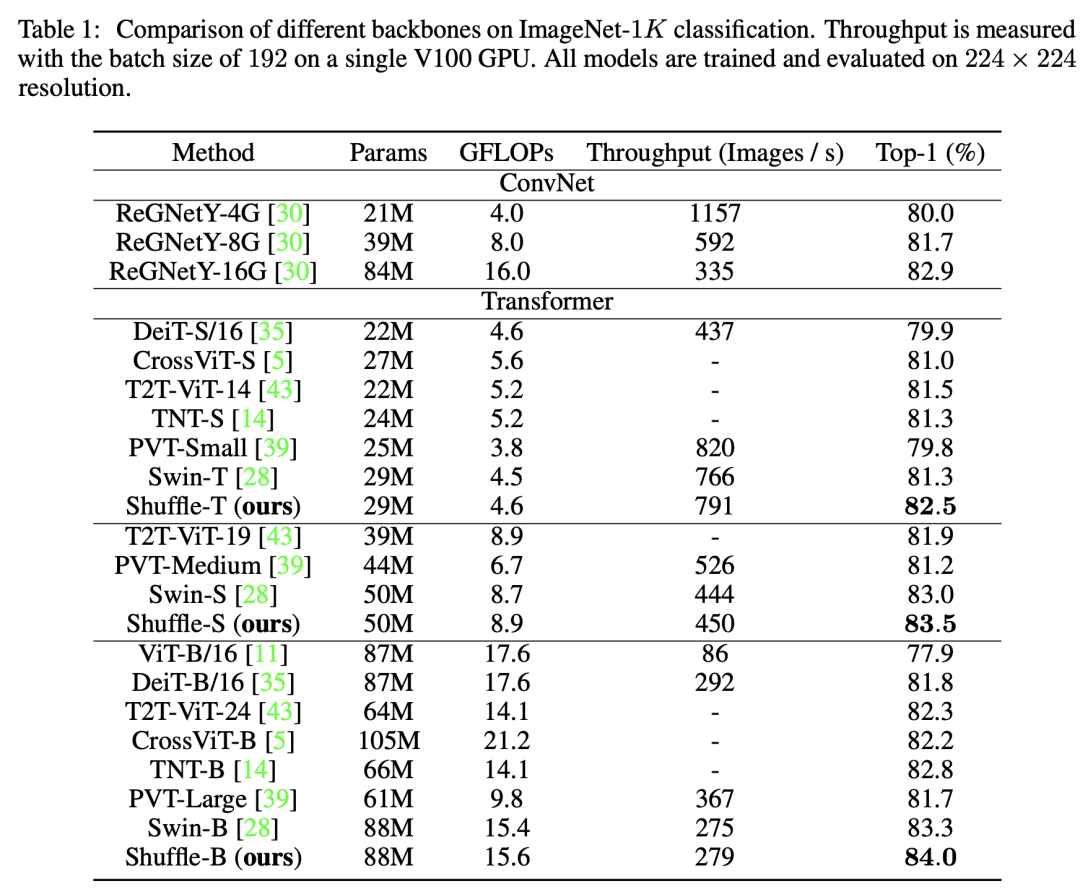
在COCO数据集上,基于Mask R-CNN,Shuffle Transformer和Swin性能不相上下:
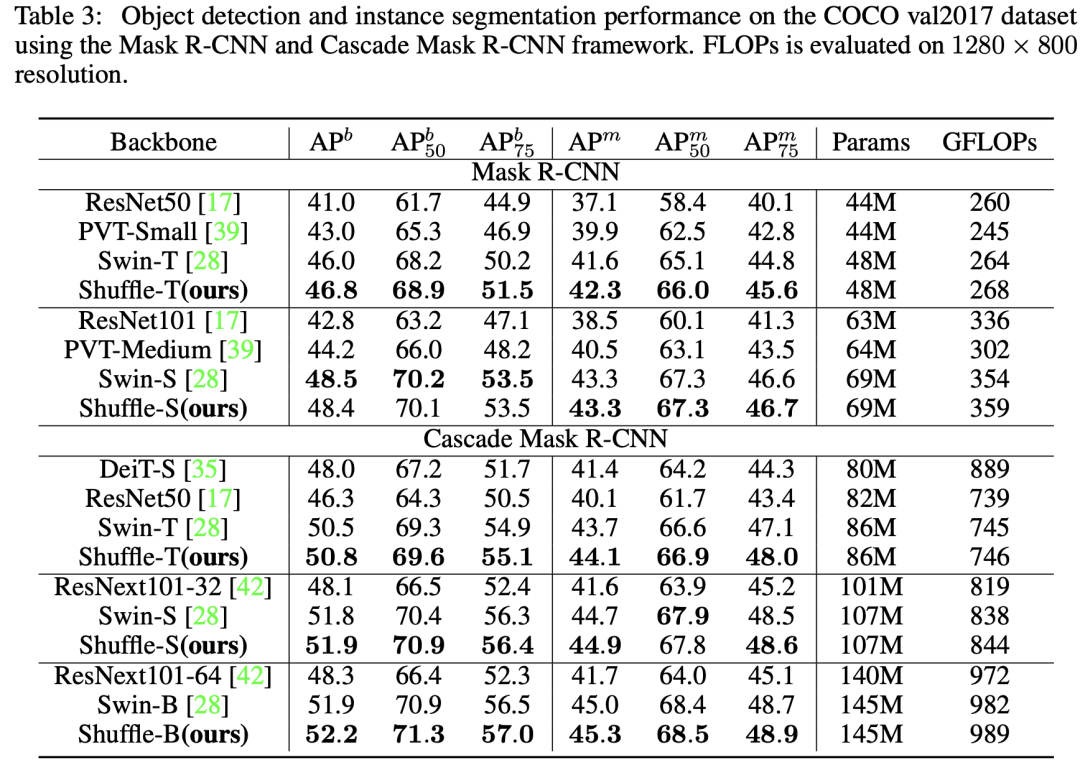
后话
可以看到,这四个模型和Swin Transformer本质上都是一种local attention,只不过它们从不同地方式来增强local attention的全局建模能力。而且,在相似的参数和计算量的条件下,5种模型在分类任务和dense任务上表现都是类似的。近期,微软在论文Demystifying Local Vision Transformer: Sparse Connectivity, Weight Sharing, and Dynamic Weight上系统地总结了Local Vision Transformer的三大特性:
Sparse connectivity:每个token的输出只依赖于其所在local window上tokens,而且各个channel之间是无联系的;(这里忽略了attention中query,key和valude的linear projections,那么attention就其实可以看成在计算好的权重下对tokens的特征进行加权求和,而且是channel-wise的) Weight sharing:权重对于各个channel是共享的; Dynamic weight:权重不是固定的,而是基于各个tokens动态生成的。
那么local attention就和Depth-Wise Convolution就很相似,首先后者也具有Sparse connectivity:只在kernel size范围内,而且各个channel之间无连接。而Depth-Wise Convolution也具有weight sharing,但是卷积核是在所有的空间位置上共享的,但不同channle采用不同的卷积核。另外depth-wise convolution的卷积核是训练参数,一旦完成训练就是固定的,而不是固定的。另外local attention丢失了位置信息,需要位置编码,但是depth-wise convolution不需要。下图是不同操作的区别:
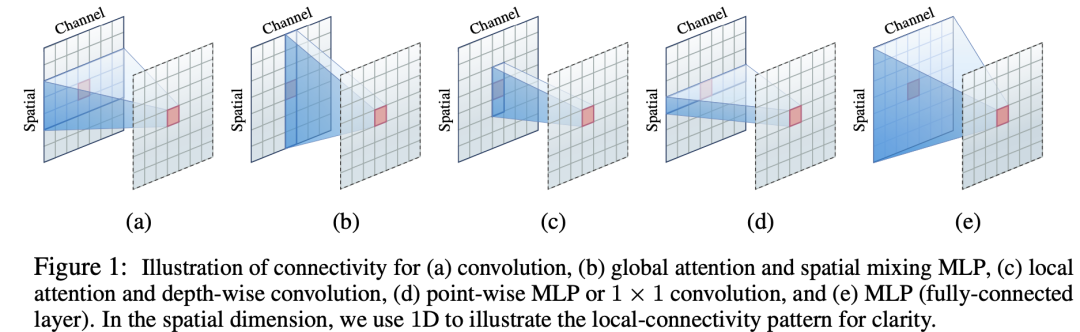
论文中也设计了基于depth-wise convolution的模型,和Swin模型结构类似:
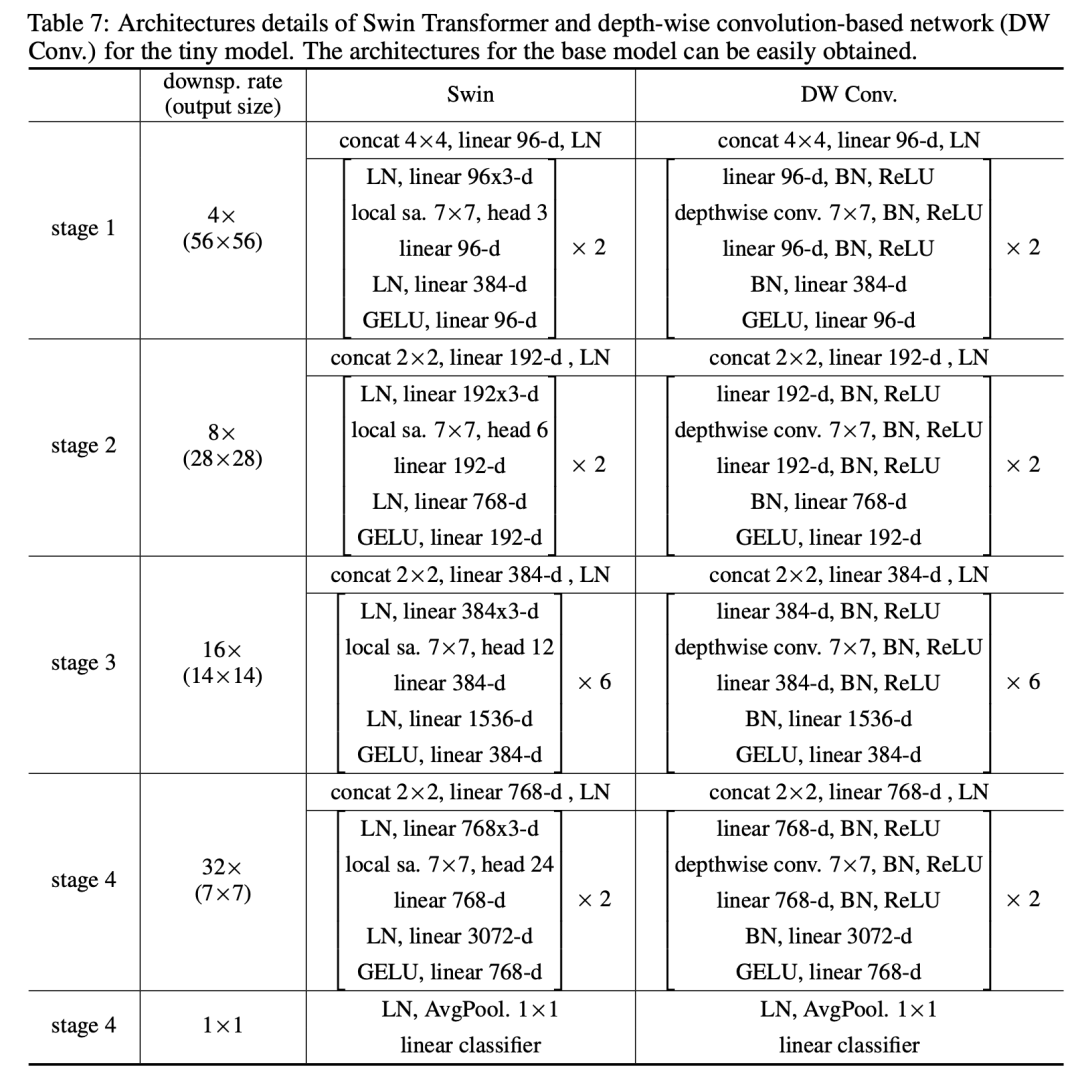
在ImageNet数据集上,DW-Conv模型效果和Swin模型相当(这里D-DW-Conv增加了动态权重的特性,类似SE模块来动态生成kernel weights):
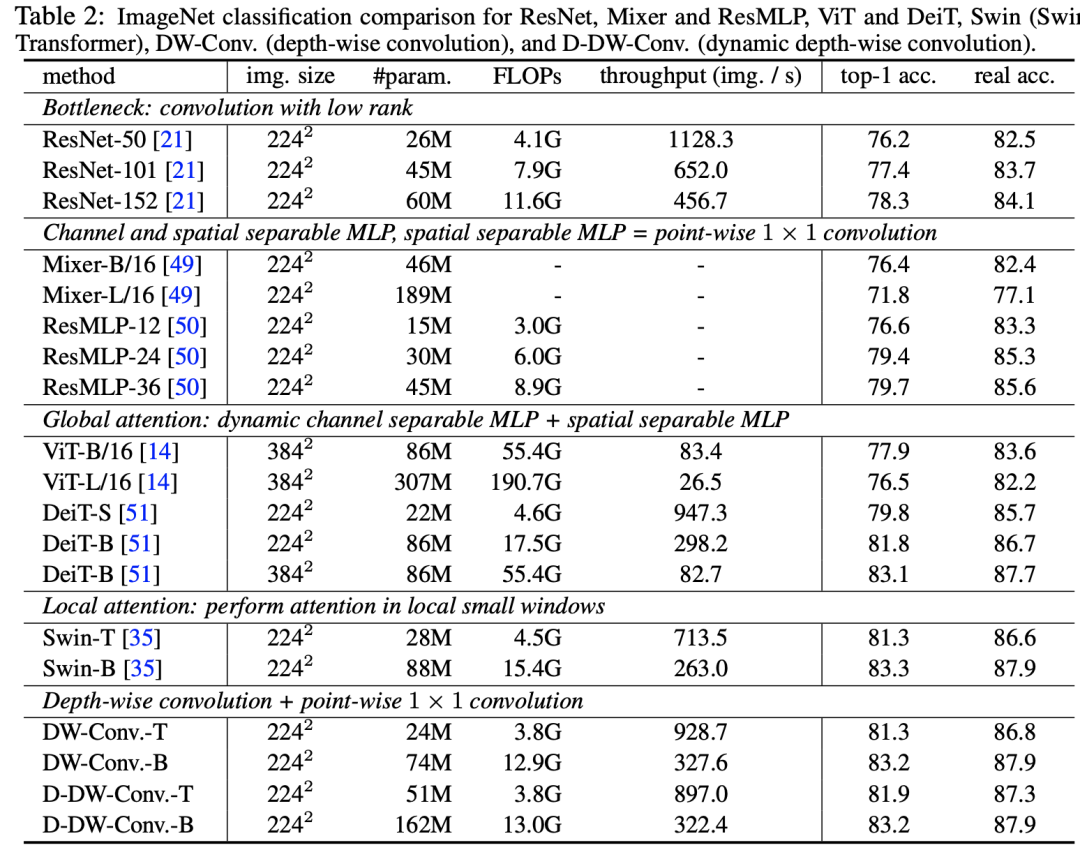
从这项研究来看,设计好的Conv模型在性能上也是可以和local attention模型匹敌的,也许local attention模型反而退化到了CNN模型。一点体外话是之前的CNN模型一般常采用3x3和1x1比较小的卷积核,但是这里采用7x7的卷积核反而大幅度提升模型效果(相比ResNet50),这里也值得深思。
参考
Shuffle Transformer: Rethinking Spatial Shuffle for Vision Transformer Twins: Revisiting the Design of Spatial Attention in Vision Transformers Glance-and-Gaze Vision Transformer MSG-Transformer: Exchanging Local Spatial Information by Manipulating Messenger Tokens Pyramid Vision Transformer: A Versatile Backbone for Dense Prediction without Convolutions Swin Transformer: Hierarchical Vision Transformer using Shifted Windows Demystifying Local Vision Transformer: Sparse Connectivity, Weight Sharing, and Dynamic Weight
推荐阅读
谷歌AI用30亿数据训练了一个20亿参数Vision Transformer模型,在ImageNet上达到新的SOTA!
"未来"的经典之作ViT:transformer is all you need!
PVT:可用于密集任务backbone的金字塔视觉transformer!
涨点神器FixRes:两次超越ImageNet数据集上的SOTA
不妨试试MoCo,来替换ImageNet上pretrain模型!
机器学习算法工程师
一个用心的公众号