ResNets首次反超有监督学习!DeepMind用自监督实现逆袭,无需标注
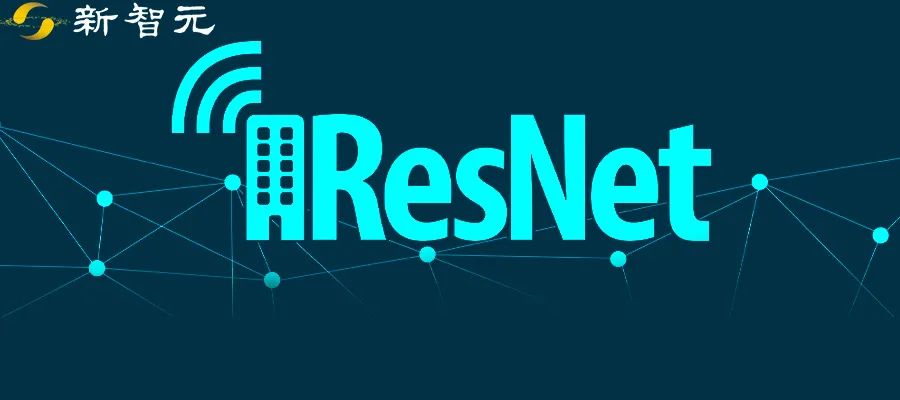
新智元报道
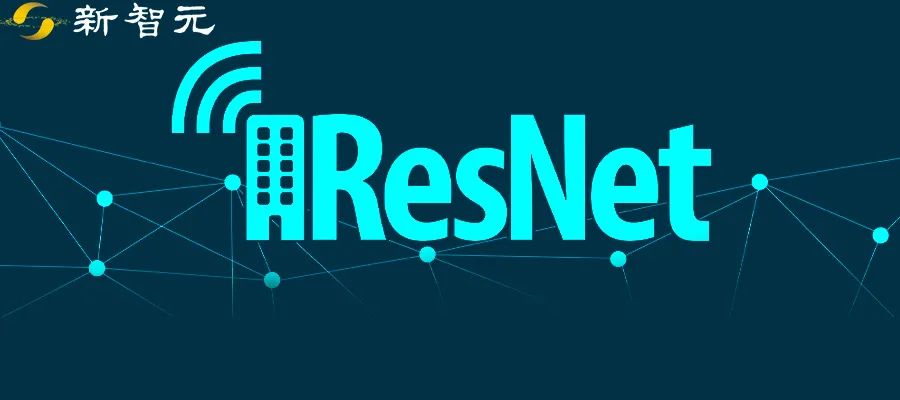
新智元报道
编辑:好困 袁榭
【新智元导读】近日,DeepMind又整了个新活:RELIC第二代!首次用自监督学习实现了对有监督学习的超越。莫非,今后真的不用标注数据了?
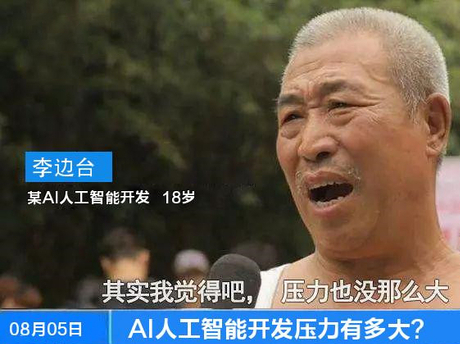
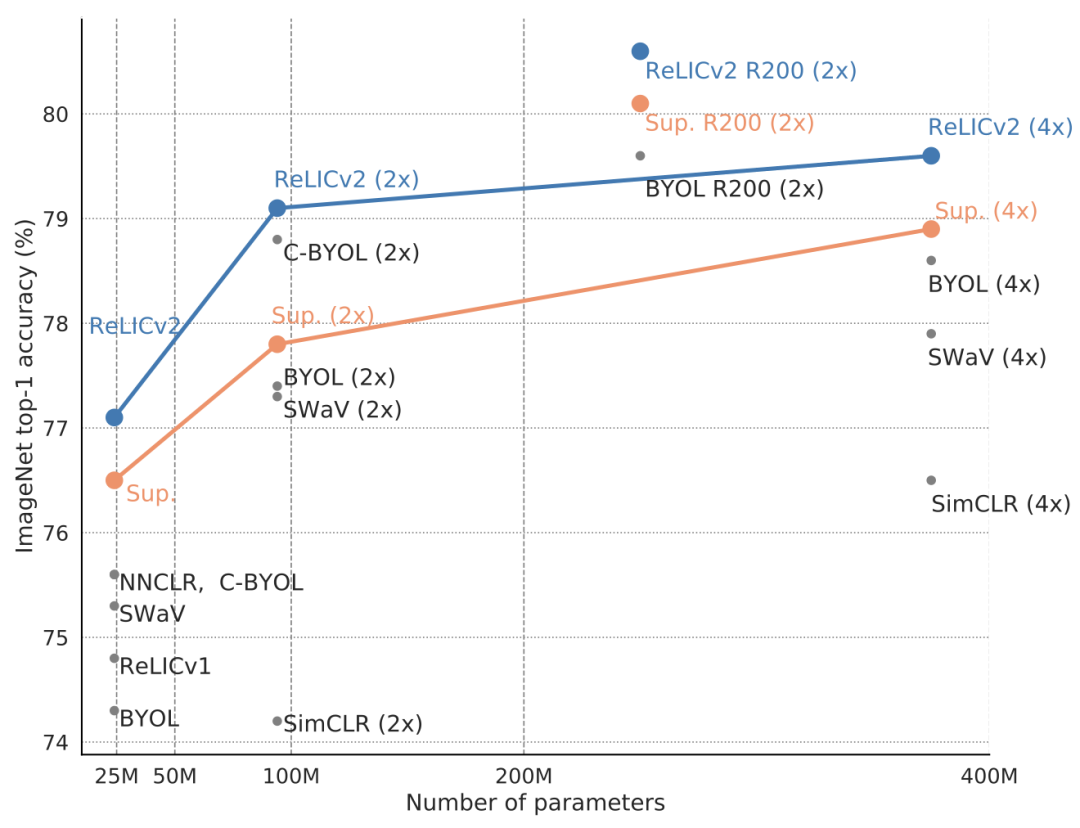
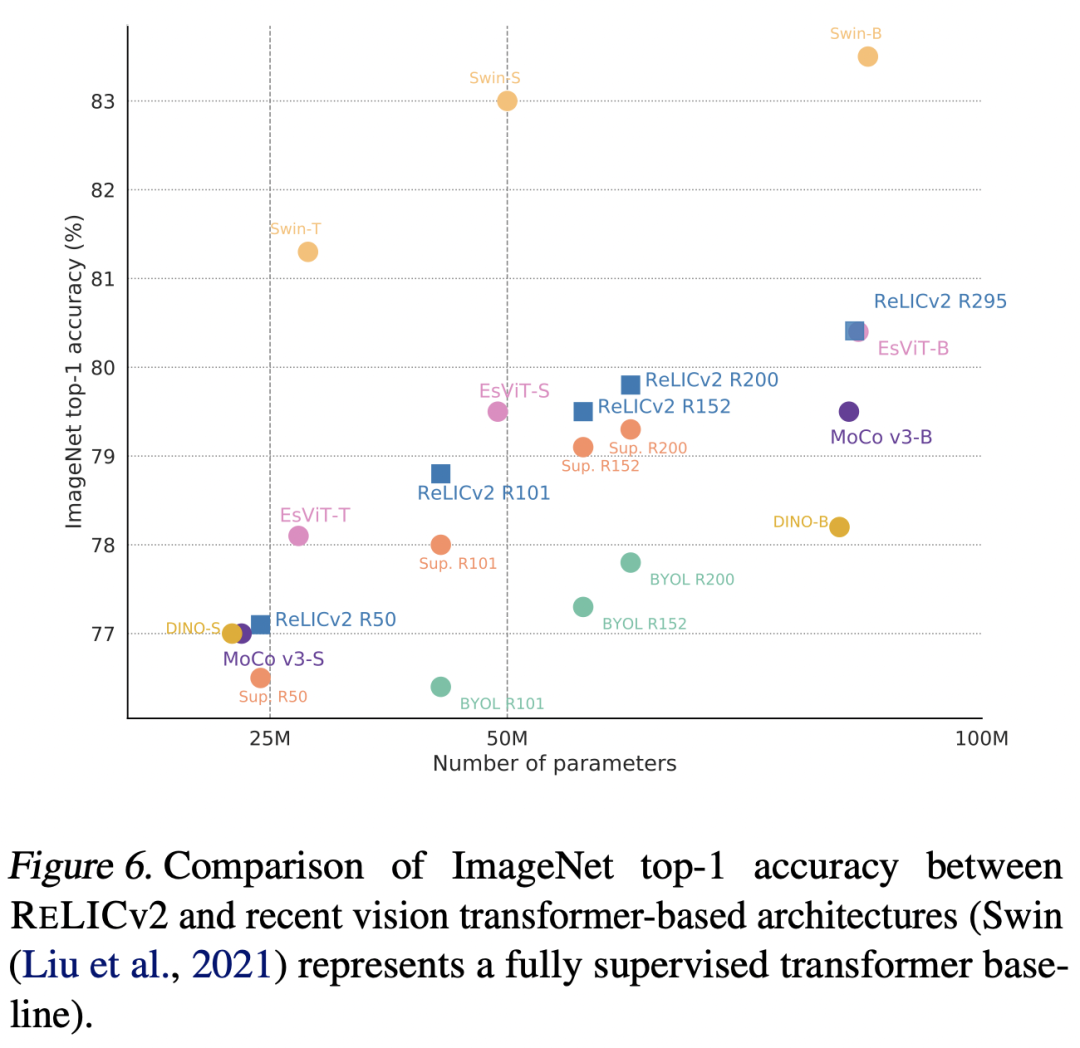
方法
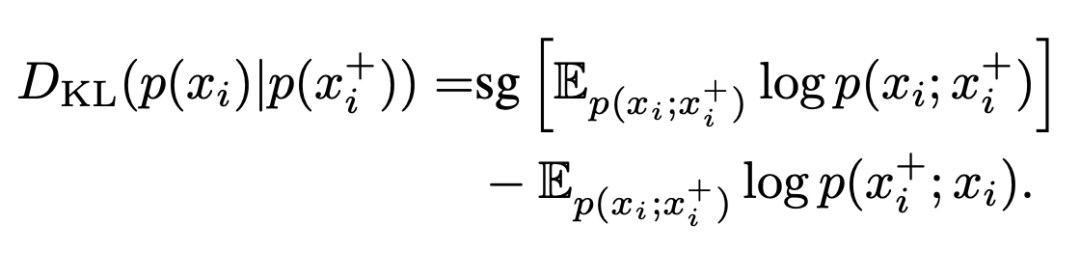
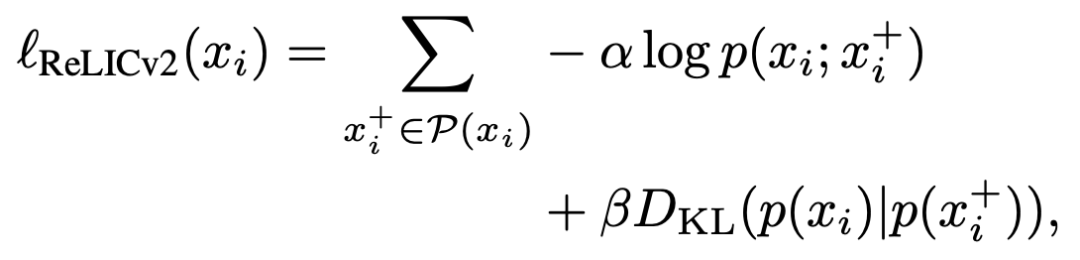
for x in batch: # load a batch of B samples
# Apply saliency mask and remove background
x_m = remove_background(x)
for i in range(num_large_crops):
# Select either original or background-removed
# Image with probability p_m
x = Bernoulli(p_m) ? x_m : x
# Do large random crop and augment
xl_i = aug(crop_l(x))
ol_i = f_o(xl_i)
tl_i = g_t(xl_i)
for i in range(num_small_crops):
# Do small random crop and augment
xs_i = aug(crop_s(x))
# Small crops only go through the online network
os_i = f_o(xs_i)
loss = 0
# Compute loss between all pairs of large crops
for i in range(num_large_crops):
for j in range(num_large_crops):
loss += loss_relicv2(ol_i, tl_j, n_e)
# Compute loss between small crops and large crops
for i in range(num_small_crops):
for j in range(num_large_crops):
loss += loss_relicv2(os_i, tl_j, n_e)
scale = (num_large_crops + num_small_crops) * num_large_crops
loss /= scale
# Compute grads, update online and target networks
loss.backward()
update(f_o)
g_t = gamma * g_t + (1 - gamma) * f_o
RELICv2的伪代码
结果
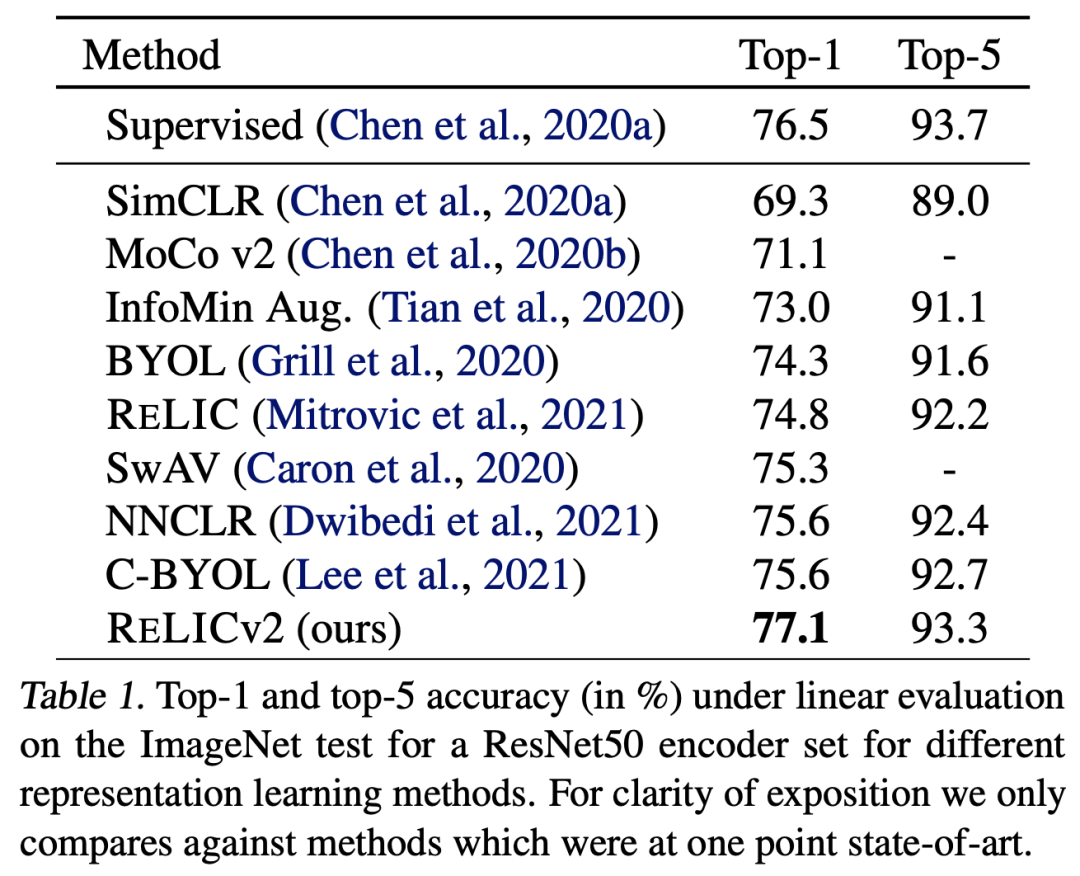
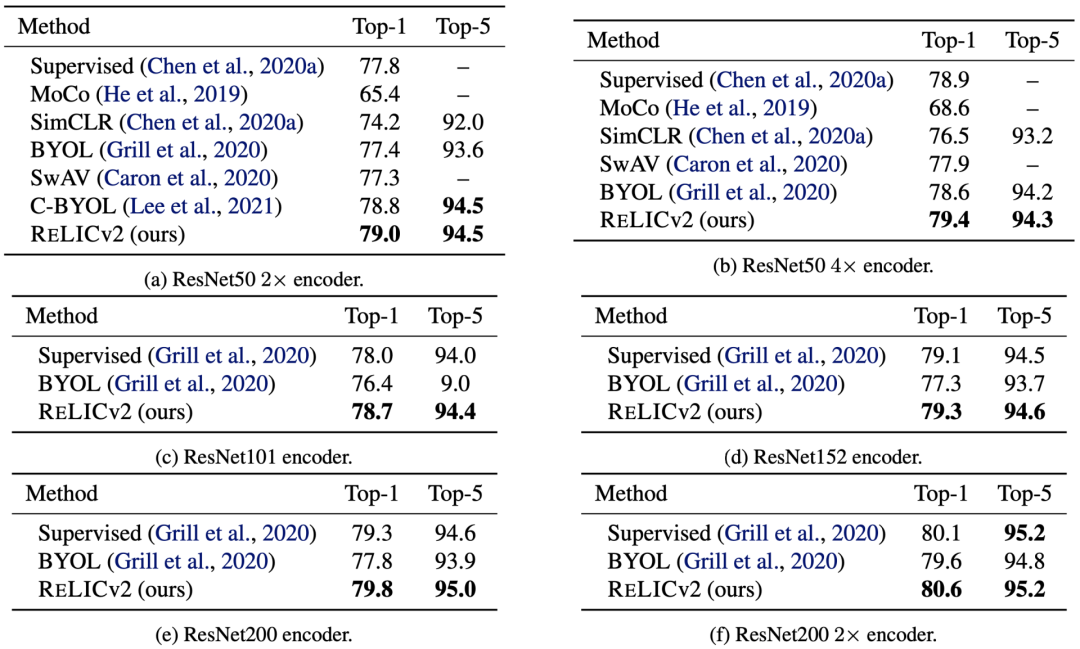
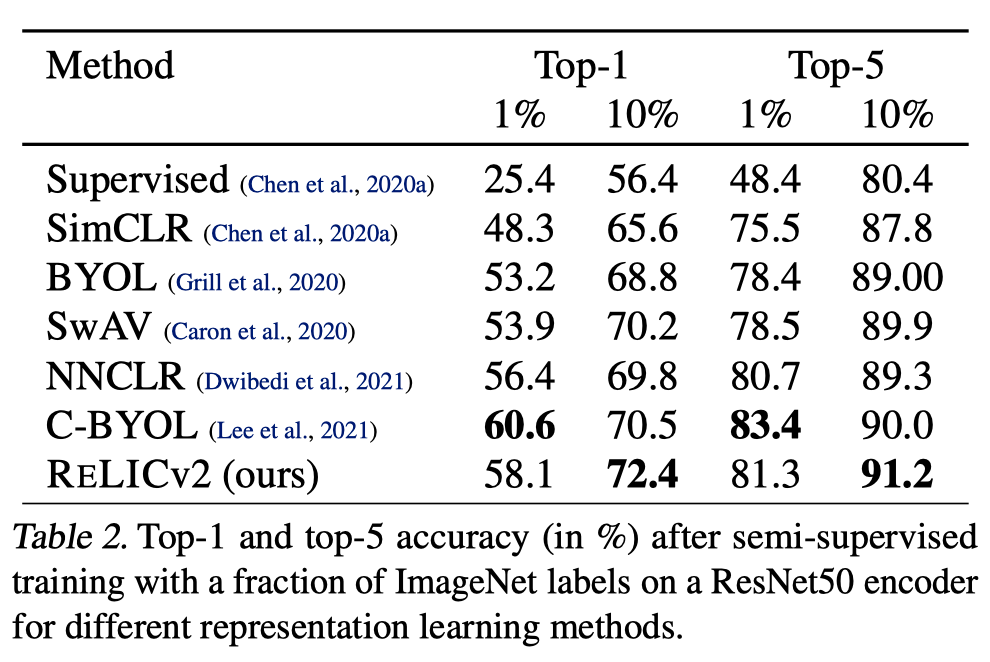
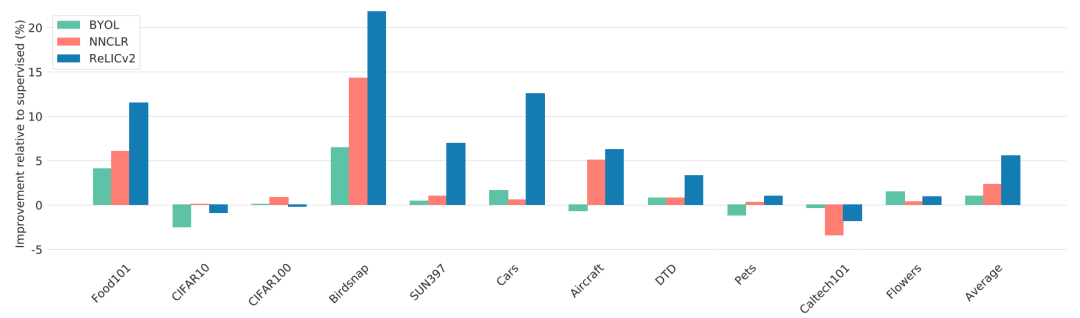
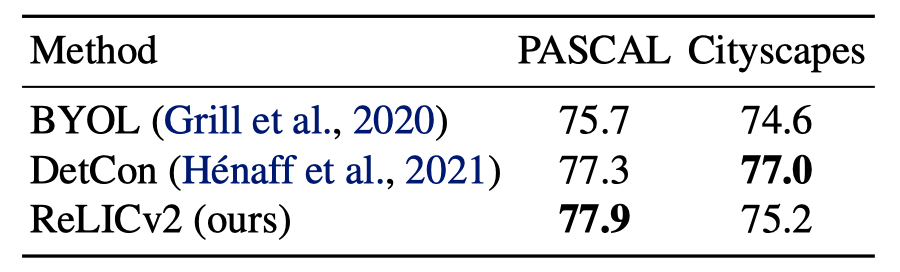
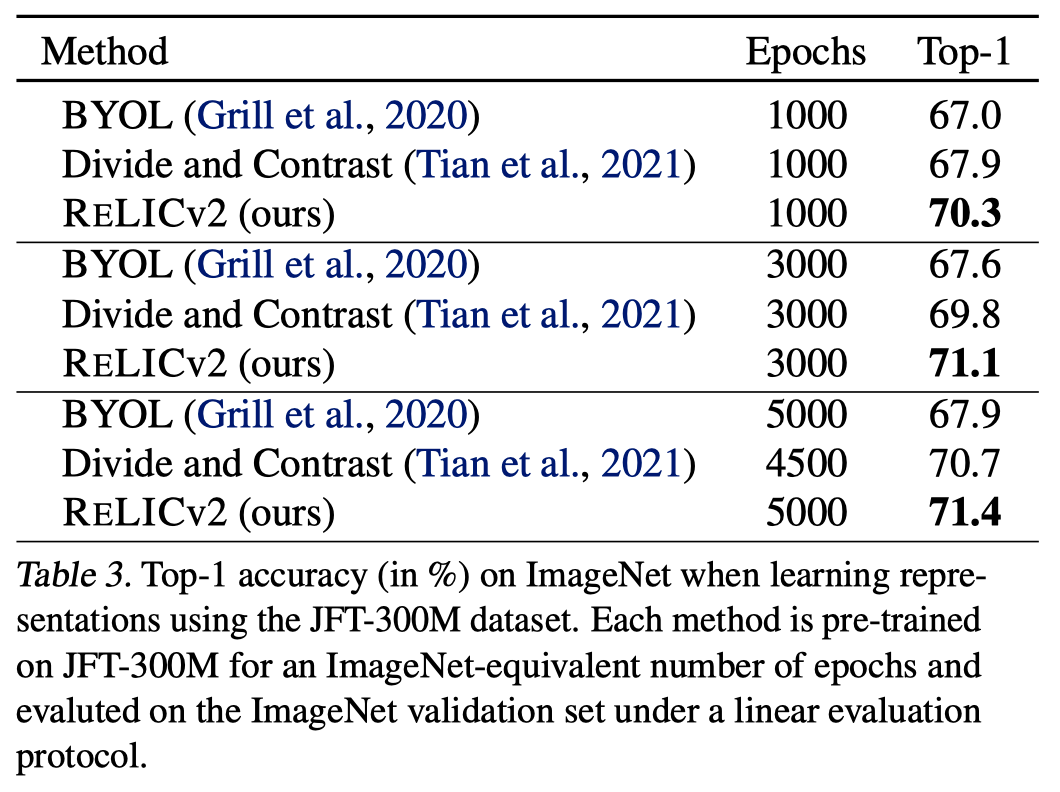
分析
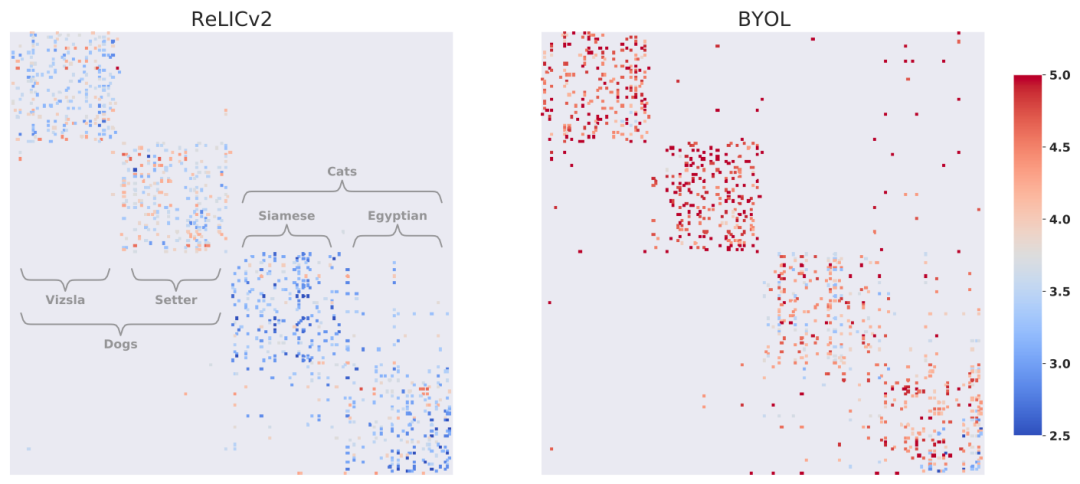
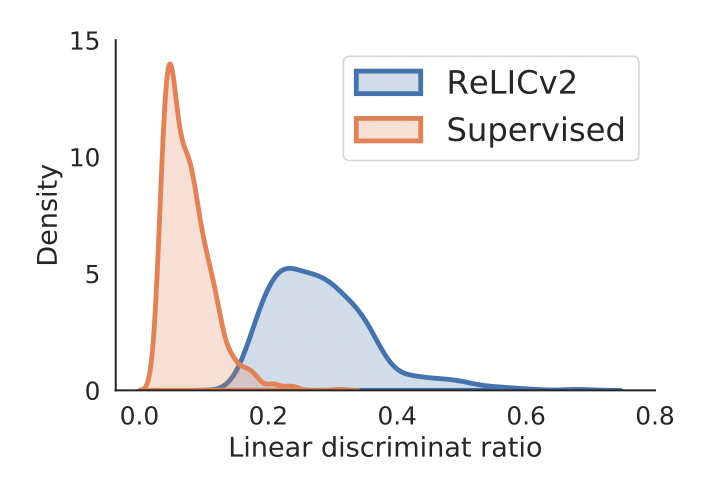
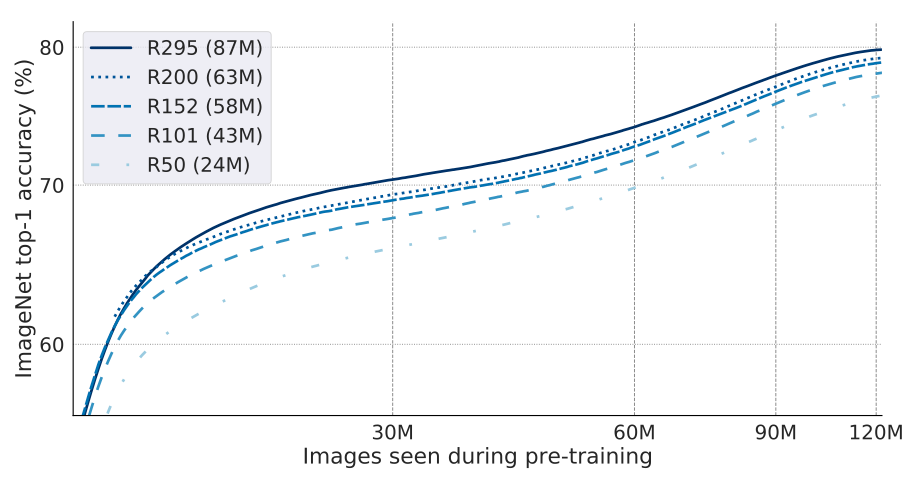
结论
参考资料:
https://arxiv.org/abs/2201.05119
评论