【NLP】Transformer温故知新
共 11968字,需浏览 24分钟
·
2020-12-01 20:44
这是之前学习paddle时候的笔记,对Transformer框架进行了拆解,附图解和代码,希望对大家有帮助 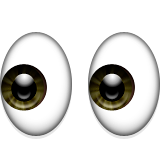
写在前面
最近在学习paddle相关内容,质量比较高的参考资料好像就paddle官方文档[1]。所以如果大家想学习一下的话,可以先简单过一遍文档,如果你之前有tensorflow或者torch的基础,看起来应该会比较快,都差不多的嘛。然后细节的部分就可以去实战看(写)代码了。下面是一个用paddle实现的目前NLP领域最火的Transformer模型,包括模型详细的拆解可视化以及对应每一步的代码实现,enjoy!
Encoder Part Residuals & Layer Norm Feed Forward Self-Attention 完整Encoder代码 Decoder Part Masked Multi-Head Attention Encoder-Decoder Attention 整体Decoder代码 Full Transformer
一、Encoder Part
下图是一个encoder block,可以看到主要由以下四部分组成:
Self-Attention Feed Forward Residual Connection Layer Norm
下面我们由简单至复杂来搭建每一部分并给出对应代码。
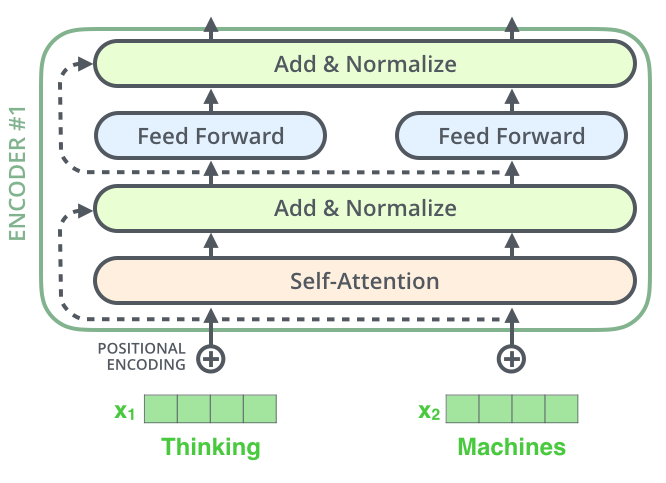
1、Residuals & Layer Norm
也就是上图中浅绿色框框中的内容。
「残差层」:大多数关于transformer的文章对于Residual的介绍都比较简单,但是实际上残差层在整个网络中的作用非常重要,它可以有效解决网络模型层数增大而引起的信息损失问题,来自kaiming大神的Deep residual learning for image recognition[2]; 「归一化」:神经网络中关于归一化的做法有很多,这里使用的是层归一化,更详细的资料推荐张俊林老师的深度学习中的Normalization模型[3]
def pre_post_process_layer(prev_out, out, process_cmd, dropout_rate=0.):
"""
根据process_cmd的值来设置不同的操作
"""
for cmd in process_cmd:
if cmd == "a": # 添加residual connection
out = out + prev_out if prev_out else out
elif cmd == "n": # 添加layer normalization
out = layers.layer_norm(out, begin_norm_axis=len(out.shape)-1,
param_attr=fluid.initializer.Constant(1.),
bias_attr=fluid.initializer.Constant(0.))
elif cmd == "d": # 添加dropout
if dropout_rate:
out = layers.dropout(out,dropout_prob=dropout_rate,
seed=dropout_seed,
is_test=False)
return out
2、Feed Forward
前馈层,上图中蓝色框框,包括两个线性变换,使用的激活函数为「relu」
注意,self-attention是对所有输入统一处理的,而前馈层是position-wise的。参数在同一层是共享的,但是在层与层之间是独立的。输入和输出的维度 ,内层的维度
def positionwise_feed_forward(x, d_inner_hid, d_hid, dropout_rate):
hidden = layers.fc(input=x,size=d_inner_hid,num_flatten_dims=2,act="relu")
if dropout_rate:
hidden = layers.dropout(hidden, dropout_prob=dropout_rate, seed=dropout_seed, is_test=False)
out = layers.fc(input=hidden, size=d_hid, num_flatten_dims=2)
return out
3、Self-Attention
Transformer中最重要的组成部分。在所有的attention中最关键就是要理解三个概念:、、。很多同学可能都对这三个词非常熟悉,但是对其具体对应的实体非常模糊,这里我们以检索为例介绍一下其原理,看看能不能理解。(已经了解的自行跳过haha)
比如你现在想要了解一下「自然语言处理」的相关信息,你就会去搜索框里输入
自然语言处理
,这时候你输入的就是 ;接着搜索库里会有很多文档,想要返回你的搜索结果,一个简单的思路就是看看你的搜索内容和文档title的相似度,这时候每个文档title就是 ,将 和每个 做点乘计算相似度,我们会得到一系列数值 ,然后用这一组值对文档,也就是我们的 进行加权召回,按得分降序返回。
所以本质上我们可以将Attention简单理解为「加权和」。更为具体的注意力介绍可以参考我之前的博客:理解Attention机制原理及模型[4]
3.1 Scaled Dot Product Attention
下面来看看具体在文本中是怎么运算的。
每一个输入经过Embedding层后转化成词向量 ; 所有token都会经过三个可学习矩阵分别映射为三个向量 、、, 对每一个单词,将其作为 ,所有单词作为 ,计算相似度得分; 将相似度得分进行规一化后与对应的 相乘,得出加权和,即为该单词的注意力得分。
公式为:
其可视化过程如下图,
转换成矩阵形式可以简化表示为,
def __compute_qkv(queries, keys, values, n_head, d_key, d_value):
"""
上述第二步,将输入通过三个可学习的矩阵映射为query、value和key
"""
q = layers.fc(input=queries,size=d_key*n_head,bias_attr=False,num_flatten_dims=2)
fc_layer = wrap_layer_with_block(
layers.fc, fluid.default_main_program().current_block()
.parent_idx) if cache is not None and static_kv else layers.fc
k = fc_layer(
input=keys,
size=d_key*n_head,
bias_attr=False,
num_flatten_dims=2)
v = fc_layer(
input=values,
size=d_value*n_head,
bias_attr=False,
num_flatten_dims=2)
return q, k, v
def scaled_dot_product_attention(q, k, v, attn_bias, d_key, dropout_rate):
"""
上述第三、四步,计算attention得分
"""
product = layers.matmul(x=q, y=k, transpose_y=True, alpha=d_key**-0.5)
if attn_bias:
product += attn_bias
weights = layers.softmax(product)
if dropout_rate:
weights = layers.dropout(
weights,
dropout_prob=dropout_rate,
seed=dropout_seed,
is_test=False)
out = layers.matmul(weights, v)
return out
注意scaled_dot_product_attention
函数中有一个attn_bias
的操作,作用是mask掉指定的位置。在整个transformer的结构中,使用的地方有三处:
Encoder的self-attention,作用是mask掉padding的位置; Decoder的encoder-self-attention,作用是mask掉padding的位置; Decoder的masked-self-attention,作用是解码过程mask掉当前词之后的词信息
3.2 Multi-Head Attention
multi-head的出发点是为了让模型在多个不同的子空间中学习到不同方面的信息,帮助模型捕获更丰富的特征。
操作也非常容易理解,
首先将输入映射为 、、, 接着拆分成 个注意力头,并行地运算上一节中的 Scaled Dot Product Attention
,最后将结果进行拼接。
整体计算公式为:
可视化如下图所示:
3.2.1 拆分
在输入张量的最后一个维度上进行reshape以拆分出多头,然后转置方便后续运算。具体而言,将输入形状为[bs,max_sequence_length,n_head * hidden_dim]
转换为[bs,n_head,max_sequence_length,hidden_dim]
def __split_heads_qkv(queries, keys, values, n_head, d_key, d_value):
# reshape:这里shape参数里的0表示从输入张量对应维数直接复制出来
# inplace=True,不进行数据的复制,运算更为高效
reshaped_q = layers.reshape(x=queries, shape=[0, 0, n_head, d_key], inplace=True)
# 转置:perm参数表示将第一个和第二个维度交换
q = layers.transpose(x=reshaped_q, perm=[0, 2, 1, 3])
reshape_layer = wrap_layer_with_block(layers.reshape,
fluid.default_main_program().current_block().parent_idx)
if cache is not None and static_kv else layers.reshape
transpose_layer = wrap_layer_with_block(layers.transpose,
fluid.default_main_program().current_block().parent_idx)
if cache is not None and static_kv else layers.transpose
reshaped_k = reshape_layer(x=keys, shape=[0, 0, n_head, d_key], inplace=True)
k = transpose_layer(x=reshaped_k, perm=[0, 2, 1, 3])
reshaped_v = reshape_layer(x=values, shape=[0, 0, n_head, d_value], inplace=True)
v = transpose_layer(x=reshaped_v, perm=[0, 2, 1, 3])
# 设计的优化,包括推断过程的缓存和处理流程
if cache is not None:
cache_, i = cache
if static_kv:
cache_k, cache_v = cache_["static_k"], cache_["static_v"]
static_cache_init = wrap_layer_with_block(
layers.assign,
fluid.default_main_program().current_block().parent_idx)
static_cache_init(k,fluid.default_main_program().global_block().var("static_k_%d" % i))
static_cache_init(v,fluid.default_main_program().global_block().var("static_v_%d" % i))
k, v = cache_k, cache_v
else:
cache_k, cache_v = cache_["k"], cache_["v"]
k = layers.concat([cache_k, k], axis=2)
v = layers.concat([cache_v, v], axis=2)
cache_["k"], cache_["v"] = (k, v)
return q, k, v
3.2.2 合并
可以认为是上一节拆分
的逆操作,先是transpose
,再是reshape
。具体而言,将输入形状为[bs,n_head,max_sequence_length,hidden_dim]
转换为[bs,max_sequence_length,n_head * hidden_dim]
def __combine_heads(x):
# 首先验证输入形状
if len(x.shape) != 4:
raise ValueError("Input(x) should be a 4-D Tensor.")
trans_x = layers.transpose(x, perm=[0, 2, 1, 3])
return layers.reshape(x=trans_x,shape=[0, 0, trans_x.shape[2] * trans_x.shape[3]],inplace=True)
3.2.3 整体
有了前面几节的函数操作之后,就可以构建整体multi-head attention了
def multi_head_attention(queries,keys,values,attn_bias,d_key,d_value,d_model, n_head=1,dropout_rate=0.,cache=None,static_kv=False):
keys = queries if keys is None else keys
values = keys if values is None else values
## 检查输入形状
if not (len(queries.shape) == len(keys.shape) == len(values.shape) == 3):
raise ValueError(
"Inputs: quries, keys and values should all be 3-D tensors.")
q, k, v = __compute_qkv(queries, keys, values, n_head, d_key, d_value)
q, k, v = __split_heads_qkv(q, k, v, n_head, d_key, d_value)
ctx_multiheads = scaled_dot_product_attention(q, k, v, attn_bias, d_model,dropout_rate)
out = __combine_heads(ctx_multiheads)
proj_out = layers.fc(input=out,size=d_model,bias_attr=False,num_flatten_dims=2)
return proj_out
4、完整Encoder代码
有了上面的铺垫之后,我们就可以写出encoder的代码框架了。具体代码篇幅原因就不再粘贴,可以根据开篇所述方式获取。
二、Decoder Part
ok,我们先停下来回顾一下前面都解决了哪些内容:
Encoder包含的几个部分:self-attention、feed-forward、add&norm 详细介绍了Scaled Dot Product Attention原理及代码实现 详细介绍了Multi-Head Attention原理及代码实现
接下去来看看transformer的右半部分:Decoder。如下图所示,是一个decoder block,主要由五部分组成:
Encoder-Decoder Attention (Masked)Self-Attention Feed Forward Residual Connection Layer Norm
1、Masked Multi-Head Attention
关于这个,我们在前面3.1节其实有过说明,当解码第 个特征向量时,我们只能看到其之前的解码结果,这样做的目的也很直观,防止信息泄露,因为我们总不能偷看答案吧哈哈。
那么具体怎么做呢?其实也不难:构造一个mask矩阵,上三角全为0,表示无法attend未来的信息,如下,
2、Encoder-Decoder Attention
其实Deocer的五个组件我们在Encoder Part里面已经完成了四个部分,只剩下一个Encoder-Decoder Attention
是没有涉及的。其实这个跟encoder_layer
的差不多,只不过是它的 和 来自encoder的输出,而 则来自decoder的上一个输出。
可视化动图就更清楚了,
3、整体Decoder代码
不啰嗦了,跟encoder很类似,看代码也很直观。
三、Full Transformer
终于快写完了....
最后我们就像搭积木一样,把前面的部分组建成一个完整的transformer网络,如下图
def transformer(model_input,src_vocab_size,trg_vocab_size,max_length,n_layer,n_head,d_key,d_value,d_model,d_inner_hid,prepostprocess_dropout,attention_dropout,
relu_dropout,preprocess_cmd,postprocess_cmd,weight_sharing,label_smooth_eps,bos_idx=0,is_test=False):
if weight_sharing:
assert src_vocab_size == trg_vocab_size, (
"Vocabularies in source and target should be same for weight sharing."
)
enc_inputs = (model_input.src_word, model_input.src_pos,model_input.src_slf_attn_bias)
dec_inputs = (model_input.trg_word, model_input.trg_pos,model_input.trg_slf_attn_bias, model_input.trg_src_attn_bias)
label = model_input.lbl_word
weights = model_input.lbl_weight
enc_output = wrap_encoder(enc_inputs,src_vocab_size,max_length,n_layer,n_head,d_key,d_value,d_model,d_inner_hid,prepostprocess_dropout,
attention_dropout,relu_dropout,preprocess_cmd,postprocess_cmd,weight_sharing,bos_idx=bos_idx)
predict = wrap_decoder(dec_inputs,trg_vocab_size,max_length,n_layer,n_head,d_key,d_value,d_model,d_inner_hid,prepostprocess_dropout,
attention_dropout,relu_dropout,preprocess_cmd,postprocess_cmd,weight_sharing,enc_output=enc_output)
# Padding index do not contribute to the total loss. The weights is used to
# cancel padding index in calculating the loss.
if label_smooth_eps:
# TODO: use fluid.input.one_hot after softmax_with_cross_entropy removing
# the enforcement that the last dimension of label must be 1.
label = layers.label_smooth(
label=layers.one_hot(
input=label, depth=trg_vocab_size),
epsilon=label_smooth_eps)
cost = layers.softmax_with_cross_entropy(logits=predict,label=label,soft_label=True if label_smooth_eps else False)
weighted_cost = layers.elementwise_mul(x=cost, y=weights, axis=0)
sum_cost = layers.reduce_sum(weighted_cost)
token_num = layers.reduce_sum(weights)
token_num.stop_gradient = True
avg_cost = sum_cost / token_num
return sum_cost, avg_cost, predict, token_num
积木搭好了,我们怎么调用呢?下面就可以写一个create_net
函数,接受输入为is_training
(是否训练阶段),model_input
(模型输入),args
(一些词表、维度、长度等模型参数)
def create_net(is_training, model_input, args):
if is_training:
sum_cost, avg_cost, _, token_num = transformer(
model_input, args.src_vocab_size, args.trg_vocab_size,
args.max_length + 1, args.n_layer, args.n_head, args.d_key,
args.d_value, args.d_model, args.d_inner_hid,
args.prepostprocess_dropout, args.attention_dropout,
args.relu_dropout, args.preprocess_cmd, args.postprocess_cmd,
args.weight_sharing, args.label_smooth_eps, args.bos_idx)
return sum_cost, avg_cost, token_num
else:
out_ids, out_scores = fast_decode(
model_input, args.src_vocab_size, args.trg_vocab_size,
args.max_length + 1, args.n_layer, args.n_head, args.d_key,
args.d_value, args.d_model, args.d_inner_hid,
args.prepostprocess_dropout, args.attention_dropout,
args.relu_dropout, args.preprocess_cmd, args.postprocess_cmd,
args.weight_sharing, args.beam_size, args.max_out_len, args.bos_idx,
args.eos_idx)
return out_ids, out_scores
本文参考资料
paddle官方文档: https://www.paddlepaddle.org.cn/
[2]Deep residual learning for image recognition: https://arxiv.org/abs/1512.03385
[3]深度学习中的Normalization模型: https://zhuanlan.zhihu.com/p/43200897
[4]理解Attention机制原理及模型: https://blog.csdn.net/Kaiyuan_sjtu/article/details/81806123
- END -
往期精彩回顾
获取本站知识星球优惠券,复制链接直接打开:
https://t.zsxq.com/qFiUFMV
本站qq群704220115。
加入微信群请扫码: