大模型LLM-微调经验分享&总结
作者:刘聪NLP
链接:https://zhuanlan.zhihu.com/p/620885226
写在前面
ChatGLM-6B模型微调
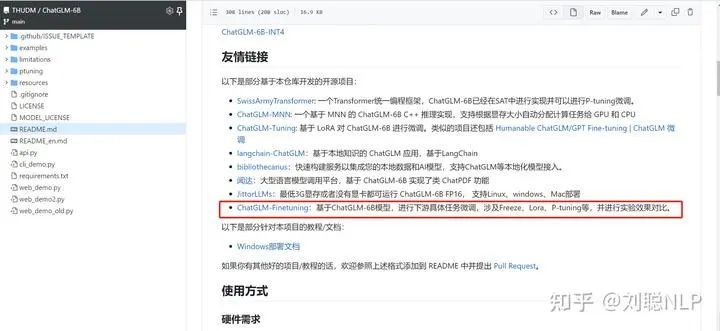
Freeze方法
for name, param in model.named_parameters():
if not any(nd in name for nd in ["layers.27", "layers.26", "layers.25", "layers.24", "layers.23"]):
param.requires_grad = False
CUDA_VISIBLE_DEVICES=0 deepspeed finetuning_freeze.py --num_train_epochs 5 --train_batch_size 2
PT方法
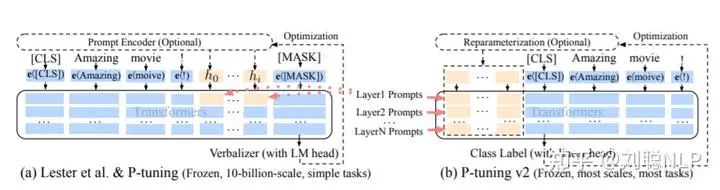
-
P-Tuning(https://arxiv.org/abs/2103.10385),仅对大模型的Embedding加入新的参数。 -
P-Tuning-V2(https://arxiv.org/abs/2110.07602),将大模型的Embedding和每一层前都加上新的参数。
config = ChatGLMConfig.from_pretrained(args.model_dir)
config.pre_seq_len = args.pre_seq_len
config.prefix_projection = args.prefix_projection
model = ChatGLMForConditionalGeneration.from_pretrained(args.model_dir, config=config)
for name, param in model.named_parameters():
if not any(nd in name for nd in ["prefix_encoder"]):
param.requires_grad = False
CUDA_VISIBLE_DEVICES=0 deepspeed finetuning_pt.py --num_train_epochs 5 --train_batch_size 2 --pre_seq_len 16
Lora方法
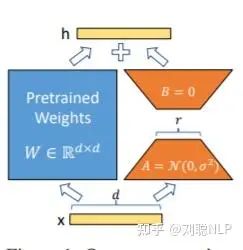
-
Lora论文:https://arxiv.org/abs/2106.09685 -
官方代码:https://github.com/microsoft/LoRA -
HuggingFace封装的peft库:https://github.com/huggingface/peft
model = ChatGLMForConditionalGeneration.from_pretrained(args.model_dir)
config = LoraConfig(r=args.lora_r,
lora_alpha=32,
target_modules=["query_key_value"],
lora_dropout=0.1,
bias="none",
task_type="CAUSAL_LM",
inference_mode=False,
)
model = get_peft_model(model, config)
CUDA_VISIBLE_DEVICES=0 deepspeed finetuning_lora.py --num_train_epochs 5 --train_batch_size 2 --lora_r 8
三元组抽取实验结果
-
模型训练时,最大长度为768,Batch大小为2,训练轮数为5,fp16训练,采用DeepSpeed的Zero-1训练; -
PT为官方的P-Tuning V2训练方法,PT-Only-Embedding表示仅对Embedding进行soft-prompt,Freeze仅训练模型后五层参数,Lora采用低秩矩阵方法训练,秩为8; -
由于之前训练PT在48G-A40显卡上会出现OOM,因此之前进行PT实验时对模型开启了gradient_checkpointing_enable,使得模型显存占用变小,但训练时长增加。 -
训练示例:
prompt_text:你现在是一个信息抽取模型,请你帮我抽取出关系内容为\"性能故障\", \"部件故障\", \"组成\"和 \"检测工具\"的相关三元组,三元组内部用\"_\"连接,三元组之间用\\n分割。文本:
输入:故障现象:发动机水温高,风扇始终是低速转动,高速档不工作,开空调尤其如此。
输出:发动机_部件故障_水温高\n风扇_部件故障_低速转动
|
|
|
|
|
|
|
|
|
|
|
|
|
|
|
|
|
|
|
|
|
|
|
|
|
-
效果为PT>Freeze>Lora>PT-Only-Embedding; -
速度为PT-Only-Embedding>Lora>Freeze>PT; -
PT-Only-Embedding效果很不理想,发现在训练时,最后的loss仅能收敛到2.几,而其他机制可以收敛到0.几。分析原因为,输出内容形式与原有语言模型任务相差很大,仅增加额外Embedding参数,不足以改变复杂的下游任务; -
PT方法占用显存更大,因为也增加了很多而外参数; -
测试耗时,采用float16进行模型推理,由于其他方法均增加了额外参数,因此其他方法的推理耗时会比Freeze方法要高。当然由于是生成模型,所以生成的长度也会影响耗时; -
模型在指定任务上微调之后,并没有丧失原有能力,例如生成“帮我写个快排算法”,依然可以生成-快排代码; -
由于大模型微调都采用大量instruction进行模型训练,仅采用单一的指令进行微调时,对原来其他的指令影响不大,因此并没导致原来模型的能力丧失; -
上面测试仅代表个人测试结果。
-
翻译任务

-
代码任务
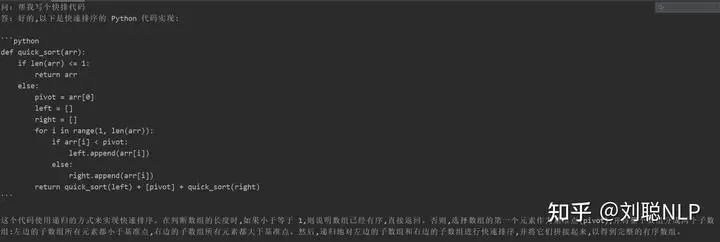
-
问答任务

中文开源大模型&项目
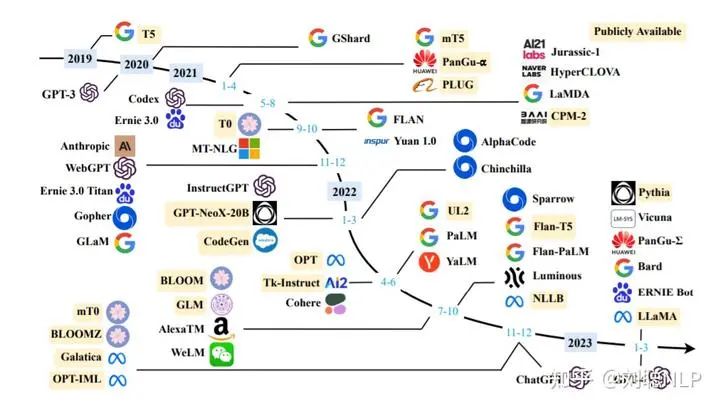
中文开源大模型
-
ChatGLM-6B:https://huggingface.co/THUDM/chatglm-6b -
ChatYuan-large-v2:https://huggingface.co/ClueAI/ChatYuan-large-v2
-
BloomZ:https://huggingface.co/bigscience/bloomz -
LLama:https://github.com/facebookresearch/llama -
Flan-T5:https://huggingface.co/google/flan-t5-xxl -
OPT:https://huggingface.co/facebook/opt-66b
中文开源指令数据
-
[1]:https://github.com/LC1332/Chinese-alpaca-lora -
[2]:https://github.com/hikariming/alpaca_chinese_dataset -
[3]:https://github.com/carbonz0/alpaca-chinese-dataset -
[4]:https://github.com/Instruction-Tuning-with-GPT-4/GPT-4-LLM -
[5]:https://github.com/LianjiaTech/BELLE -
[6]:https://huggingface.co/datasets/JosephusCheung/GuanacoDataset
开源项目
-
BELLE:https://github.com/LianjiaTech/BELLE -
ChatGLM:https://github.com/THUDM/ChatGLM-6B -
Luotuo-Chinese-LLM:https://github.com/LC1332/Luotuo-Chinese-LLM -
stanford_alpaca:https://github.com/tatsu-lab/stanford_alpaca
总结
END
评论