详细记录YOLACT实例分割ncnn实现
点击上方“小白学视觉”,选择加"星标"或“置顶”
重磅干货,第一时间送达
端到端一阶段完成实例分割 速度快,550x550图片在TitanXP上号称达到33FPS 开源代码,pytorch大法好!
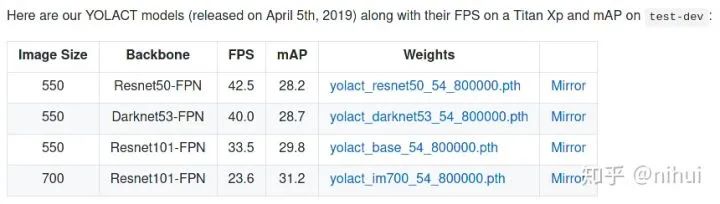
$ python eval.py --trained_model=weights/yolact_resnet50_54_800000.pth --score_threshold=0.15 --top_k=15 --image=test.jpg
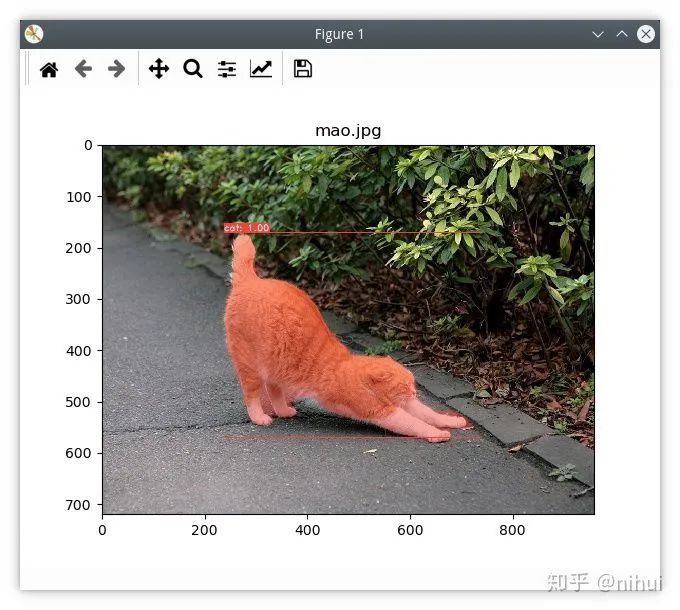
0x3 去掉后处理导出onnx
def evalimage(net:Yolact, path:str, save_path:str=None):
frame = torch.from_numpy(cv2.imread(path)).cuda().float()
batch = FastBaseTransform()(frame.unsqueeze(0))
preds = net(batch)
torch.onnx._export(net, batch, "yolact.onnx", export_params=True, keep_initializers_as_inputs=True, opset_version=11)
# As of March 10, 2019, Pytorch DataParallel still doesn't support JIT Script Modules
use_jit = False
后处理部分没有标准化,每个项目作者的实现细节也各不相同,比如各种nms和bbox计算方式,ncnn很难用统一的op实现(caffe-ssd因为只有一种版本,所以有实现) 后处理在onnx中会转换成一大坨胶水op,非常琐碎,在框架中实现效率低下 onnx的大部分胶水op,ncnn不支持或有兼容问题,比如Gather等,无法直接使用
# return self.detect(pred_outs, self)
return pred_outs;
$ python eval.py --trained_model=weights/yolact_resnet50_54_800000.pth --score_threshold=0.15 --top_k=15 --image=test.jpg
$ pip install -U onnx --user
$ pip install -U onnxruntime --user
$ pip install -U onnx-simplifier --user
$ python -m onnxsim yolact.onnx yolact-sim.onnx
Graph must be in single static assignment (SSA) form, however '523' has been used as output names multiple times
python -m onnxsim --skip-fuse-bn yolact.onnx yolact-sim.onnx
0x5 ncnn模型转换和优化
./onnx2ncnn yolact-sim.onnx yolact.param yolact.bin
./ncnnoptimize yolact.param yolact.bin yolact-opt.param yolact-opt.bin 0
0x6 手工微调模型
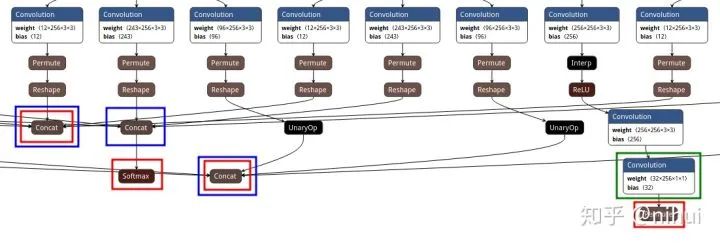
Convolution Conv_263 1 1 617 619 0=32 1=1 5=1 6=8192 9=1
Permute Transpose_265 1 1 619 620 0=3
UnaryOp Tanh_400 1 1 814 815 0=16
Concat Concat_401 5 1 634 673 712 751 790 816 0=-3
Concat Concat_402 5 1 646 685 724 763 802 817 0=-3
Concat Concat_403 5 1 659 698 737 776 815 818 0=-3
Softmax Softmax_405 1 1 817 820 0=1 1=1
ncnn::Extractor ex = yolact.create_extractor();
ncnn::Mat in(550, 550, 3);
ex.input("input.1", in);
ncnn::Mat b620;
ncnn::Mat b816;
ncnn::Mat b818;
ncnn::Mat b820;
ex.extract("620", b620);// 32 x 138x138
ex.extract("816", b816);// 4 x 19248
ex.extract("818", b818);// 32 x 19248
ex.extract("820", b820);// 81 x 19248
Concat Concat_401 5 1 634 673 712 751 790 816 0=0
Concat Concat_402 5 1 646 685 724 763 802 817 0=0
Concat Concat_403 5 1 659 698 737 776 815 818 0=0
ncnn::Extractor ex = yolact.create_extractor();
ncnn::Mat in(550, 550, 3);
ex.input("input.1", in);
ncnn::Mat maskmaps;
ncnn::Mat location;
ncnn::Mat mask;
ncnn::Mat confidence;
ex.extract("619", maskmaps);// 138x138 x 32
ex.extract("816", location);// 4 x 19248
ex.extract("818", mask);// maskdim 32 x 19248
ex.extract("820", confidence);// 81 x 19248
0x7 生成prior
const int conv_ws[5] = {69, 35, 18, 9, 5};
const int conv_hs[5] = {69, 35, 18, 9, 5};
const float aspect_ratios[3] = {1.f, 0.5f, 2.f};
const float scales[5] = {24.f, 48.f, 96.f, 192.f, 384.f};
// make priorbox
ncnn::Mat priorbox(4, 19248);
{
float* pb = priorbox;
for (int p = 0; p < 5; p++)
{
int conv_w = conv_ws[p];
int conv_h = conv_hs[p];
float scale = scales[p];
for (int i = 0; i < conv_h; i++)
{
for (int j = 0; j < conv_w; j++)
{
// +0.5 because priors are in center-size notation
float cx = (j + 0.5f) / conv_w;
float cy = (i + 0.5f) / conv_h;
for (int k = 0; k < 3; k++)
{
float ar = aspect_ratios[k];
ar = sqrt(ar);
float w = scale * ar / 550;
float h = scale / ar / 550;
// This is for backward compatability with a bug where I made everything square by accident
// cfg.backbone.use_square_anchors:
h = w;
pb[0] = cx;
pb[1] = cy;
pb[2] = w;
pb[3] = h;
pb += 4;
}
}
}
}
}
0x8 YOLACT全流程实现
预处理部分
# These are in BGR and are for ImageNet
MEANS = (103.94, 116.78, 123.68)
STD = (57.38, 57.12, 58.40)
const int target_size = 550;
int img_w = bgr.cols;
int img_h = bgr.rows;
ncnn::Mat in = ncnn::Mat::from_pixels_resize(bgr.data, ncnn::Mat::PIXEL_BGR2RGB, img_w, img_h, target_size, target_size);
const float mean_vals[3] = {123.68f, 116.78f, 103.94f};
const float norm_vals[3] = {1.0/58.40f, 1.0/57.12f, 1.0/57.38f};
in.substract_mean_normalize(mean_vals, norm_vals);
后处理部分
// generate all candidates for each class
for (int i=0; i<num_priors; i++)
{
// find class id with highest score
// start from 1 to skip background
// ignore background or low score
if (label == 0 || score <= confidence_thresh)
continue;
// apply center_size to priorbox with loc
float var[4] = {0.1f, 0.1f, 0.2f, 0.2f};
float pb_cx = pb[0];
float pb_cy = pb[1];
float pb_w = pb[2];
float pb_h = pb[3];
float bbox_cx = var[0] * loc[0] * pb_w + pb_cx;
float bbox_cy = var[1] * loc[1] * pb_h + pb_cy;
float bbox_w = (float)(exp(var[2] * loc[2]) * pb_w);
float bbox_h = (float)(exp(var[3] * loc[3]) * pb_h);
float obj_x1 = bbox_cx - bbox_w * 0.5f;
float obj_y1 = bbox_cy - bbox_h * 0.5f;
float obj_x2 = bbox_cx + bbox_w * 0.5f;
float obj_y2 = bbox_cy + bbox_h * 0.5f;
// clip inside image
// append object candidate
}
// merge candidate box for each class
for (int i=0; i<(int)class_candidates.size(); i++)
{
// sort + nms
}
// sort all result by score
// keep_top_k
分割图生成
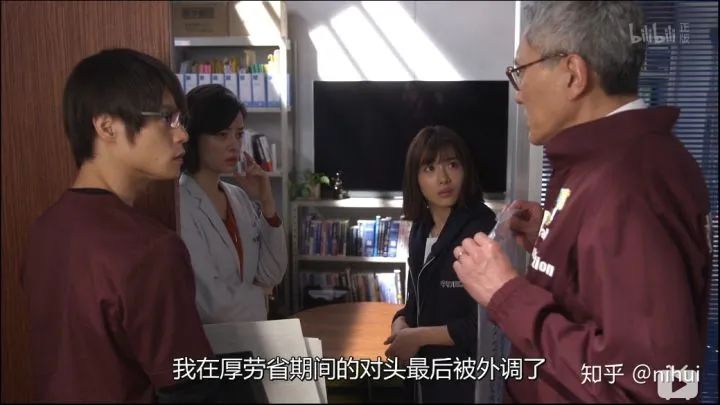
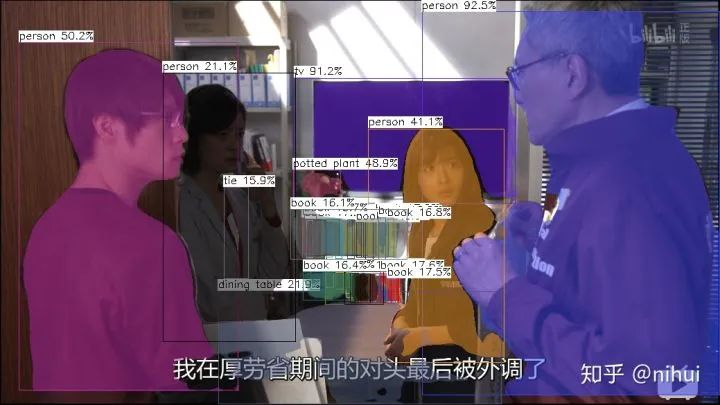
0x9 补充学习资料
https://link.zhihu.com/?target=https%3A//github.com/Tencent/ncnn
好消息!
小白学视觉知识星球
开始面向外开放啦👇👇👇
下载1:OpenCV-Contrib扩展模块中文版教程 在「小白学视觉」公众号后台回复:扩展模块中文教程,即可下载全网第一份OpenCV扩展模块教程中文版,涵盖扩展模块安装、SFM算法、立体视觉、目标跟踪、生物视觉、超分辨率处理等二十多章内容。 下载2:Python视觉实战项目52讲 在「小白学视觉」公众号后台回复:Python视觉实战项目,即可下载包括图像分割、口罩检测、车道线检测、车辆计数、添加眼线、车牌识别、字符识别、情绪检测、文本内容提取、面部识别等31个视觉实战项目,助力快速学校计算机视觉。 下载3:OpenCV实战项目20讲 在「小白学视觉」公众号后台回复:OpenCV实战项目20讲,即可下载含有20个基于OpenCV实现20个实战项目,实现OpenCV学习进阶。 交流群
欢迎加入公众号读者群一起和同行交流,目前有SLAM、三维视觉、传感器、自动驾驶、计算摄影、检测、分割、识别、医学影像、GAN、算法竞赛等微信群(以后会逐渐细分),请扫描下面微信号加群,备注:”昵称+学校/公司+研究方向“,例如:”张三 + 上海交大 + 视觉SLAM“。请按照格式备注,否则不予通过。添加成功后会根据研究方向邀请进入相关微信群。请勿在群内发送广告,否则会请出群,谢谢理解~
评论